A Physics-Induced Deep Learning Scheme for Electromagnetic Inverse Scattering
IEEE TRANSACTIONS ON MICROWAVE THEORY AND TECHNIQUES(2024)
摘要
This article studies the full-wave electromagnetic inverse scattering (EMIS) problem that aims to retrieve the permittivities of dielectric scatterers based on the knowledge of measured scattered fields. A physics-induced deep learning (DL) scheme termed contrastive learning-based subspace optimization and semantic segmentation-assisted reconstruction (CLSO-SSR) is proposed to solve this problem. The proposed CLSO-SSR model is novel in aspects: 1) a contrastive learning network to provide an initial estimate of the contrast source so that both the probability of the local optima and the time consumption of the inversion process are greatly reduced and 2) a semantic segmentation network with an attention mechanism to mitigate the nonlinearity caused by the multiple scattering effects. Extensive numerical experiments are carried out to show that the proposed CLSO-SSR scheme can significantly improve upon the permittivity reconstruction performance as opposed to existing alternatives.
更多查看译文
关键词
Electromagnetic interference,Imaging,Permittivity,Iterative methods,Image reconstruction,Microwave theory and techniques,Microwave imaging,Contrastive learning (CL),deep learning (DL),electromagnetic inverse scattering (EMIS),semantic segmentation
AI 理解论文
溯源树
样例
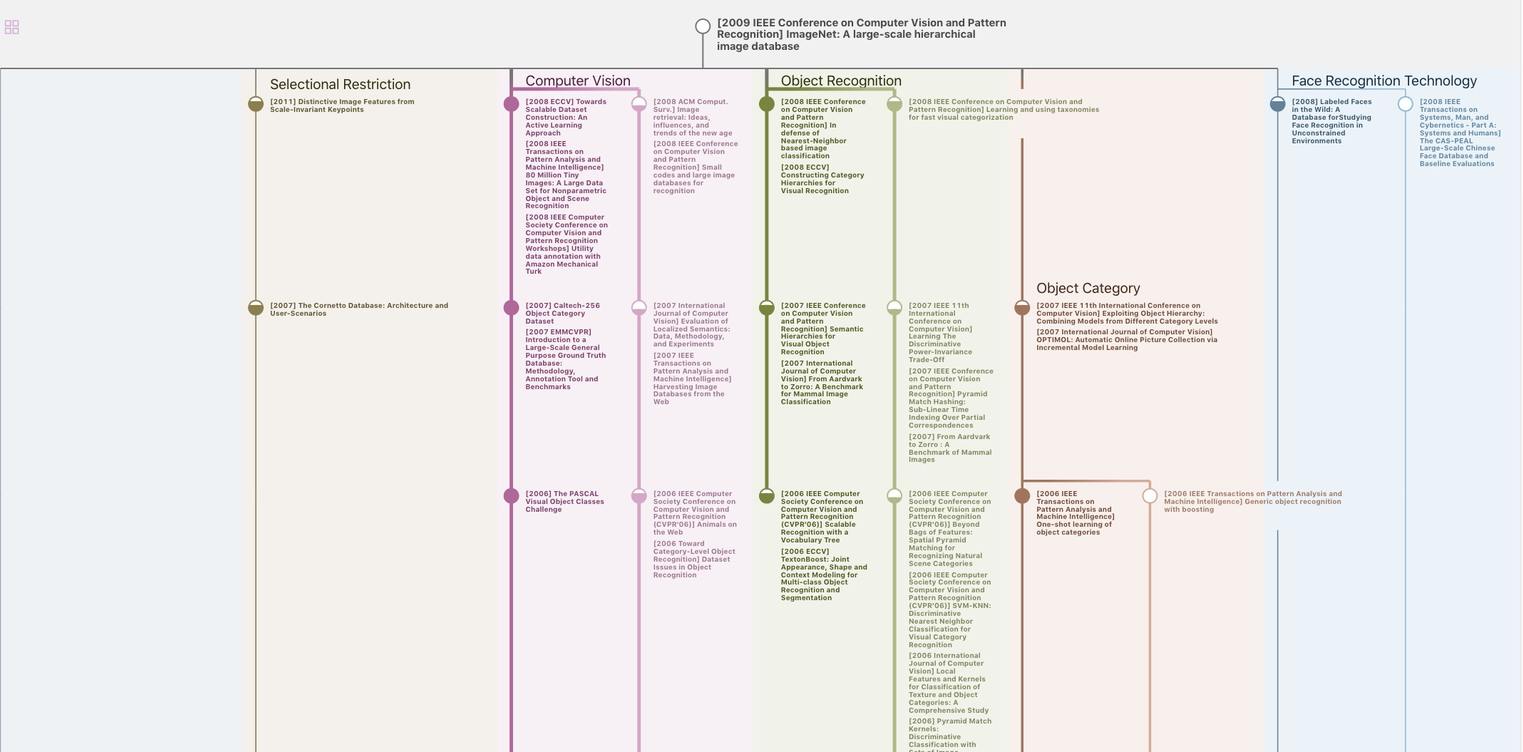
生成溯源树,研究论文发展脉络
Chat Paper
正在生成论文摘要