Boosting separated softmax with discrimination for class incremental learning
JOURNAL OF VISUAL COMMUNICATION AND IMAGE REPRESENTATION(2023)
摘要
Deep neural networks (DNNs) suffer from catastrophic forgetting when learning new classes continually and tend to classify old samples to new classes. Existing methods like exemplar-memory and knowledge distillation alleviate forgetting, but face prediction bias due to data imbalance. Separated softmax (Ahn et al., 2021) was proposed to solve this problem. However, they ignore the discrimination between old classes and new classes, which greatly limits the performance of DNNs. To enhance model’s discrimination, we propose discriminative separated softmax. We divide new samples into two parts: one combined with old samples to build a balanced dataset of all seen classes, and the other combined with old samples as an imbalanced dataset. Furthermore, we apply mixup for these two datasets respectively to enhance discrimination. We evaluate our method on CIFAR100 and ImageNet100. Experimental results show that our method can effectively improve the discrimination and achieves superior performance compared with separated softmax, while outperforming state-of-the-art methods.
更多查看译文
关键词
Incremental learning,Discrimination enhancement,Discriminative separated softmax
AI 理解论文
溯源树
样例
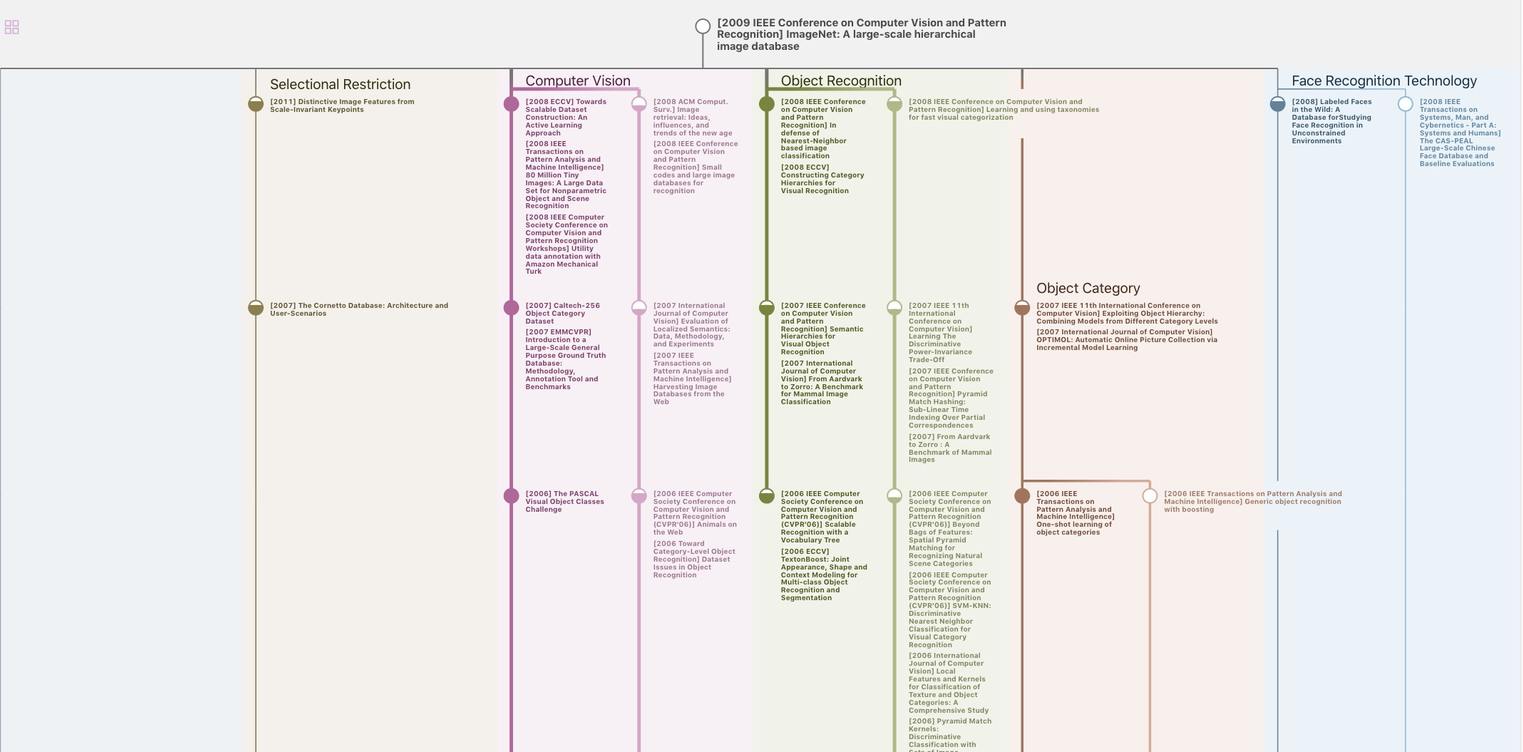
生成溯源树,研究论文发展脉络
Chat Paper
正在生成论文摘要