Drug-Target Interaction Prediction Based on Interpretable Graph Transformer Model.
ICIC (3)(2023)
摘要
This study proposes a novel architecture for drug-target interaction (DTI) prediction by leveraging protein binding sites and self-attention mechanisms. The architecture consists of four modules: Data Preparation, Graph Embedding Learning, Feature Extraction, and Prediction. Protein binding sites are extracted from the 3D structure of proteins using a simulation-based model in the Data Preparation module to simplify model complexity. A map of protein pockets and ligands is then generated and utilized to learn embeddings using Topology Adaptive Graph Convolutional Networks to extract global and local features of the protein pocket and ligand. The protein pocket and ligand signature are fused via the Self-attentive Bidirectional Long Short-Term Memory block to obtain a representation of the drug-target complex. The resulting cascaded representation is then fed into a binary classifier for predicting DTI. By employing the self-attention mechanism in the network, the attention output is computed using cascading embeddings of drug-target pairs as inputs, enabling interpretability by identifying the protein regions that interact with ligands in a given drug-target pair. The experimental results demonstrate the superiority of the proposed architecture over existing DTI predictive models.
更多查看译文
关键词
graph,interaction,prediction,drug-target
AI 理解论文
溯源树
样例
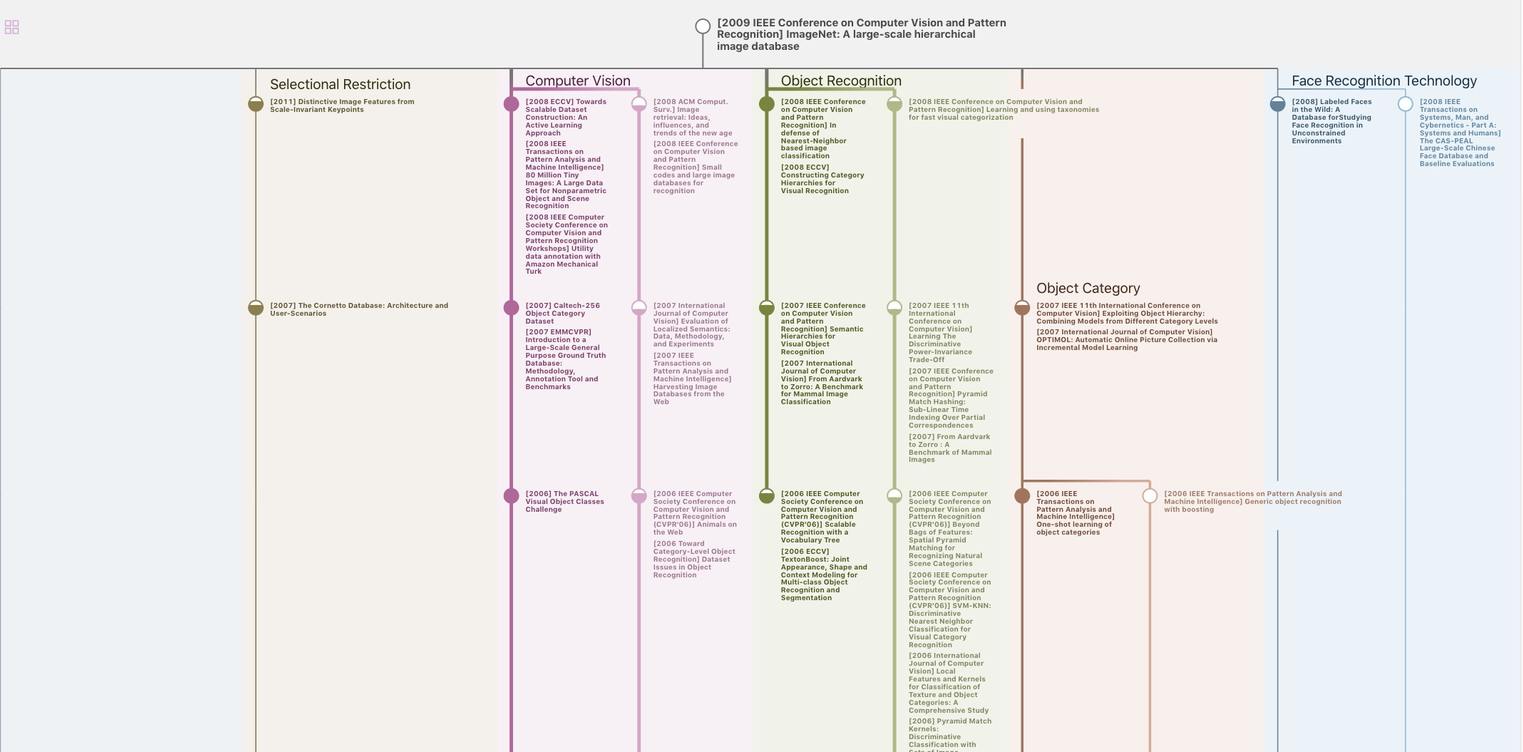
生成溯源树,研究论文发展脉络
Chat Paper
正在生成论文摘要