Underestimation-Aware Failure Statistical Model Creation for Large Scale Critical Systems
DSN-W(2023)
摘要
The two main dependability design quantitative assessment approaches are data-driven (black box) and model-driven (white box). Generally, the data-driven approach is more viable for complex systems because the model-driven approach is often computationally difficult. Meanwhile, the data-driven approach suffers from data modelling-related problems, such as model accuracy, data quality, and scalable data-processing needs. Owing to complexity, the data-driven approach is more viable for modern, dynamically composed, shared resource virtualized and containerized - distributed systems. However, the empirical models must deal with model accuracy risks. Estimation inaccuracy risks are often asymmetric for critical systems: overestimations "only" lead to unnecessary resource usage, but underestimations can lead to compromised extra-functional requirements. Rare failures with high severity are prime culprits of underestimation due to the difficulties of estimating long and heavy-tailed distributions over scarce data. Extreme value analysis is an available toolset for this problem; however, its known methods are not ready for the Big Data of modern distributed systems. Under some statistical restrictions, this paper proposes a novel Big Data capable extreme value analysis based computational model. At its core, the proposed method filters non-extreme values in an automated and scalable way to reduce subsequent data processing needs. We evaluated our approach on real-life container restart time data, but the potential applicability is far broader. In the case study, our method discarded 90 to 95% of the data without losing a single extreme value.
更多查看译文
关键词
automated threshold selection,big data,critical systems,extreme value analysis,peak over threshold method
AI 理解论文
溯源树
样例
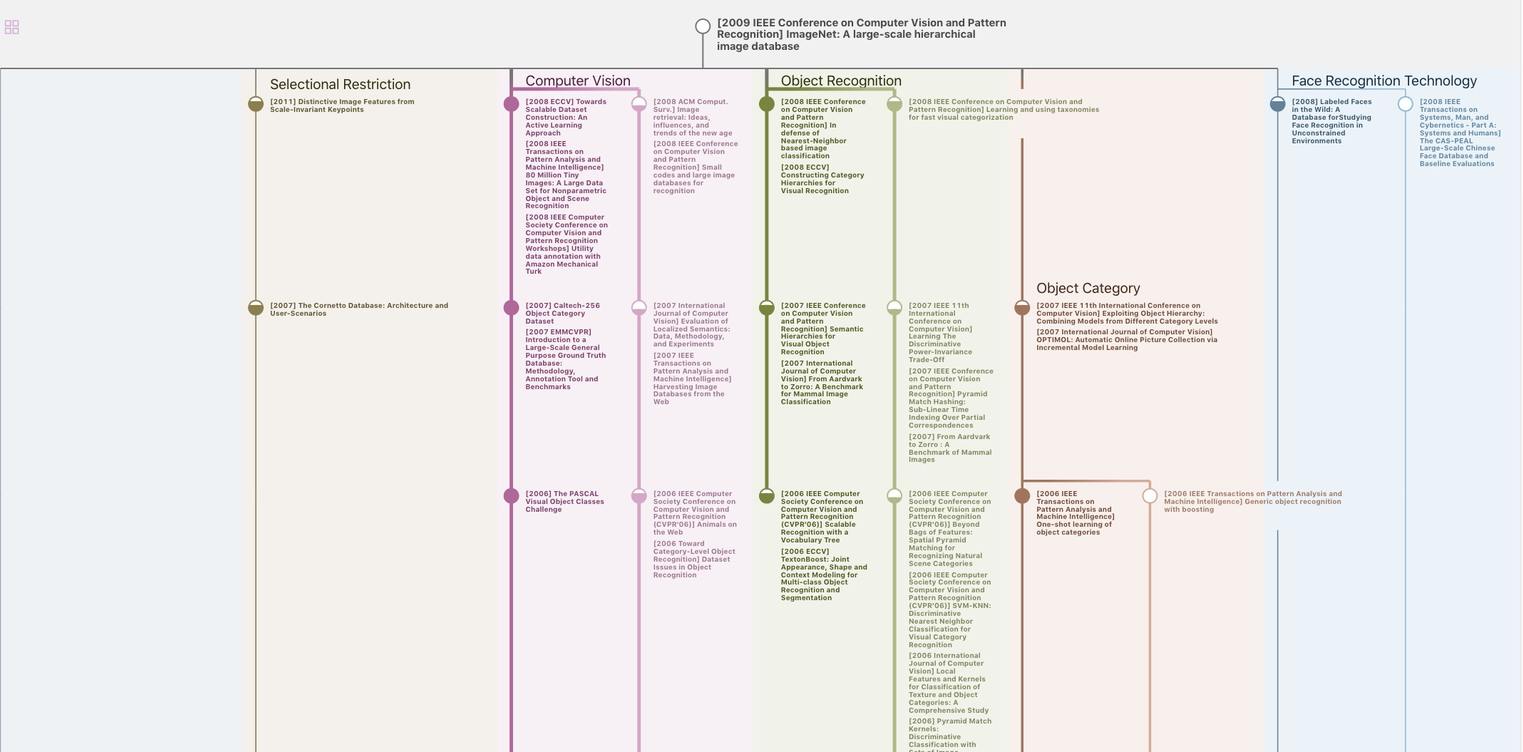
生成溯源树,研究论文发展脉络
Chat Paper
正在生成论文摘要