A Deep Learning Approach Incorporating Data Missing Mechanism in Predicting Acute Kidney Injury in ICU.
ICIC (3)(2023)
摘要
Acute Kidney Injury (AKI) is common in the intensive care units (ICUs) and is associated with an increased risk of hospital mortality and additional healthcare-related costs. The prediction of AKI can help clinicians to identify patients’ risk of deterioration and intervene in the early stage of disease progression to prevent further damage. The development of EHR systems and the capabilities of data mining and machine learning methods have inspired us to explore novel tools for analyzing disease trajectories and provide us an opportunity for identifying early AKI onset more accurately. In this study, we applied the Attention LSTM model (ALSTM) and LightGBM models for AKI prediction at 4 prediction windows, where data imputation approaches were evaluated accordingly. The ALSTM models with Gaussian Process (GP) imputation showed higher performance in longer prediction windows, achieving AUC of 0.901, 0.890 and 0.877, and accuracy of 0.818, 0.806 and 0.797 in the 6-, 12- and 24-h model. While in the 2-h prediction model, LightGBM with linear imputation achieved the best AUC of 0.908. The deep learning models showed high potential in identifying patients at high risk of AKI earlier, which could provide information to guide personalized treatment. Early prediction of AKI could provide clinicians with decision support for early intervention and focus on the identified risk factors.
更多查看译文
关键词
predicting acute kidney injury,deep learning,icu
AI 理解论文
溯源树
样例
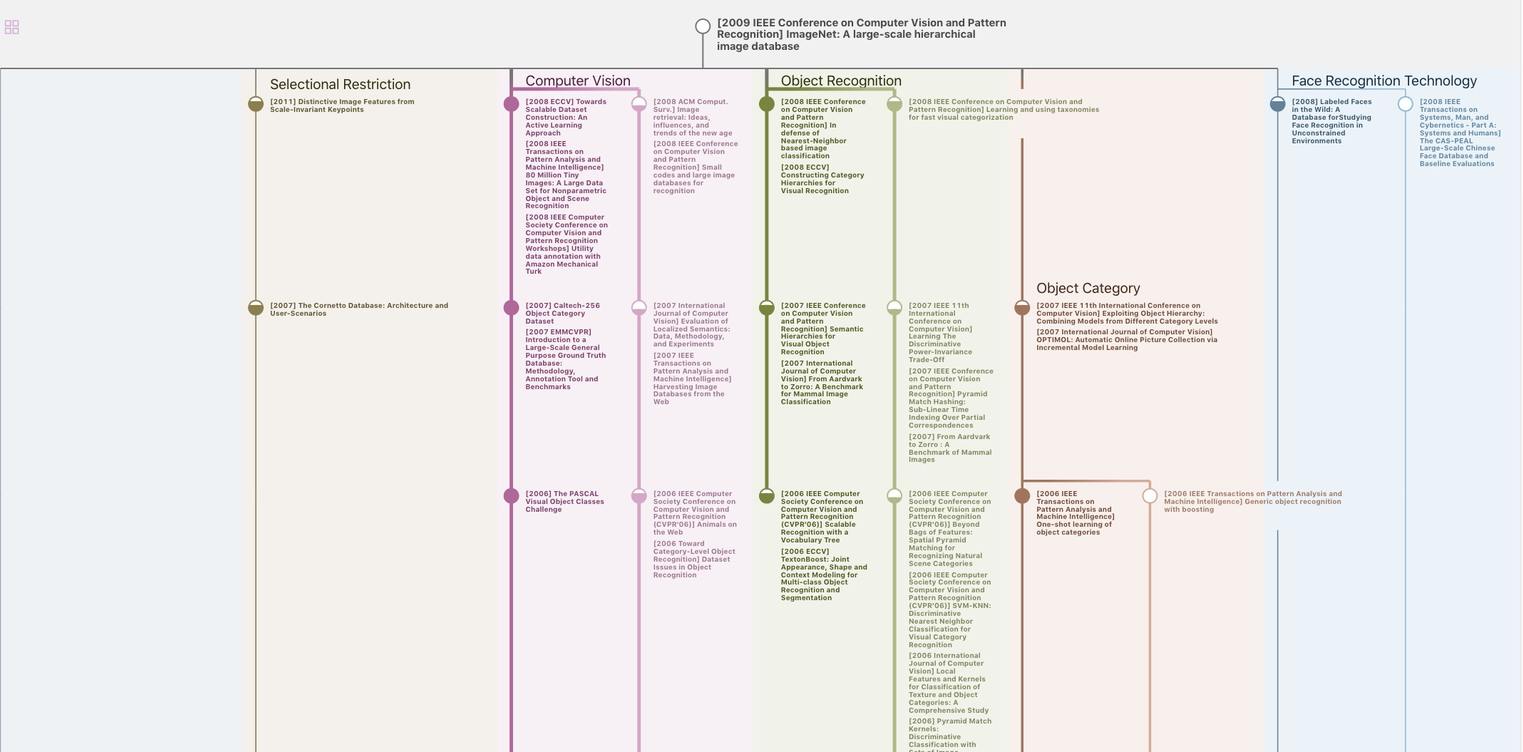
生成溯源树,研究论文发展脉络
Chat Paper
正在生成论文摘要