AIMC Modeling and Parameter Tuning for Layer-Wise Optimal Operating Point in DNN Inference.
IEEE Access(2023)
摘要
Analog in-memory computing (AIMC) has been utilized in convolutional neural networks (CNNs) edge inference engines to solve the memory bottleneck problem and increase efficiency. However, AIMC analog-to-digital converters (ADCs) restricted resolution imposes quantization of output activations that can reduce the accuracy without meticulous optimization. A study conducted output quantization calibration and obtained configurations with which low-resolution ADCs did not affect the accuracy. The configurations were layer-specific. Therefore, a real-time quantization adjustment was required. AIMC output quantization is adjusted by controlling analog gain entangling it with analog parameters and nonlinear functions. AIMC dynamic output quantization control without interrupting its operation has been an unsettled problem until now. This paper introduces a technique for imposing output quantization configurations obtained from calibration processes on AIMC through circuit parameters setup. The technique permits on-the-fly quantization adjustments enabling layer-wise calibration that increases achievable network accuracies on AIMC platforms. As a case study, we deployed the method on the AIMC macro of an artificial intelligence (AI) inference engine SoC platform with a RISC-V processor and hybrid DIgital-ANAlog accelerators (DIANA). We related its controllable circuit parameters with the quantization configuration in a look-up table. This case study has noteworthy side benefits in identifying platform limitations due to nonlinearities and design imperfections. These limitations are investigated, and design advice that is transferable to future AIMC designs is provided to avoid imperfections such as mismatch, bias voltage drop, and interconnect delay. In addition, the study of output quantization from different levels of abstraction leads to design guidelines to facilitate dynamic quantization control during the application phase.
更多查看译文
关键词
Analog in-memory computing (AIMC), deep neural network (DNN), convolutional neural network (CNN), application-specific integrated circuit (ASIC), artificial intelligence hardware acceleration, modeling, characterization, quantization
AI 理解论文
溯源树
样例
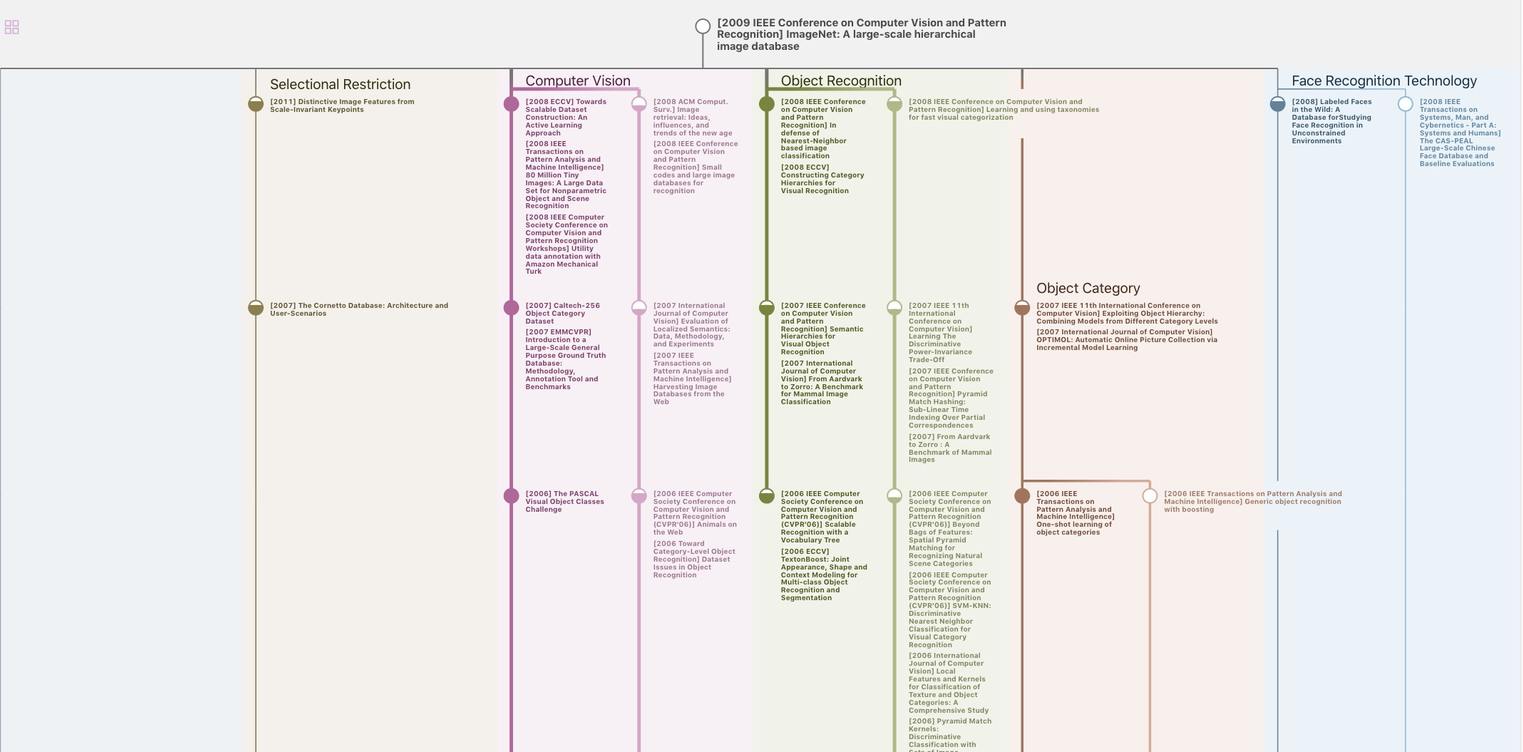
生成溯源树,研究论文发展脉络
Chat Paper
正在生成论文摘要