Synthesizing differentially private location traces including co-locations
INTERNATIONAL JOURNAL OF INFORMATION SECURITY(2023)
摘要
Privacy-preserving location synthesizers have been widely studied to perform private geo-data analysis. They have also been used for generating datasets for research or competitions. However, existing location synthesizers do not take into account the friendship information of users. Because friends tend to visit the same place at the same time in practice, a location synthesizer should consider such co-locations of friends to generate a more realistic dataset. In this paper, we propose a novel location synthesizer that generates location traces including co-locations of friends. Our location synthesizer models the information about the co-locations with two parameters: friendship probability and co-location count matrix . Our synthesizer generates a synthetic graph based on the friendship probability and then generates synthetic co-locations using the synthetic graph and the co-location count matrix. The two parameters in our synthesizer provide strong privacy guarantees—the friendship probability provides node differential privacy (DP) and the co-location count matrix provides user-level DP. We evaluate our synthesizer using two real datasets. Our experimental results show that our synthesizer preserves co-locations and other statistical features while providing DP with reasonable privacy budgets, e.g., 0.2-node DP and 2-user-level DP.
更多查看译文
关键词
Location synthesizer,Differential privacy,User-level DP,Node DP,Co-location
AI 理解论文
溯源树
样例
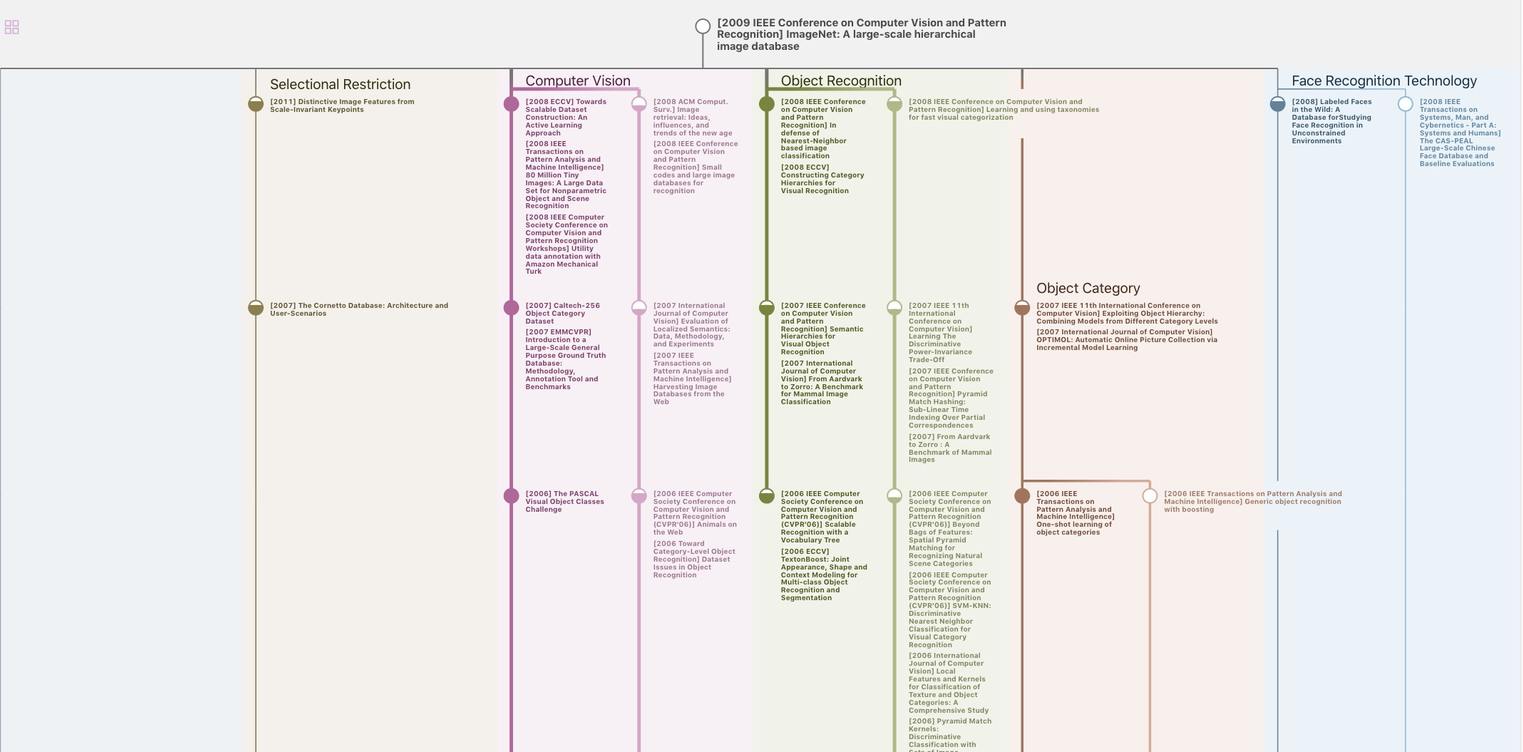
生成溯源树,研究论文发展脉络
Chat Paper
正在生成论文摘要