Improved environmental chemistry property prediction of molecules with graph machine learning
GREEN CHEMISTRY(2023)
摘要
Rapid prediction of environmental chemistry properties is critical for the green and sustainable development of the chemical industry and drug discovery. Machine learning methods can be applied to learn the relations between chemical structures and their environmental impact. Graph machine learning, by learning the representations directly from molecular graphs, may have better predictive power than conventional feature-based models. In this work, we leveraged graph neural networks to predict the environmental chemistry properties of molecules. To systematically evaluate the model performance, we selected a representative list of datasets, ranging from solubility to reactivity, and compared them directly to commonly used methods. We found that the graph model achieved near state-of-the-art accuracy for all tasks and, for several, improved the accuracy by a large margin over conventional models that rely on human-designed chemical features. This demonstrates that graph machine learning can be a powerful tool to perform representation learning for environmental chemistry. Further, we compared the data efficiency of conventional feature-based models and graph neural networks, providing guidance for model selection dependent on the size of datasets and feature requirements.
更多查看译文
关键词
graph machine learning,molecules,chemistry,machine learning,prediction
AI 理解论文
溯源树
样例
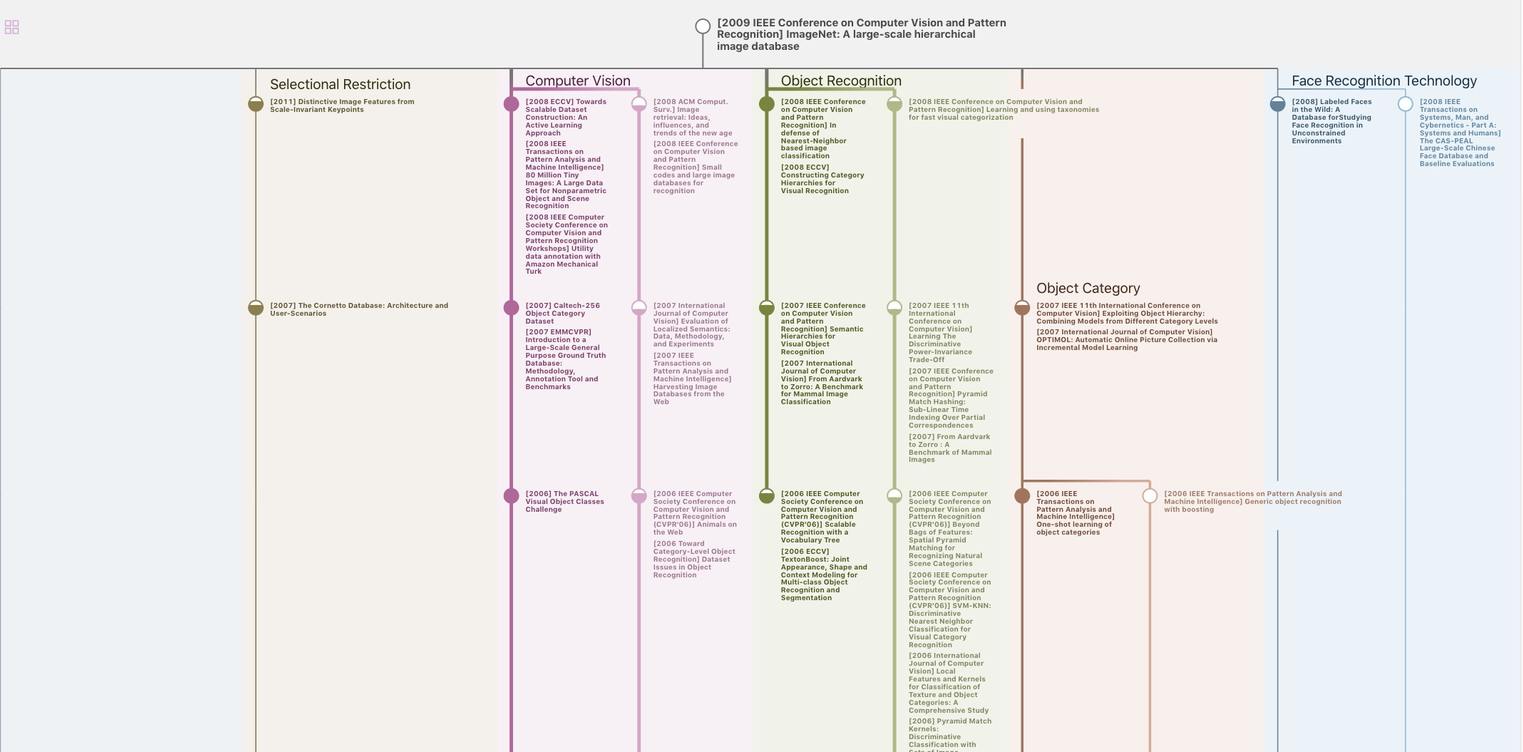
生成溯源树,研究论文发展脉络
Chat Paper
正在生成论文摘要