On the impact of deep neural network calibration on adaptive edge offloading for image classification.
J. Netw. Comput. Appl.(2023)
摘要
Edge devices can offload deep neural network (DNN) inference to the cloud to overcome energy or processing constraints. Nevertheless, offloading adds communication delay, which increases the overall inference time. An alternative is to use adaptive offloading based on early-exit DNNs. Early-exit DNNs have branches inserted at the output of given intermediate layers. These side branches provide confidence estimates. If the confidence level of the decision produced is sufficient, the inference is made by the side branch. Otherwise, the edge offloads the inference decision to the cloud, which implements the remaining DNN layers. Thus, the offloading decision depends on reliable confidence levels provided by the side branches at the device. This article provides an extensive calibration study on different datasets and early-exit DNNs for the image classification task. Our study shows that early-exit DNNs are often miscalibrated, overestimating their prediction confidence and making unreliable offloading decisions. To evaluate the impact of calibration on accuracy and latency, we introduce two novel application-level metrics and evaluate well-known DNN models in a realistic edge computing scenario. The results demonstrated that calibrating early-exit DNNs improves the probabilities of meeting accuracy and latency requirements.
更多查看译文
关键词
Early-exit deep neural networks,Edge computing,Deep neural network calibration,Edge offloading
AI 理解论文
溯源树
样例
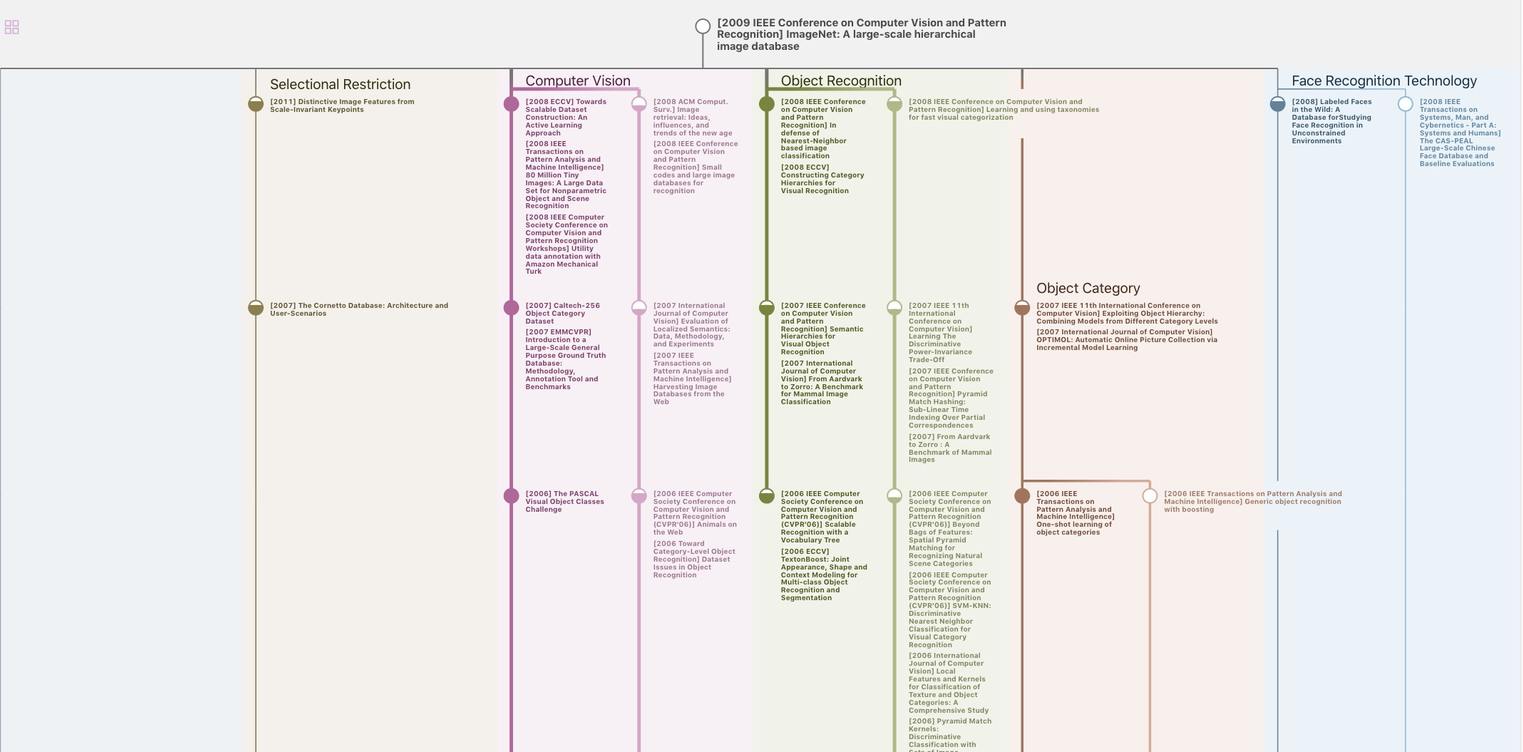
生成溯源树,研究论文发展脉络
Chat Paper
正在生成论文摘要