A Cross-Modality Feature Transfer Method for Target Detection in SAR Images.
IEEE Trans. Geosci. Remote. Sens.(2023)
摘要
Synthetic aperture radar (SAR) ship detection methods have achieved remarkable progress in recent years. However, unlike RGB images, the characteristics of SAR imaging will result in non-intuitive feature representations. Furthermore, due to the insufficient data of SAR images, existing methods relying on plenty of labeled SAR images may be hard to achieve promising performance. To address the aforementioned issues, a cross-modality feature transfer (CMFT) method is proposed in this article, which enhances feature representations in the SAR modality by transferring rich knowledge in the RGB modality. First, we propose a multilevel modality alignment network (MMAN), which encourages the model to effectively learn modality-invariant features and alleviate the large cross-modality discrepancies by aligning features from multilevels (scene level, local level, global level, and instance level). Second, to address the underperformance of samples with non-intuitive features in the modality alignment, we introduce a hard-sample supervision module (HSM) in the stage of feature extraction, which can thoroughly exploit the feature of hard-to-align samples by giving more optimization energy for them. Third, to enhance the discriminability of instance-level features, a feature complementary module (FCM) is customized to fully explore the potential complementary clues between instance-level features and context information for the instance-level feature alignment. Extensive experimental results demonstrate that the CMFT outperforms the state-of-the-art detectors. Compared to the baseline model, CMFT improves the accuracy by 3.1% mean average precision (mAP) on the SSDD dataset and 3.4% mAP on the HRSID dataset, demonstrating its superior SAR ship detection performance.
更多查看译文
关键词
Cross-modality feature transfer (CMFT),deep learning,synthetic aperture radar (SAR) ship detection
AI 理解论文
溯源树
样例
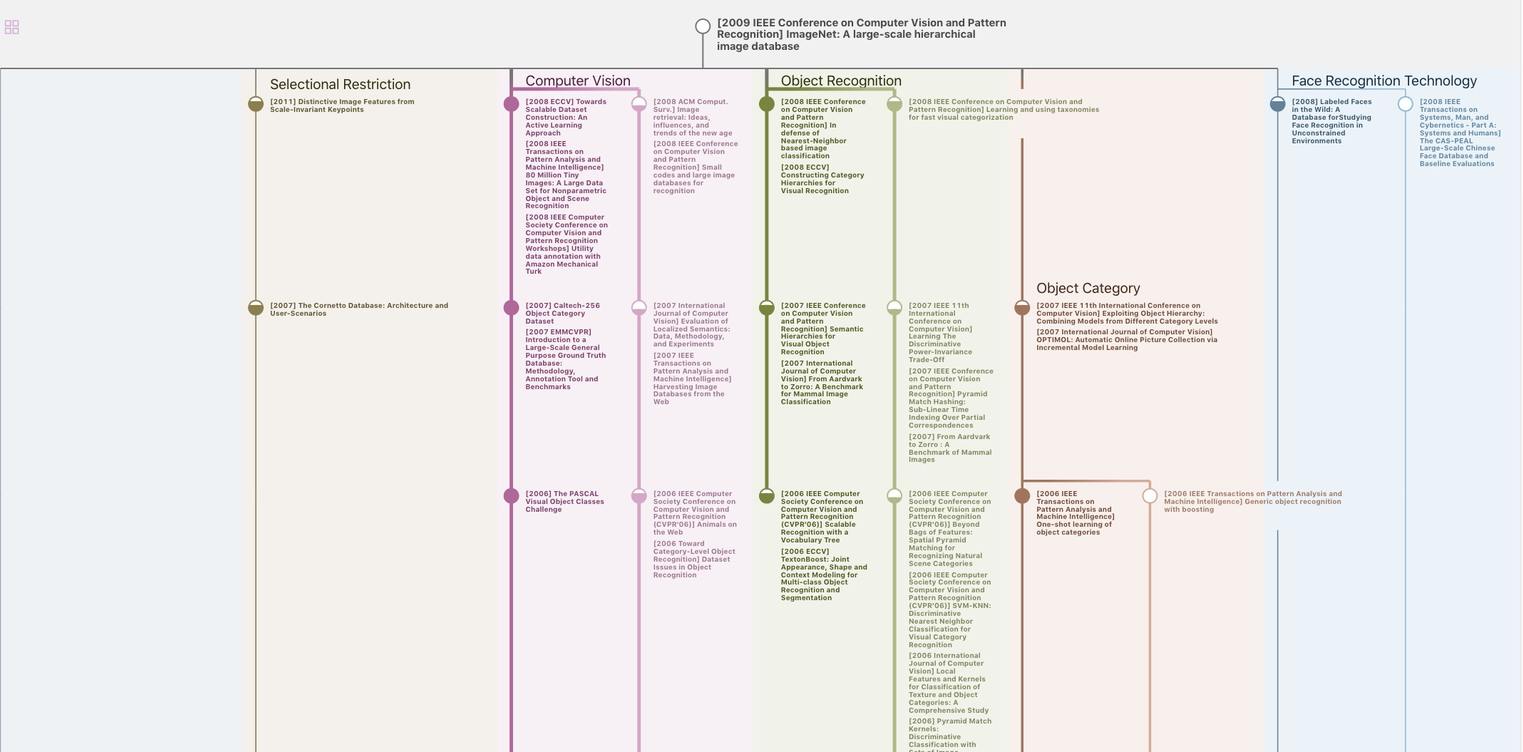
生成溯源树,研究论文发展脉络
Chat Paper
正在生成论文摘要