Self-Supervised SpectralSpatial Graph Prototypical Network for Few-Shot Hyperspectral Image Classification
IEEE Trans. Geosci. Remote. Sens.(2023)
摘要
In recent years, deep learning has been widely applied to hyperspectral image (HSI) classification (HIC) with great success. However, since labeling hyperspectral images (HSIs) is time-consuming and labor-intensive, a limited number of labeled HSIs are available, making it difficult to train feature extractors and classifiers. To address this challenge, this article introduces a self-supervised spectral-spatial graph prototypical network (S4GPN) for few-shot HIC. In addition, we combine self-supervised learning (SSL) with few-shot learning (FSL) to provide additional semantic information to improve classification accuracy. Our method consists of three stages, including the prototypical network (PN) stage, the SSL stage, and the fusion stage, with each stage progressively improving the classification performance. In the PN stage, we perform supervised learning using a PN structure and leverage the supervised information to guide SSL. In the SSL stage, we use the SimSiam structure in a novel way for model training after the augmentation of spectral and spatial data separately. Finally, the features learned in the first two stages are fused to improve the quality of the feature representation. These three stages use the same structured feature extractors. To extract more diverse and discriminative feature representations for the HIC task, our method uses the graph convolution network (GCN) and the dense network (Densenet) to extract spectral information and spatial information, respectively. Experiments on four datasets and comparisons with the state-of-the-art methods demonstrate that our proposed S4GPN outperforms other methods for HIC.
更多查看译文
关键词
Few-shot learning (FSL), hyperspectral image (HSI) classification (HIC), prototypical network (PN), self-supervised learning (SSL)
AI 理解论文
溯源树
样例
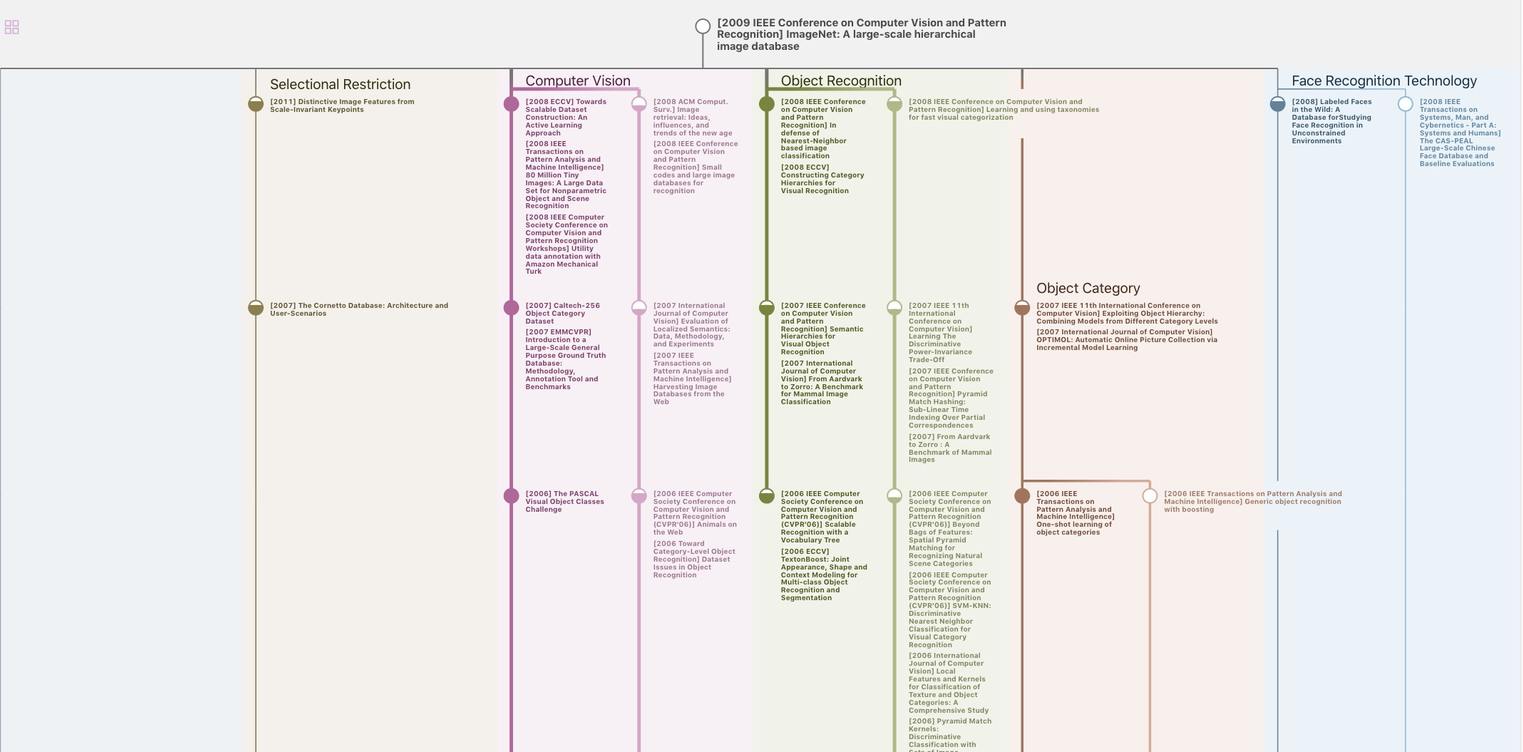
生成溯源树,研究论文发展脉络
Chat Paper
正在生成论文摘要