Finding robust minimizer for non-convex phase retrieval
INVERSE PROBLEMS AND IMAGING(2024)
摘要
The phase retrieval task has received considerable attention in recent years. Here, phase retrieval refers to the problem of recovering the clear image from magnitude-only data of its Fourier transform, or other linear transforms. As the observed magnitude signal is usually corrupted by heavy noise, retrieving the original object is rather difficult. In this paper, we investigate a regularization-based framelet method to recover the phase information and alleviate the influence of noise. Indeed, since phase retrieval is usually modeled by a non-convex model, how to find the solution efficiently is an intricate challenge. For this reason, we first reformulate the objective function as the difference between two convex functions. By introducing the boosted difference of convex algorithm (BDCA), the proposed scheme has good performance in handling the phase retrieval problem. Theoretically, we also present the convergence analysis of the numerical algorithm. To exhibit the effectiveness of our approach, we consider two classical regularizers with the proposed framework. Besides, two different measurement models are carefully studied to illustrate the robustness of our scheme. Numerical experiments demonstrate clearly that the proposed framelet method is effective and robust in tackling the non-convex phase retrieval task.
更多查看译文
关键词
Phase retrieval,non-convex model,BDCA,total variation,tight frame
AI 理解论文
溯源树
样例
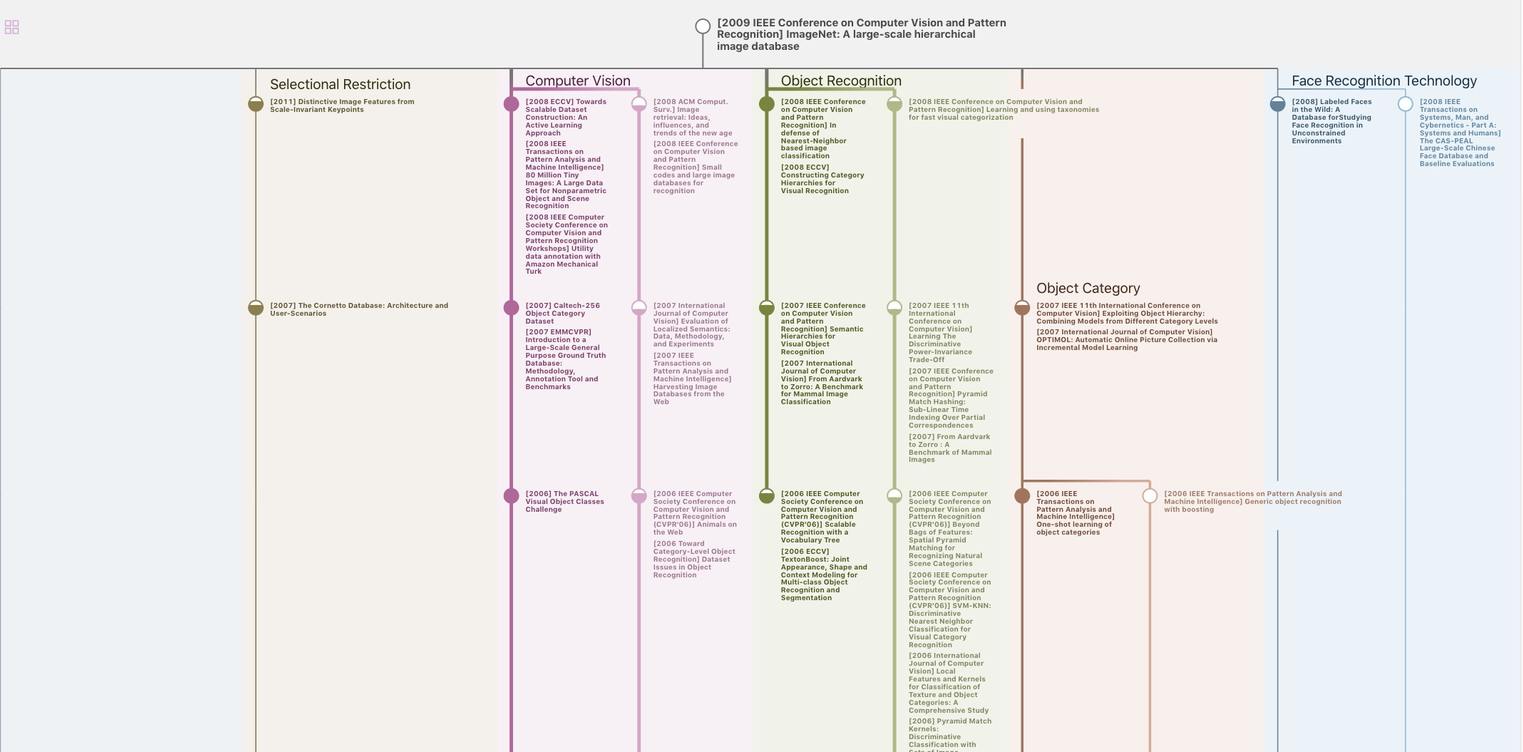
生成溯源树,研究论文发展脉络
Chat Paper
正在生成论文摘要