Enhancing PLM Performance on Labour Market Tasks via Instruction-based Finetuning and Prompt-tuning with Rules
CoRR(2023)
摘要
The increased digitization of the labour market has given researchers, educators, and companies the means to analyze and better understand the labour market. However, labour market resources, although available in high volumes, tend to be unstructured, and as such, research towards methodologies for the identification, linking, and extraction of entities becomes more and more important. Against the backdrop of this quest for better labour market representations, resource constraints and the unavailability of large-scale annotated data cause a reliance on human domain experts. We demonstrate the effectiveness of prompt-based tuning of pre-trained language models (PLM) in labour market specific applications. Our results indicate that cost-efficient methods such as PTR and instruction tuning without exemplars can significantly increase the performance of PLMs on downstream labour market applications without introducing additional model layers, manual annotations, and data augmentation.
更多查看译文
关键词
labour market tasks,plm performance,finetuning,instruction-based,prompt-tuning
AI 理解论文
溯源树
样例
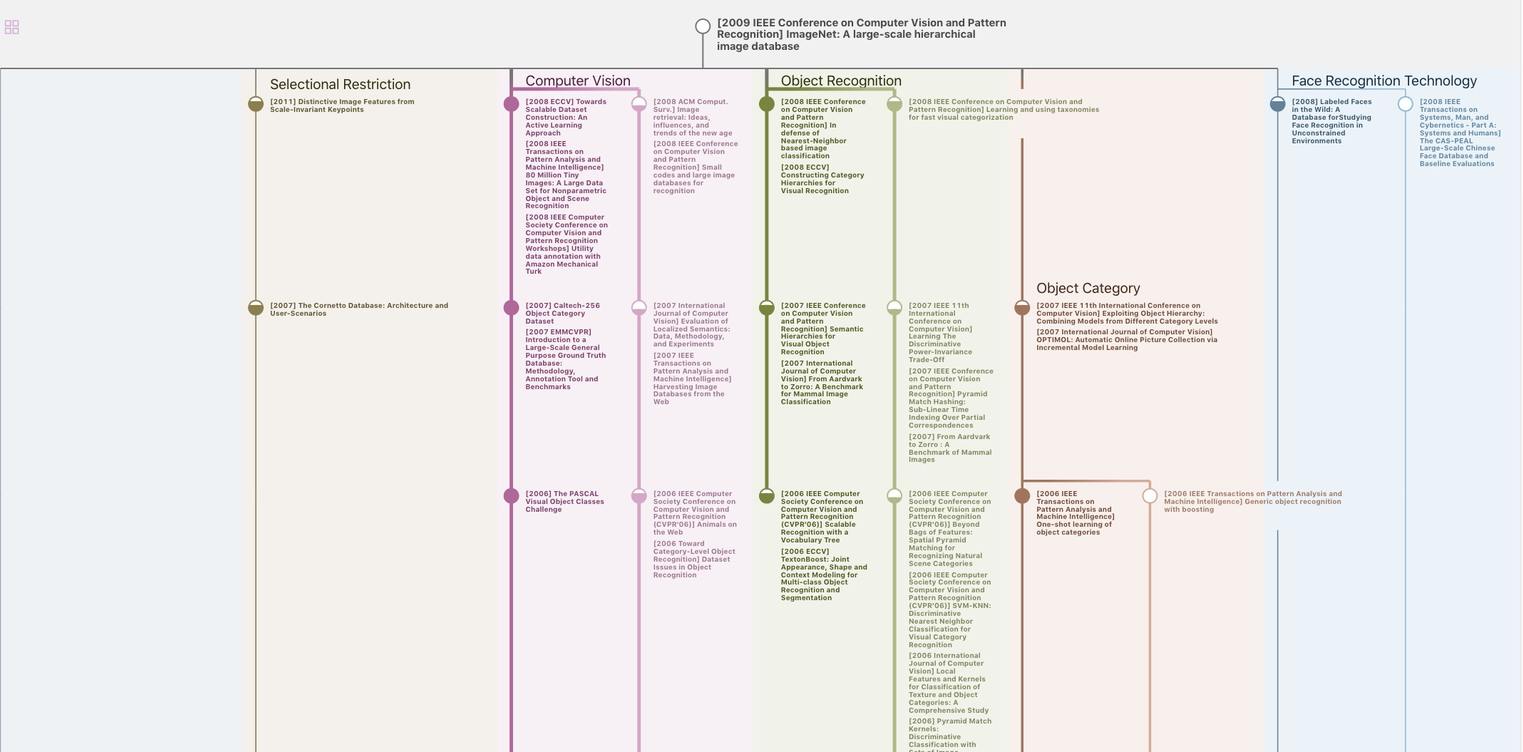
生成溯源树,研究论文发展脉络
Chat Paper
正在生成论文摘要