Lightweight Open Data Assimilation of Pan-European Urban Air Quality
IEEE ACCESS(2023)
摘要
The number of ambient air quality monitoring stations is growing globally, driven by the need to quantify potential health risks posed by air pollution on urban populations. Reliable, robust and interoperable air quality monitoring requires observations with consistent accuracy and low amounts of missing data. In practice, this is challenging to achieve due to the measurement limitations and complexity of the physical phenomena. Data assimilation methods are widely used to fill missing or faulty observations and improve data quality by combining observations from fixed air quality monitoring ground stations with large-scale numerical models. A further advantage of data assimilation is that it can decrease costs by reusing existing open government data. A key requirement for assimilation is that uncertainty estimates are available for both measurements and model data. However, this poses a major bottleneck for widespread data assimilation with open data because uncertainty estimates are frequently unavailable. Additional challenges addressed in this work include the needs to impute missing data and process observations and model simulation results at different temporal and spatial scales. To address these challenges, we have developed novel, lightweight data assimilation algorithms based on recursive least-squares. The algorithms provide a fully data-driven way to estimate unknown uncertainties by defining the weights of the input data sources using least-squares data assimilation. The lightweight data assimilation algorithms can be executed to update the current state estimate in near real-time scenarios to improve the accuracy, completeness, and precision of the analysis estimate. A sensitivity analysis is conducted using synthetic data based on logistic maps with increasing noise levels. In addition, the proposed assimilation algorithms are applied to large-scale open pan-European air quality monitoring station data. The data were obtained from 86 stations for CO, 593 stations for NO2, 462 stations for O-3, 137 stations for SO2, 254 stations for PM2.5, and 445 stations for PM10 in the period from 2022-01-27 01:00:00 to 2022-02-25 15:00:00 from the European Environmental Agency (EEA) and corresponding simulation results from the System for Integrated modeLling of Atmospheric composition (SILAM, global, version 5.7, FRC forecasts at the surface). The proposed lightweight data assimilation methods were found suitable to improve the completeness (filling in all missing data), accuracy (taken as the RMSE between the assimilation results and ground station observations) and precision for all of the open air quality parameters evaluated in this work. Furthermore, the proposed lightweight assimilation algorithms may also provide new and cost-effective methods to improve the data quality of the growing number of Internet of Things (IoT) urban air quality sensors.
更多查看译文
关键词
Ambient air quality,data assimilation,environmental monitoring,open data,uncertainty quantification
AI 理解论文
溯源树
样例
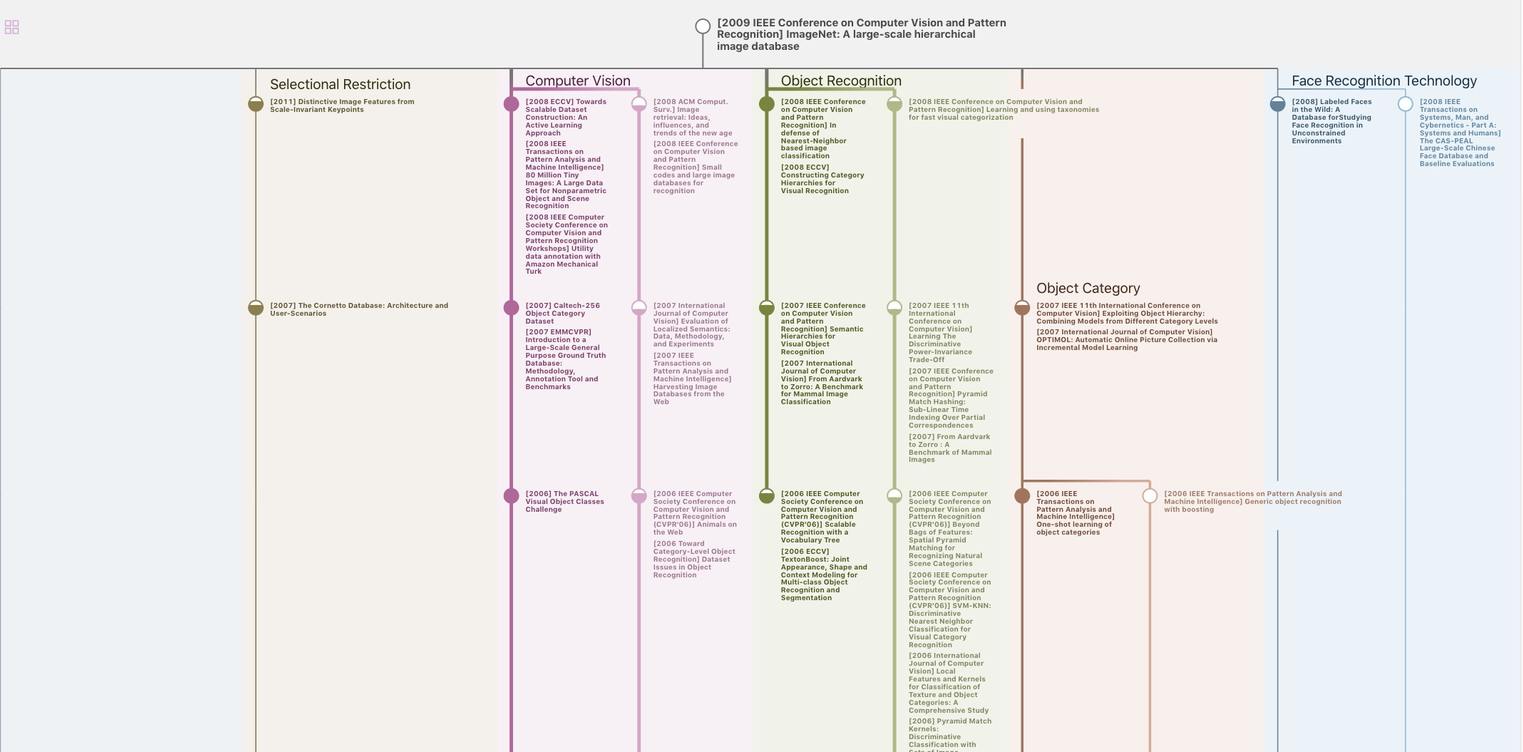
生成溯源树,研究论文发展脉络
Chat Paper
正在生成论文摘要