A Customized Meta-Learning Framework for Diagnosing New Faults From Unseen Working Conditions With Few Labeled Data
IEEE-ASME TRANSACTIONS ON MECHATRONICS(2023)
摘要
Few-shot fault diagnosis aims to detect novel faults with only a few labeled samples in each category. Most of the few-shot learning (FSL)-based fault diagnosis models use meta-learning frameworks because of their effectiveness and simplicity. However, these models often fail to be generalized in unseen working conditions that exhibit domain shifts. This study focuses on the few-shot fault diagnosis while addressing the challenges in domain-shift scenarios by developing a customized meta-learning framework, which consists of three key contributions: 1) a fused deep feature learning strategy is designed using multidomain signals in time, frequency, and time-frequency to extract more discriminative features from a few labeled samples; 2) a domain shift-learned feature transformation layer is introduced by modulating the feature activations with affine transformations into the meta-learner to tackle challenges due to domain shifts under unseen working conditions; and 3) a Mahalanobis distance-based metric function is constructed leveraging an additional neural network to learn the spread variance of each fault pattern to ensure an accurate and robust label prediction. The proposed framework is tested using real-world datasets and the ablation study demonstrates the effectiveness of its key components. The results also show that the proposed framework outperforms the state-of-the-art FSL algorithms that fail to consider the domain-shift scenarios.
更多查看译文
关键词
Domain shift,fault diagnosis,few-shot learning (FSL),meta-learning,novelty detection
AI 理解论文
溯源树
样例
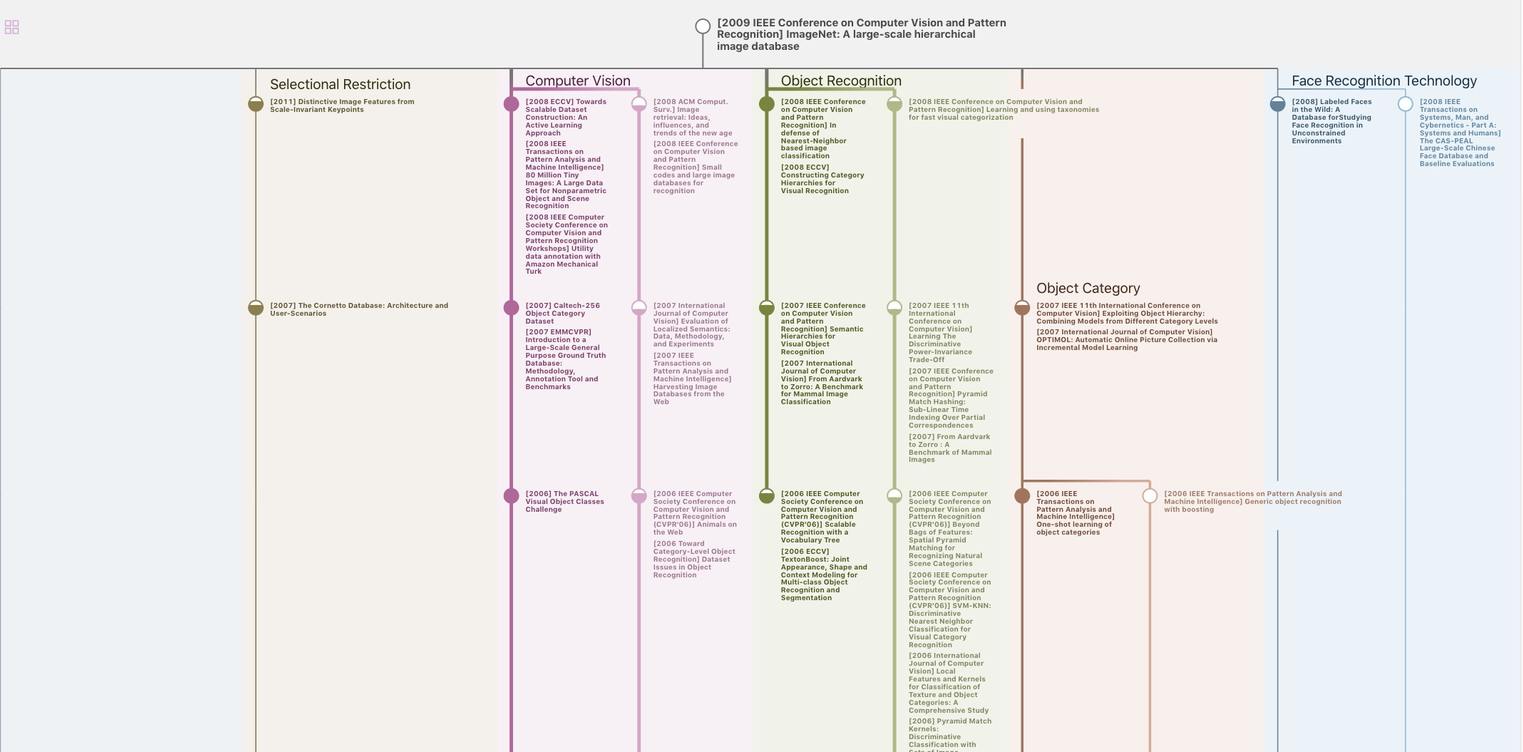
生成溯源树,研究论文发展脉络
Chat Paper
正在生成论文摘要