Cesarean Section Classification Using Machine Learning With Feature Selection, Data Balancing, and Explainability.
IEEE Access(2023)
摘要
Disease samples are naturally fewer than healthy samples which introduces bias in the training of machine learning (ML) models. Current study focuses in learning discriminating patterns between cesarean and non-cesarean phenomena based on a dataset consisting of 161 features of total 692 cesarean and 5465 non-cesarean samples which comes as four folds based on four different hospitals (hospital A, B, C and D). The dataset is noisy, contains missing values, features are at different scales and above all, 161 features are quite a large in number and risks containing unnecessary information with respect to learning to separate the C-section class from non-cesarean.This study introduced a data pre-processing pipeline, resolving issues with data imbalance, handling missing values, identifying and deleting outliers, etc. A novel ensemble model is proposed which is able to consistently perform better irrespective of data volumes (data fold A, A+B, A+B+C and A+B+C+D) and pre-processing pipeline and achieved 96-99% accuracy across data volumes. Finally, the proposed model’s decision-making was explained in terms of prominent features where higher values of features like Episiotomy, age of women and Fetal intrapartum pH accounts for causing C-section.
更多查看译文
关键词
Cesarean section,feature selection,data balancing,machine learning,explainable AI
AI 理解论文
溯源树
样例
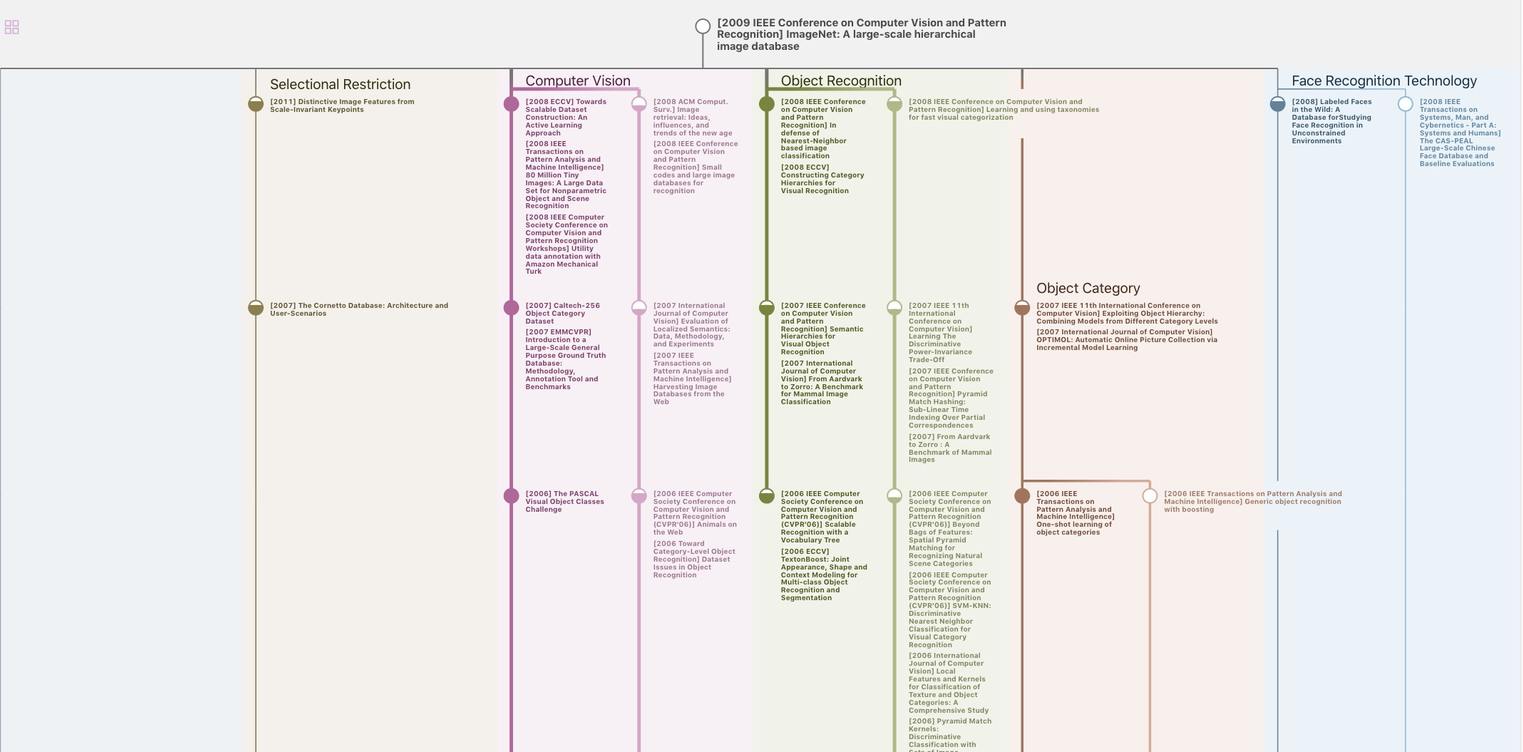
生成溯源树,研究论文发展脉络
Chat Paper
正在生成论文摘要