Visual privacy behaviour recognition for social robots based on an improved generative adversarial network
IET COMPUTER VISION(2024)
摘要
Although social robots equipped with visual devices may leak user information, countermeasures for ensuring privacy are not readily available, making visual privacy protection problematic. In this article, a semi-supervised learning algorithm is proposed for visual privacy behaviour recognition based on an improved generative adversarial network for social robots; it is called PBR-GAN. A 9-layer residual generator network enhances the data quality, and a 10-layer discriminator network strengthens the feature extraction. A tailored objective function, loss function, and strategy are proposed to dynamically adjust the learning rate to guarantee high performance. A social robot platform and architecture for visual privacy recognition and protection are implemented. The recognition accuracy of the proposed PBR-GAN is compared with Inception_v3, SS-GAN, and SF-GAN. The average recognition accuracy of the proposed PBR-GAN is 85.91%, which is improved by 3.93%, 9.91%, and 1.73% compared with the performance of Inception_v3, SS-GAN, and SF-GAN respectively. Through a case study, seven situations are considered related to privacy at home, and develop training and test datasets with 8,720 and 1,280 images, respectively, are developed. The proposed PBR-GAN recognises the designed visual privacy information with an average accuracy of 89.91%.
更多查看译文
关键词
computer vision,convolutional neural nets,feature extraction,human-robot interaction,image recognition
AI 理解论文
溯源树
样例
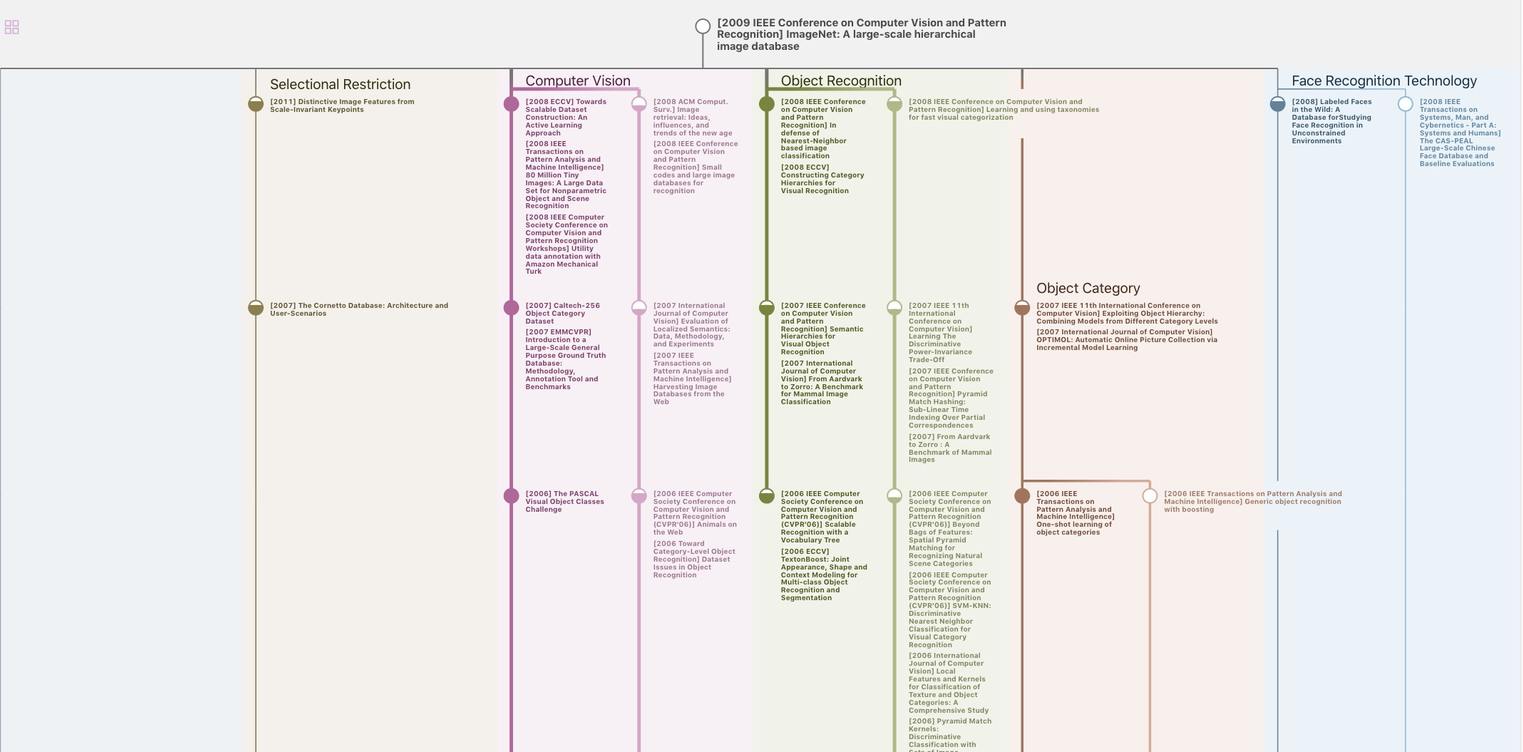
生成溯源树,研究论文发展脉络
Chat Paper
正在生成论文摘要