Generalised Mutual Information: a Framework for Discriminative Clustering
CoRR(2023)
摘要
In the last decade, recent successes in deep clustering majorly involved the Mutual Information (MI) as an unsupervised objective for training neural networks with increasing regularisations. While the quality of the regularisations have been largely discussed for improvements, little attention has been dedicated to the relevance of MI as a clustering objective. In this paper, we first highlight how the maximisation of MI does not lead to satisfying clusters. We identified the Kullback-Leibler divergence as the main reason of this behaviour. Hence, we generalise the mutual information by changing its core distance, introducing the Generalised Mutual Information (GEMINI): a set of metrics for unsupervised neural network training. Unlike MI, some GEMINIs do not require regularisations when training as they are geometry-aware thanks to distances or kernels in the data space. Finally, we highlight that GEMINIs can automatically select a relevant number of clusters, a property that has been little studied in deep discriminative clustering context where the number of clusters is a priori unknown.
更多查看译文
关键词
generalised mutual information,clustering,discriminative
AI 理解论文
溯源树
样例
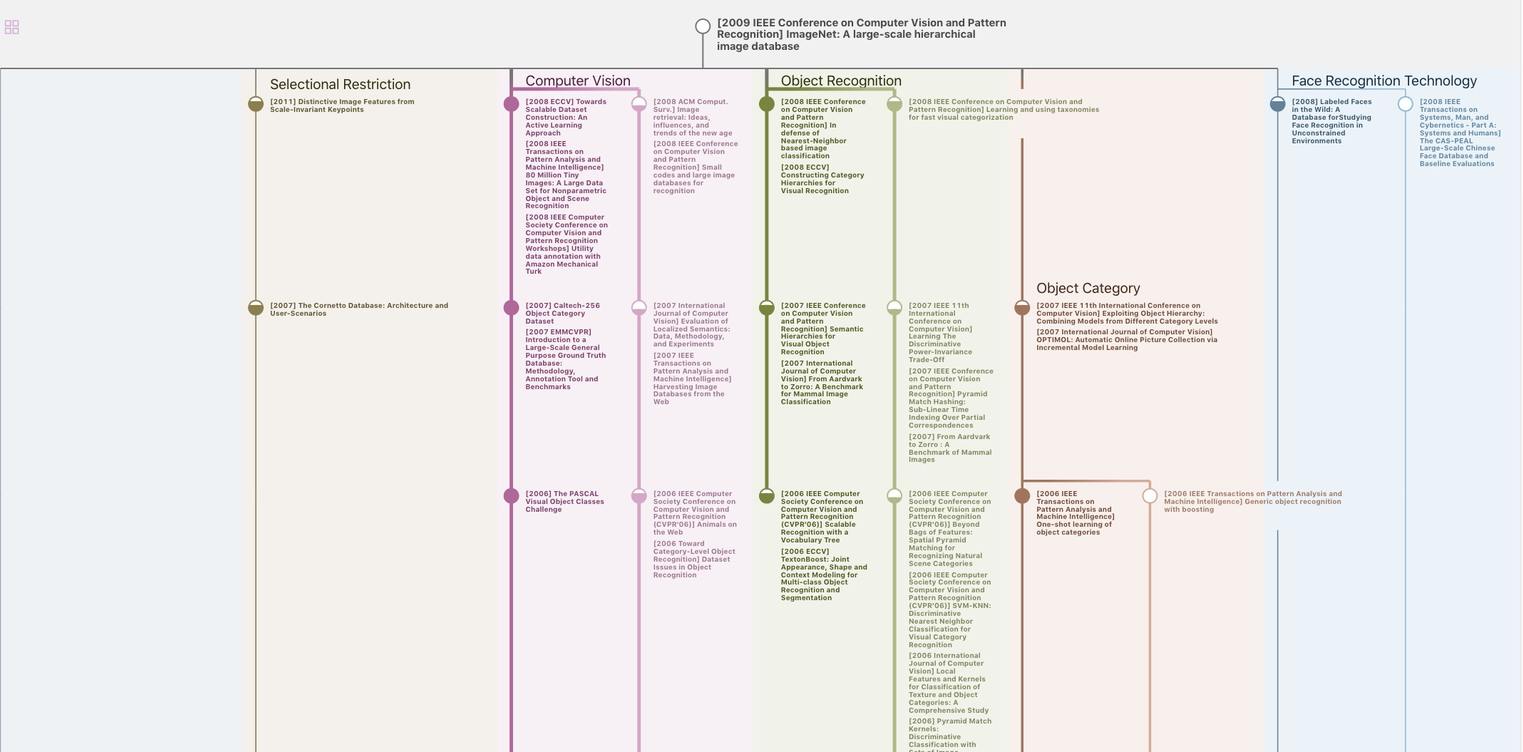
生成溯源树,研究论文发展脉络
Chat Paper
正在生成论文摘要