Adaptive Learning Against Muscle Fatigue for A-Mode Ultrasound-Based Gesture Recognition
IEEE Trans. Instrum. Meas.(2023)
摘要
It is evident that the state-of-the-art in multisensory hand gesture recognition indicates the superior performance of the A-mode ultrasound (AUS) modality over its counterparts. Nevertheless, the practical implementation of AUS-based gesture recognition in real-life scenarios is impeded by its susceptibility to muscle fatigue, thereby limiting its long-term applicability. Therefore, it is crucial to explore the role of muscle fatigue in the stability of AUS-based long-term human-machine interfaces. Endowing the recognition model with the adaptability to dynamic muscle fatigue state could potentially offer a viable solution to this issue. In this work, we propose an adaptive AUS-oriented neural network (A2USNet), which comprises two components: a convolutional neural network (CNN)-based adaptive feature extractor (AFE), and a category template-based adaptive classifier. A2USNet possesses the ability to update the feature representation and decision boundary in response to muscle fatigue, consequently mitigating performance deterioration. To validate the effectiveness of our proposed method, nine subjects were recruited to participate in the experiment, and they were asked to perform 15 subtle gestures for 16 trials, with AUS data collected. The experimental results demonstrate that the proposed method can yield a remarkable improvement of 26.79% in classification accuracy during the late stage of muscle fatigue, and the proposed method significantly outperforms conventional adaptive linear discriminant analysis (LDA) and incremental support vector machine (SVM). This study paves the way for establishing AUS as a viable option for long-term gesture recognition.
更多查看译文
关键词
Adaptive learning,A-mode ultrasound (AUS),muscle fatigue
AI 理解论文
溯源树
样例
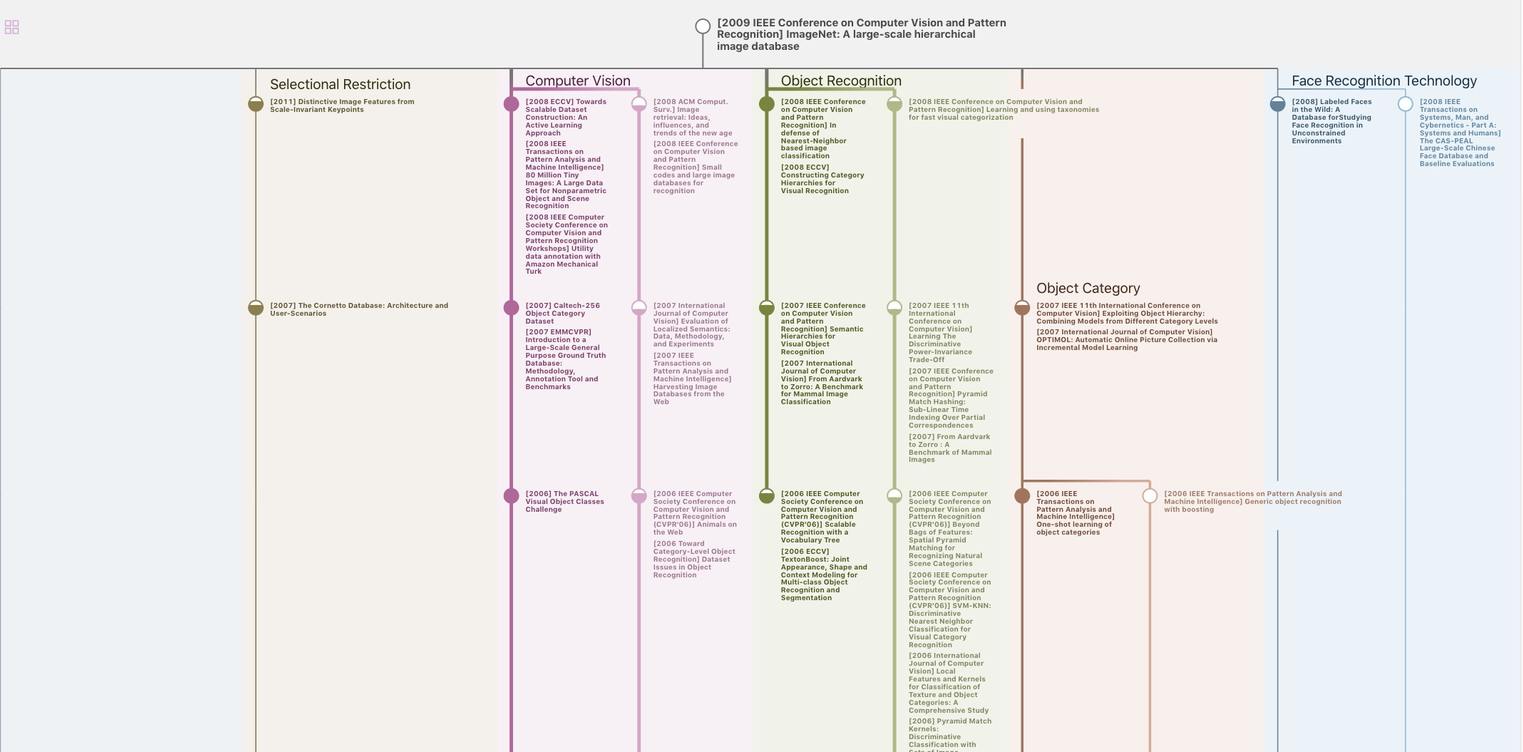
生成溯源树,研究论文发展脉络
Chat Paper
正在生成论文摘要