LoRA-like Calibration for Multimodal Deception Detection using ATSFace Data
CoRR(2023)
摘要
Recently, deception detection on human videos is an eye-catching techniques and can serve lots applications. AI model in this domain demonstrates the high accuracy, but AI tends to be a non-interpretable black box. We introduce an attention-aware neural network addressing challenges inherent in video data and deception dynamics. This model, through its continuous assessment of visual, audio, and text features, pinpoints deceptive cues. We employ a multimodal fusion strategy that enhances accuracy; our approach yields a 92\% accuracy rate on a real-life trial dataset. Most important of all, the model indicates the attention focus in the videos, providing valuable insights on deception cues. Hence, our method adeptly detects deceit and elucidates the underlying process. We further enriched our study with an experiment involving students answering questions either truthfully or deceitfully, resulting in a new dataset of 309 video clips, named ATSFace. Using this, we also introduced a calibration method, which is inspired by Low-Rank Adaptation (LoRA), to refine individual-based deception detection accuracy.
更多查看译文
关键词
multimodal,deception detection,attention mechanism,ensemble,calibration
AI 理解论文
溯源树
样例
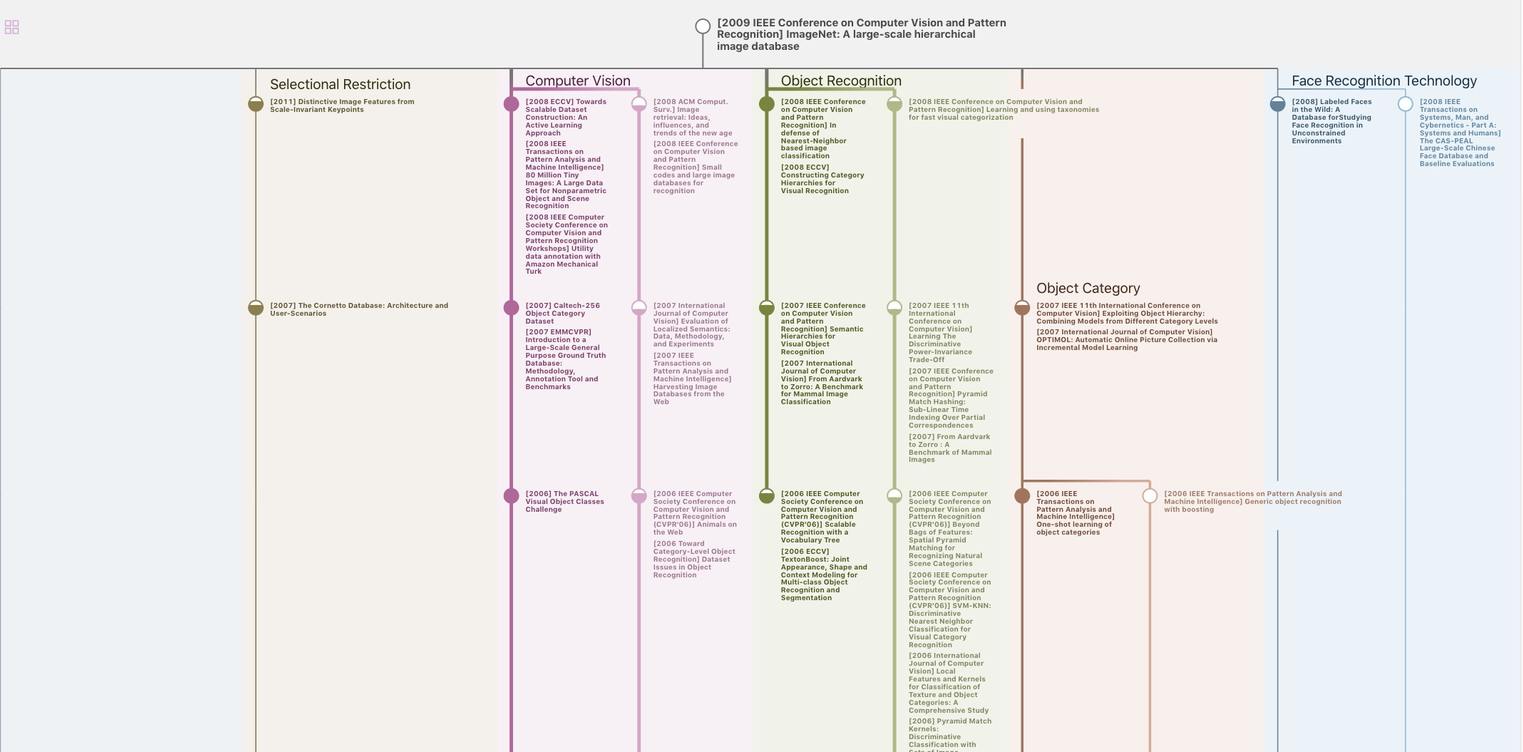
生成溯源树,研究论文发展脉络
Chat Paper
正在生成论文摘要