Situation-Aware Cluster and Quantization Level Selection Algorithm for Fast Federated Learning.
IEEE Internet Things J.(2023)
摘要
In federated learning (FL), which clients and quantization levels are selected for the deep model parameters has a significant impact on learning time as well as learning accuracy. This is not a trivial issue because it is also significantly affected by factors, such as computational power, communication capacity, and data distribution. Considering these factors, we formulate a joint optimization problem for clustering and selecting clusters with quantization levels. Due to the high complexity of the formulated problem, we propose a situation-aware cluster and quantization level selection (SITUA-CQ) algorithm. In this algorithm, the FL server first assembles clients into clusters to mitigate the impact of biased data distributions and determines the most suitable clusters and quantization levels based on their computing power and channel quality. Extensive simulation results show that SITUA-CQ can reduce the round time by up to 80.3% compared to conventional algorithms.
更多查看译文
关键词
Cluster and quantization level selection, clustering, federated learning (FL)
AI 理解论文
溯源树
样例
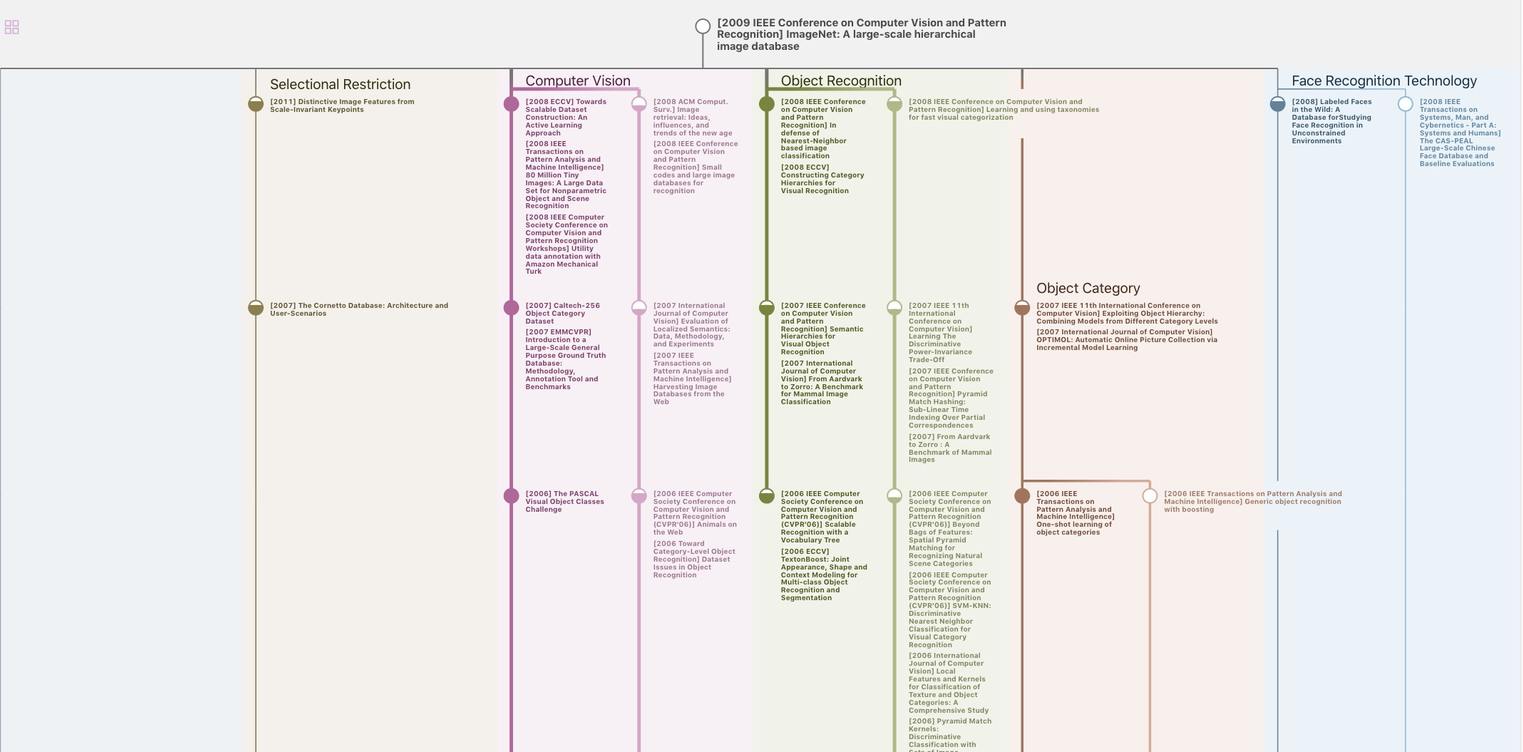
生成溯源树,研究论文发展脉络
Chat Paper
正在生成论文摘要