Finding Paths With Least Expected Time in Stochastic Time-Varying Networks Considering Uncertainty of Prediction Information
IEEE TRANSACTIONS ON INTELLIGENT TRANSPORTATION SYSTEMS(2023)
摘要
An increasing number of vehicles cause the deteriorating congestion problem, which leads to the excessive time spent in commuting. Thus, finding fast driving paths gathers growing interest from travelers and governments. However, effective route planning in traffic networks is deemed to be a considerable challenge due to the complex variations of traffic conditions. The existing traffic state aware routing strategies include two main categories: trip planning based on the traffic state before departure or short-term traffic prediction. The former may incur newly emerged jams because of the rapid en-route evolution of traffic state. The nonnegligible prediction errors would make the latter method deviate from the optimal paths. Moreover, these two deterministic routing strategies may easily result in late arrival for important events. To address this nontrivial problem, we propose a sophisticated route planning approach. Specifically, our method employs empirical observations of traffic prediction results to develop statistical models of error distributions. Then, the empirical error distributions are incorporated with the speed prediction values to constitute the stochastic and time-varying (STV) road network model. Thirdly, the least expected time (LET) routing problem in this STV network is defined. To determine the LET paths, we develop the time-varying K-fastest paths algorithm to generate the candidate paths and the discrete numerical method to compare their expected travel time. Finally, we collect the real-world traffic speed and trajectory data for experiments. The comparison results validate that our approach achieves the best performance and the improvement over other baselines is significant in peak hours.
更多查看译文
关键词
Route planning,reliable path,statistical analysis,traffic prediction,travel time uncertainty
AI 理解论文
溯源树
样例
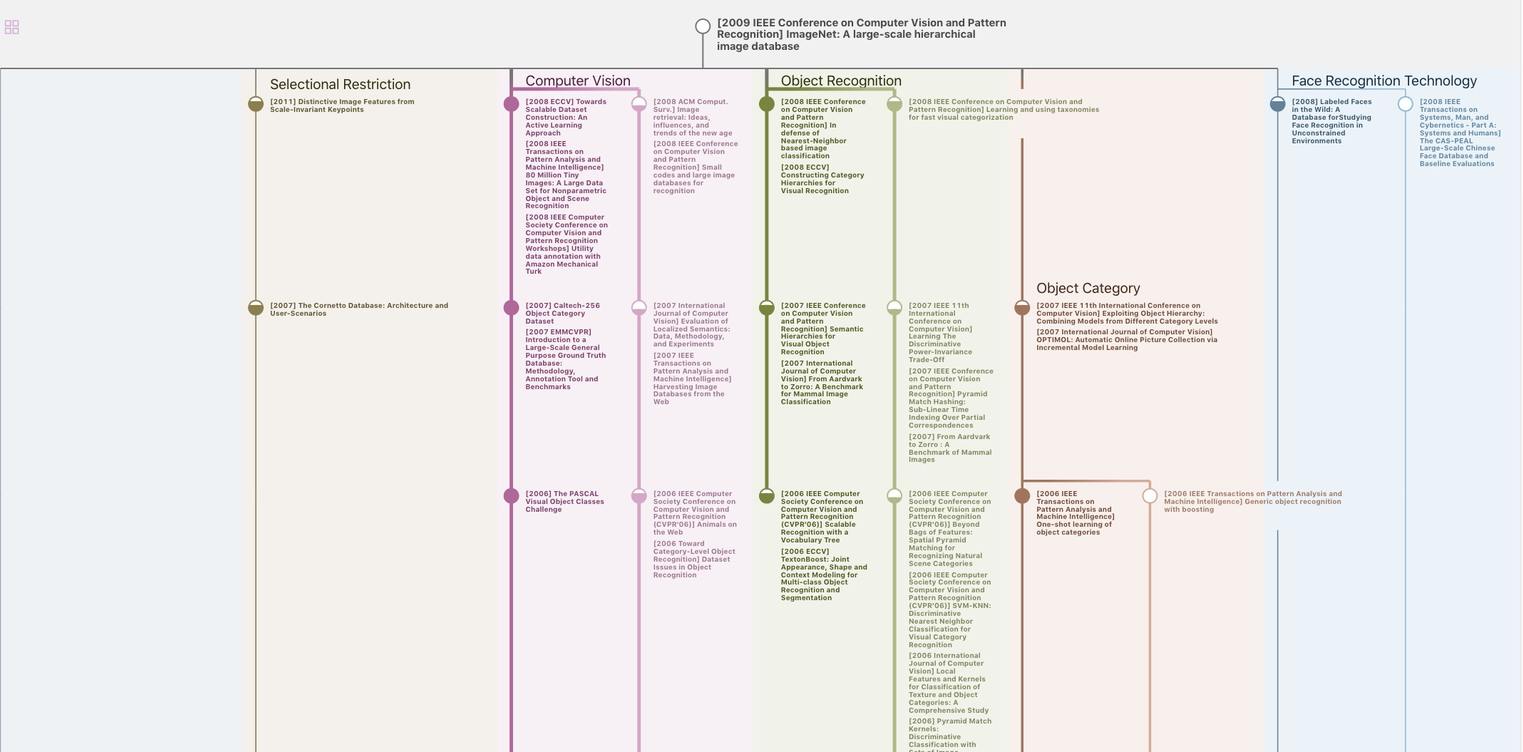
生成溯源树,研究论文发展脉络
Chat Paper
正在生成论文摘要