A novel two-stage bio-inspired method using red deer algorithm for data clustering
EVOLUTIONARY INTELLIGENCE(2023)
摘要
Data clustering is a method of dividing data points into similar groups. In the last few decades, there have been numerous advancements in algorithms and methodologies for the analysis of clustered data. Although some algorithms like K -means, due to their easy implementation and interpretability, are widely used for clustering, their capability of providing good solutions might be sensitive to the number of dimensions and clusters. Due to the advantages of metaheuristic-based clustering approaches like strong searchability, they have been widely used to tackle the clustering problem. Based on this premise, we propose a new bio-inspired red deer clustering algorithm (RDCA). Red deer algorithm (RDA) is a new metaheuristic inspired by the behavior of the Scottish red deer during a breeding season. First, the data space is partitioned into several divisions, each of which is represented by a disjunctive normal form expression using CLIQUE algorithm. Then a search strategy employing a modified version of RDA is pursued. Experiments are carried out to assess the performance of RDCA in comparison to eight traditional methods using six real-world and three generated datasets in terms of cluster validity measures, namely compactness, separation, combined, Turi, Davies Bouldin, and normalized mutual information score. The proposed method can handle multiple objective functions simultaneously and shows good performance when the data dimension is large. The results indicate that in most scenarios, the proposed method outperforms its counterparts. It is also shown that the proposed algorithm is less sensitive to increasing the number of clusters and dimensions.
更多查看译文
关键词
Data clustering, Red deer algorithm, Evolutionary-based clustering, Grid-based clustering, RDA-based clustering, Unsupervised learning
AI 理解论文
溯源树
样例
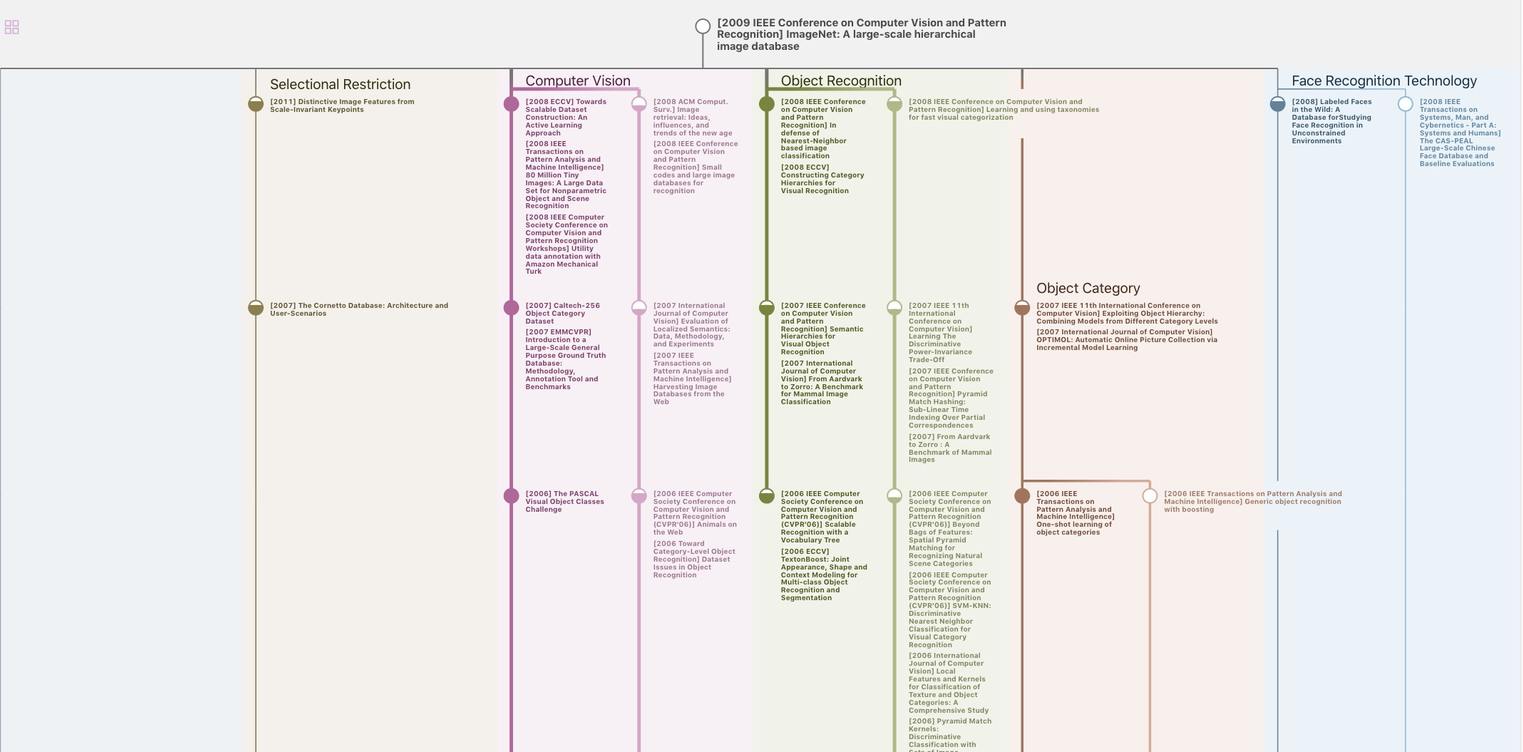
生成溯源树,研究论文发展脉络
Chat Paper
正在生成论文摘要