Detection of Denial-of-Service Attack in Wireless Sensor Networks: A Lightweight Machine Learning Approach
IEEE Access(2023)
摘要
The characteristics and performance of wireless sensor networks (WSNs) are the main reasons for their rapid expansion in various fields. However, these networks are extremely susceptible to multiple security assaults, including denial-of-service (DoS) attacks, which are among the most prevalent in these networks. This study sheds light on WSN restrictions, weaknesses, and security threats with a focus on DoS attacks. Recent techniques for DoS attack detection have been investigated thoroughly, highlighting their achievements and limitations. This provides valuable insight into the current state of recent research in this field. Accordingly, this study proposes a lightweight machine learning detection approach based on a decision tree (DT) algorithm with the Gini feature selection method to detect DoS attacks in WSNs. An enhanced version of the WSN-DS dataset, developed by the author, was used to train and test the proposed approach. The proposed approach has shown good performance by achieving an accuracy rate of 99.5% with minimum overhead compared to random forest (RF), extreme gradient boosting (XGBoost), and k-nearest neighbor (KNN) classifiers. It only takes 9.7%, 13%, and 2% of the processing time required by FR, XGBoost, and KNN respectively, which indicates that our proposed approach significantly outperforms these classifiers in terms of processing time. It is noteworthy that RF achieved an accuracy that was somewhat superior; however, the proposed approach greatly surpassed RF by taking only 9.7% of the RF processing time, which is an important factor in meeting WSN constraints.
更多查看译文
关键词
Deep learning,DoS attacks,cyber security,feature selection,IoT security,network security,machine learning,wireless sensor networks,WSN attacks,WSN constraints,WSN security
AI 理解论文
溯源树
样例
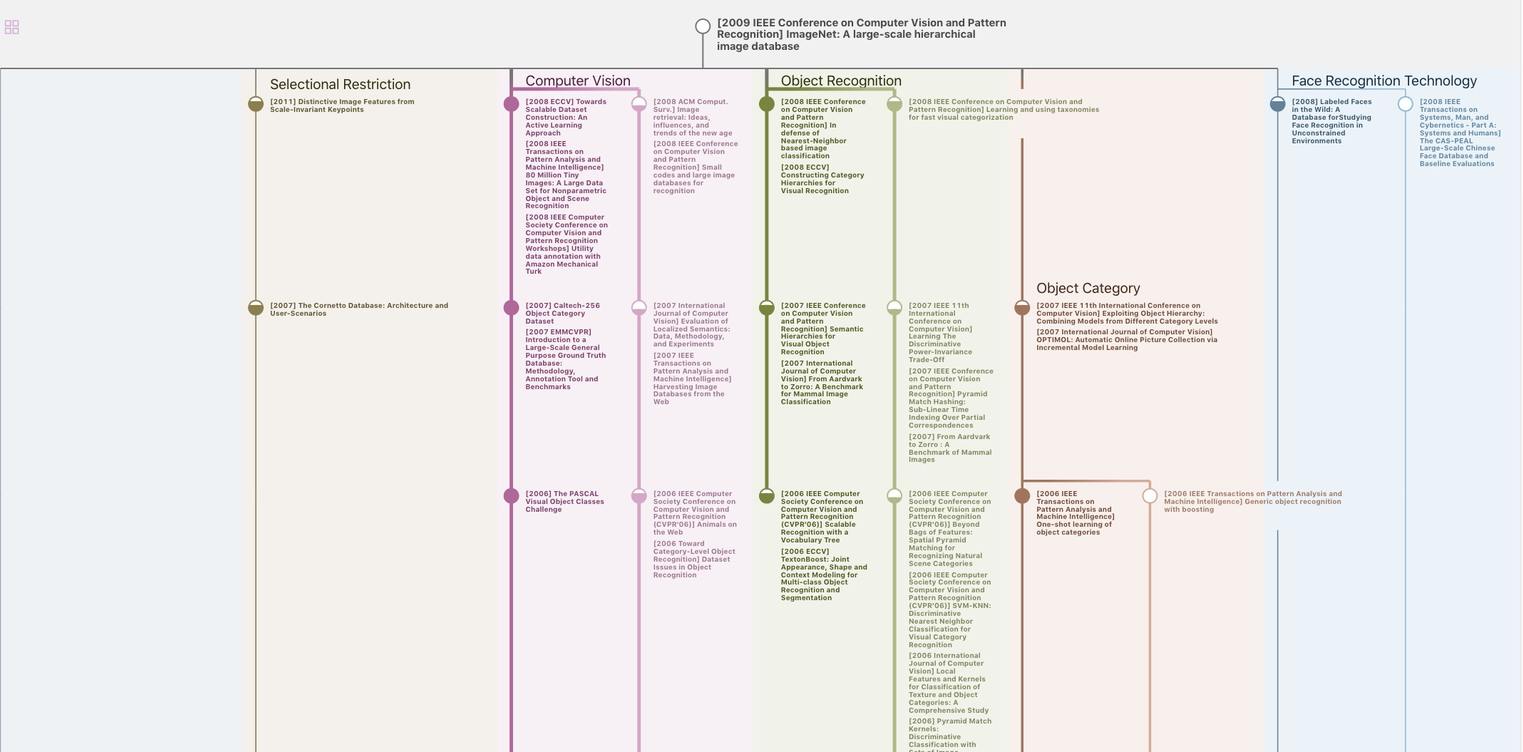
生成溯源树,研究论文发展脉络
Chat Paper
正在生成论文摘要