Spatiotemporal Multiscale Correlation Embedding With Process Variable Reorder for Industrial Soft Sensing.
IEEE Trans. Instrum. Meas.(2023)
摘要
Soft sensor techniques have been extensively utilized for predicting key variables in the process industry. To extract fine-grained and holistic features for efficient prediction, proper modeling strategies for multiscale spatial and temporal correlations are heavily desired. In this article, we summarize the multiscale correlations as a short and long temporal dependency, local and global spatial relevance, and spatiotemporal coupled correlations. Such correlations should be explicitly and comprehensively characterized as different key variables often require diverse or multiscale spatiotemporal features. To this end, a spatiotemporal multiscale correlation embedding (STMCE) framework is proposed for soft sensing with characterization and refining of multiscale spatial and temporal features. On the one hand, we design a reorder data patch embedding (RDPE) strategy to extract local spatiotemporal coupled correlations by reordering the permutation of process variables and embedding local correlations into high-dimensional vectors. On the other hand, we propose a global feature fusion and refining network (GFFRNet) via a self-attention mechanism and feature refining technique, which enables the model to effectively aggregate and privilege predictive features for key variable prediction. Superior to existing methods that partially focus on spatial or temporal dependencies, our approach explicitly models both local and global spatiotemporal coupled relevance under a multiscale perspective, encouraging the model to explore more fine-grained and comprehensive information from process data and further improve prediction accuracy. In comparison with ten baseline models, our STMCE empirically presents the state-of-the-art results on both benchmark and real-world industrial datasets, for example, obtaining a relative MAE reduction of 13.32% compared with the second-best method in the benchmark multiphase flow dataset.
更多查看译文
关键词
Process variable reorder,soft sensor,spatiotemporal multiscale correlation embedding (STMCE)
AI 理解论文
溯源树
样例
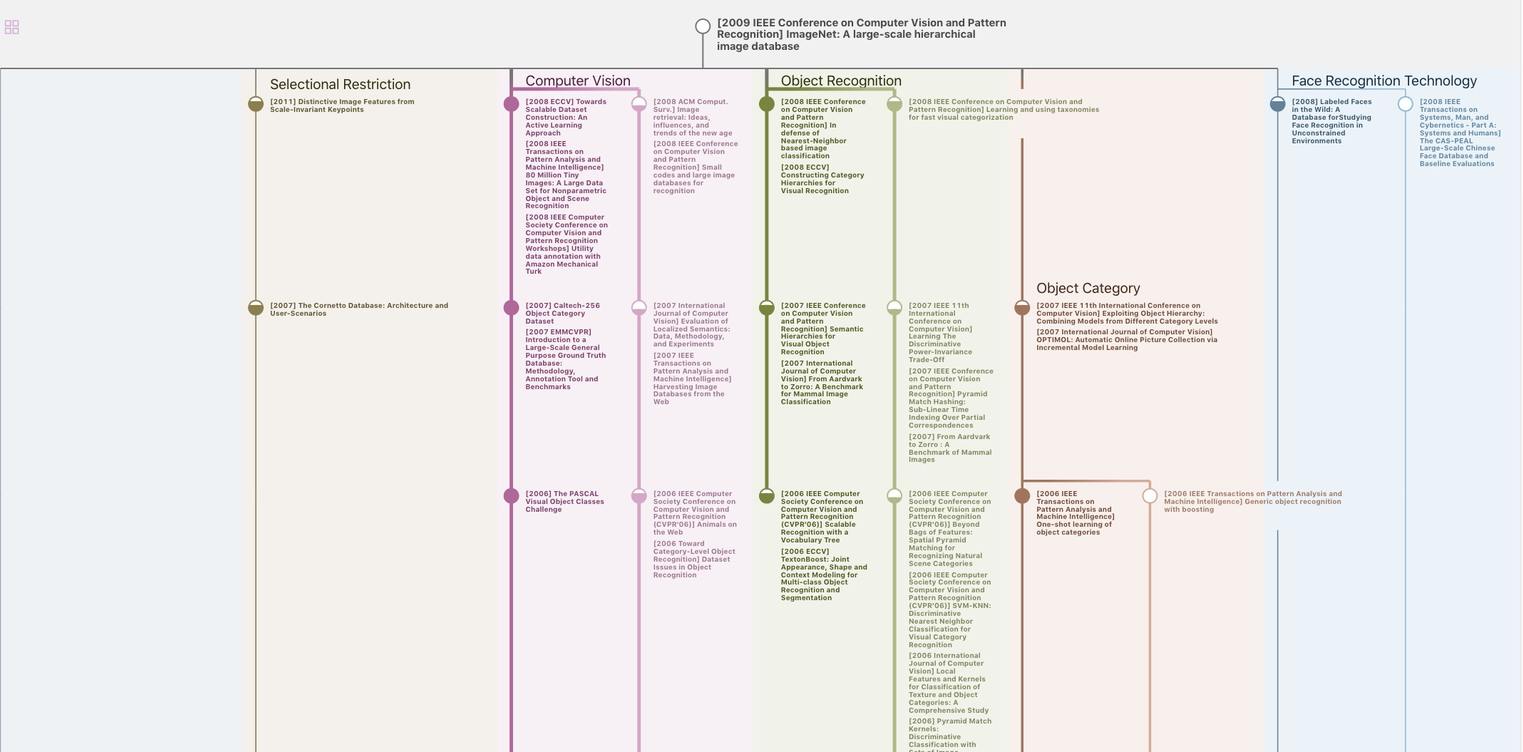
生成溯源树,研究论文发展脉络
Chat Paper
正在生成论文摘要