Geometrical positioning surveying-based features for BEOL line-end-pull-back modeling using regression-based machine-learning
JOURNAL OF MICRO-NANOPATTERNING MATERIALS AND METROLOGY-JM3(2023)
摘要
Background:Line-end-pull-back (LEPB) is a well-known systematic defect in BEOL metal layers, where a line-end (LE) tip is pulled back from its desired location due to lithography (litho) process effects. Severe LEPB directly affects BEOL connectivity and may lead to partial or total metal-via disconnection.Aim:LEPB can be characterized through model-based litho simulations but at the cost of high computational resource consumption. This study aims to provide a fast and accurate approximation of computationally expensive litho simulations through regression-based machine learning (ML) modeling.Approach: LEPB modeling is approached through the LightGBM model. Input features were approached using density pixels, density concentric circle area sampling (CCAS), and geometrical positioning surveying (GPS), which is an edge-based engine that provides a direct one-to-one mapping between model features and geometrical measurements between the LE as a point-of-interest and its surrounding contextual patterns. The importance of LightGBM features by splits was employed to reduce features across the used approaches.Results: The reduced features of GPS produced almost the same modeling quality (training: RMS = 0.571 nm, d(EWD) = 0.297 nm, and R-2 % = 98.74 % , and testing: RMS = 0.643 nm, d(EWD) = 0.344 nm, and R-2 % = 98.40 % ) with -22.22 % fewer number of features and less feature extraction runtime compared to the full features set of density pixels and density CCAS approaches.Conclusions: Compared to model-based litho simulations, the obtained calibrated ML models can be used to provide fast, yet accurate predictions of the amounts of pull-back or extensions introduced at LEs near vias, eliminating a major contributor to systematic IC yield loss.
更多查看译文
关键词
modeling,features,surveying-based,line-end-pull-back,regression-based,machine-learning
AI 理解论文
溯源树
样例
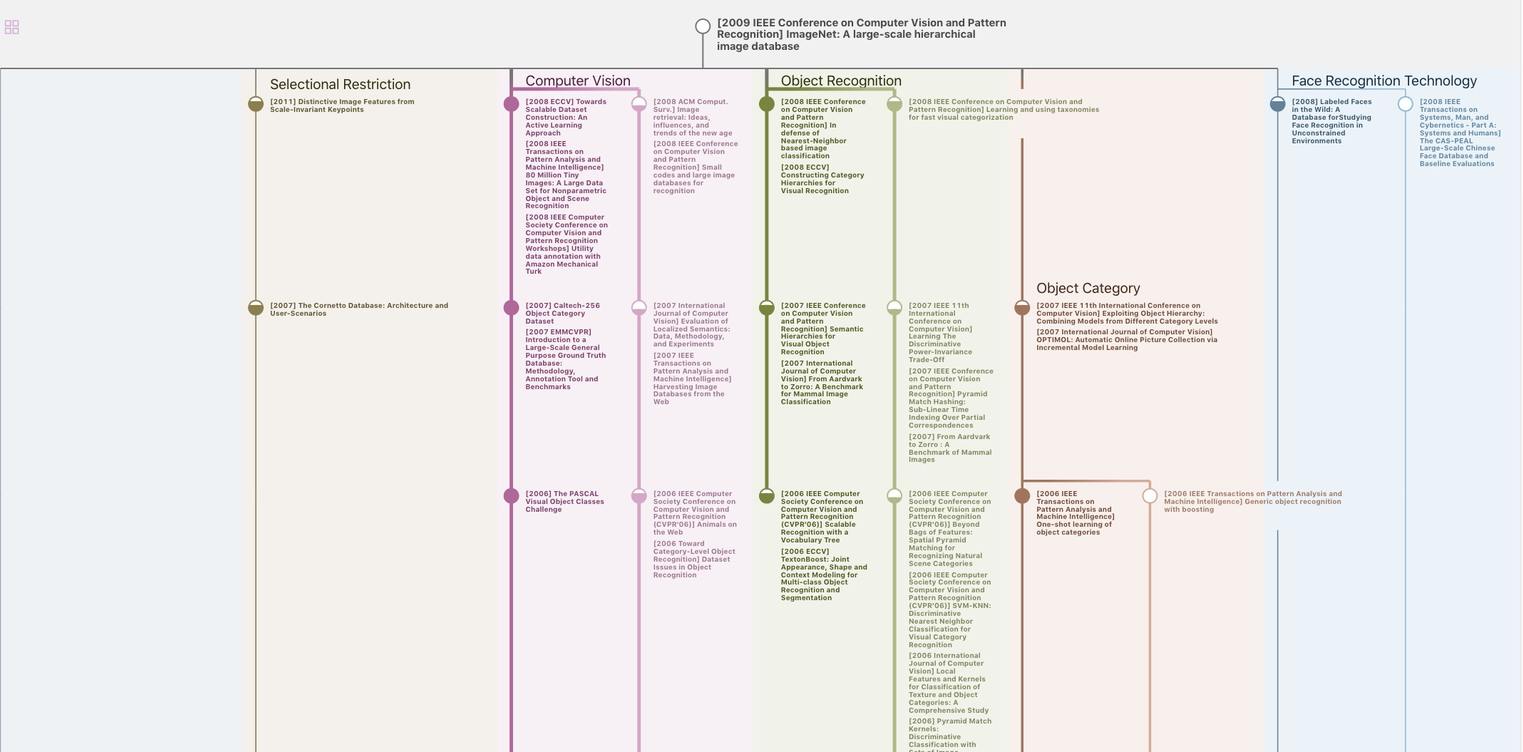
生成溯源树,研究论文发展脉络
Chat Paper
正在生成论文摘要