Map Based RNN Model for Proactive Prediction of Received Power Distribution in an Indoor Area
2023 17TH EUROPEAN CONFERENCE ON ANTENNAS AND PROPAGATION, EUCAP(2023)
摘要
This paper presents a proactive prediction method for received power distribution using GRU (Gated Recurrent Unit), which is one type of RNN (Recurrent Neural Network), as deep learning. In addition to the 50 most recent RSSI (Received Signal Strength Indicator) data acquired approximately every 0.1 seconds as input data, the distance and LoS/NLoS between transmitter and receiver at the prediction target position were used as a map data. As an output data, the median, 5%, and 95% values of RSSI after 5 seconds were predicted. The output data was derived using 50 points (about 5 seconds) of RSSI data. We used RSSI data of 5.6GHz band wireless LAN measured in an indoor environment for training data and validation data. With the proposed method, the RMSE (Root Mean Squared Error) for the validation data is approximately 1.4 dB, improving prediction accuracy by 1.4 dB and 0.6 dB for prediction using the current observed value and prediction using only the latest RSSI, respectively.
更多查看译文
关键词
propagation, received power prediction, deep learning, RNN, GRU
AI 理解论文
溯源树
样例
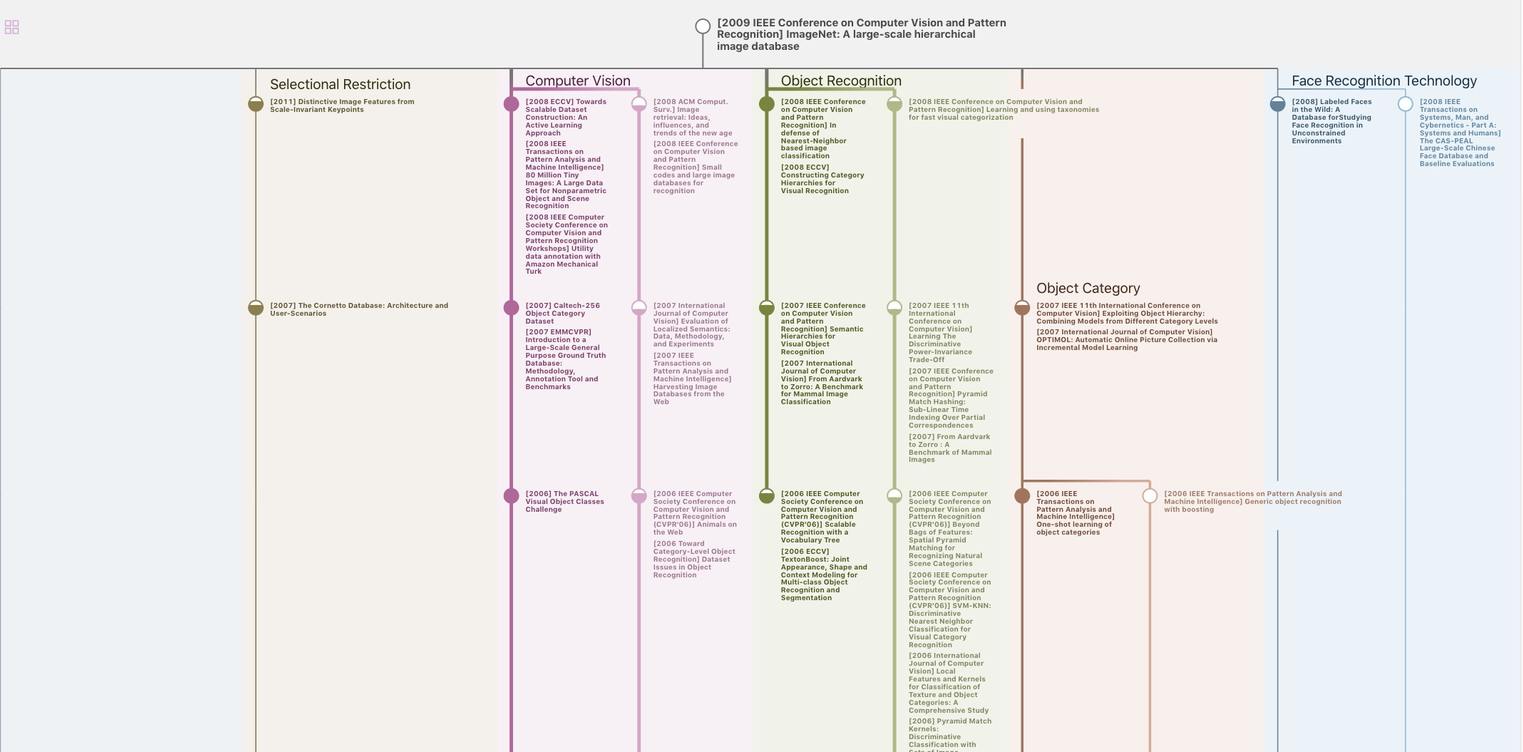
生成溯源树,研究论文发展脉络
Chat Paper
正在生成论文摘要