A Novel Graph Representation Learning Model for Drug Repositioning Using Graph Transition Probability Matrix Over Heterogenous Information Networks.
ICIC (3)(2023)
摘要
Computational drug repositioning is a promising strategy in discovering new indicators for approved or experimental drugs. However, most of computational-based methods fall short of taking into account the non-Euclidean nature of biomedical network data. To address this challenge, we propose a graph representation learning model, called DDAGTP, for drug repositioning using graph transition probability matrix in heterogenous information networks (HINs), In particular, DDAGTP first integrates three different types of drug-disease, drug-protein and protein-disease association networks and their biological knowledge to construct a heterogeneous information network (HIN). Then, a graph convolution autoencoder model is adopted by combining graph transfer probabilities to learn the feature representation of drugs and diseases. Finally, DDAGTP incorporates a CatBoost classifier to complete the task of drug-disease association prediction. Experimental results demonstrate that DDAGTP achieves the excellent performance on all benchmark datasets when compared with state-of-the-art prediction models in terms of several evaluation metrics.
更多查看译文
关键词
graph representation,graph transition probability matrix,drug repositioning
AI 理解论文
溯源树
样例
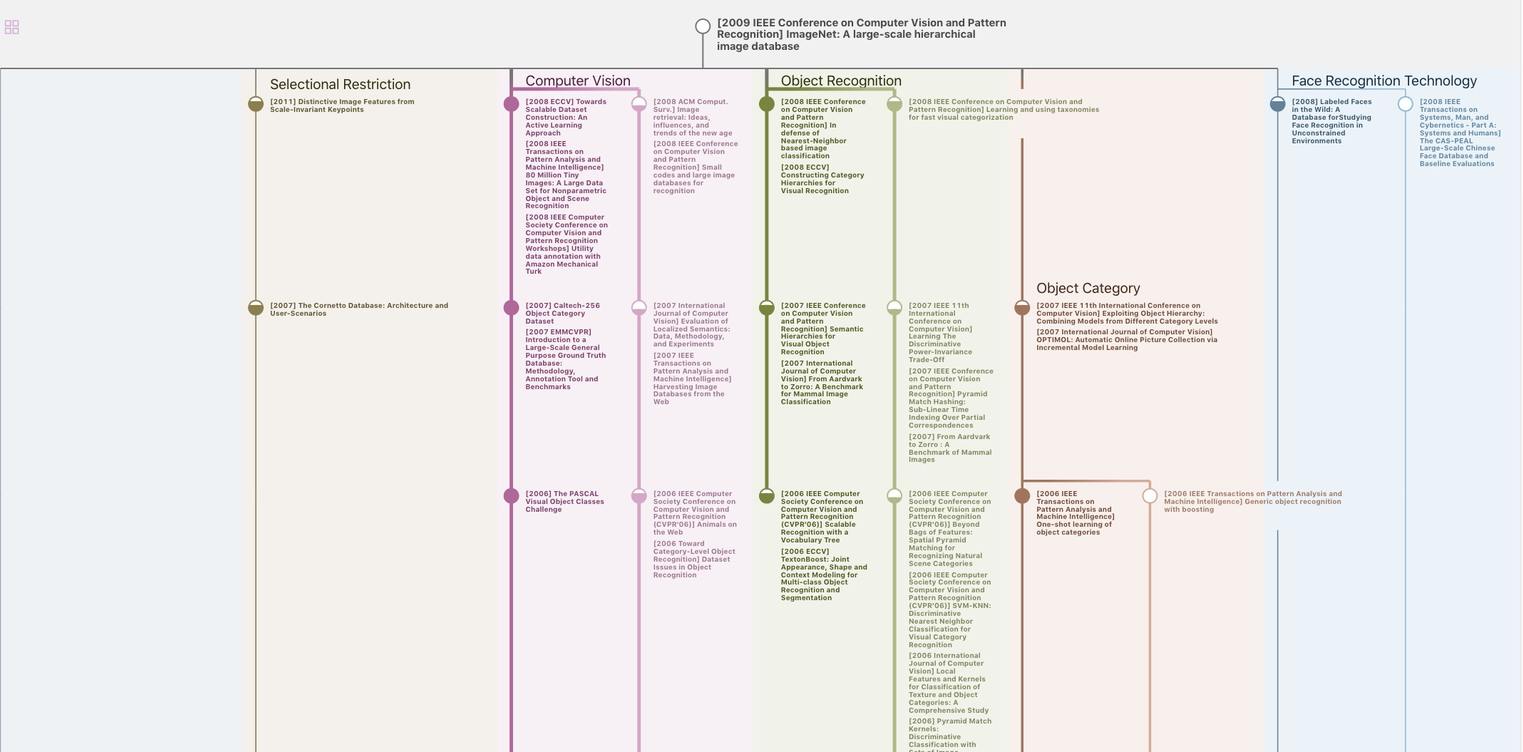
生成溯源树,研究论文发展脉络
Chat Paper
正在生成论文摘要