Channel Boosting, Cross-Layer Feature Integration, and Multi-Scale Classification for Fine-Grained Visual Classification
2023 4th International Conference on Information Science, Parallel and Distributed Systems (ISPDS)(2023)
摘要
Fine-grained visual classification (FGVC) is extremely challenging compared to basic-level classification. Although some attention-based methods have achieved promising results, they commonly fail to explore the benefits of multi-scale visual information and neglect the importance of information from intermediate layers in the classification network. In this work, we propose a unified framework for FGVC that integrates channel attention, spatial attention, and cross-layer feature interaction. We also demonstrate the significance of information from intermediate layers. Firstly, we introduce a channel boosting module that enhances the most salient parts in the feature maps. Secondly, we enhance and classify the outputs of intermediate layers, emphasizing their importance in the classification task. Furthermore, we introduce a cross-layer feature interaction module capable of integrating information from different feature layers. Lastly, the suggested framework does not require any bounding boxes or part annotations and can be trained seamlessly end-to-end. Extensive experiments show that our proposed method achieves competitive performance on three fine-grained classification benchmarks.
更多查看译文
关键词
Fine-grained Classification,Attention mechanism,Region boosting,Deep learning
AI 理解论文
溯源树
样例
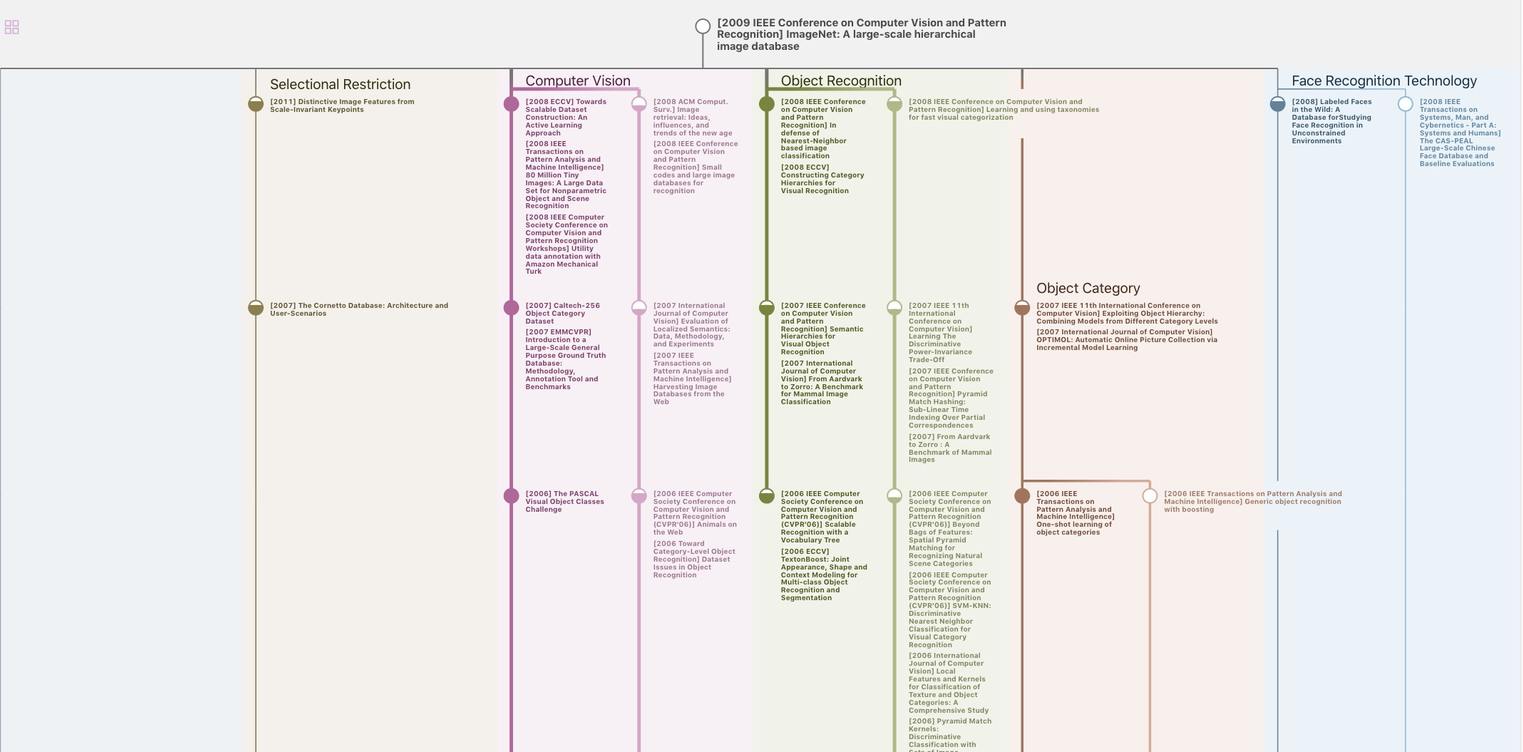
生成溯源树,研究论文发展脉络
Chat Paper
正在生成论文摘要