GeneSpider: Inferring Gene Regulation Relationships Through Graph Neural Network from Single-Cell RNA Sequence Data.
ICIC (3)(2023)
摘要
Predicting gene regulation relationships from single-cell expression data is an important problem in the field of genomics. Numerous methods have been developed to address the challenges of high dimensionality and sparsity in single-cell expression data, including machine learning and deep learning techniques. In this paper, we present a novel framework called GeneSpider for inferring gene regulation networks from single-cell RNA sequence expression data. We use Germain Angular Summation Field to encode single-cell RNA sequence expression data to image representation, which reduces the impact of sparseness and high dimensionality of single-cell RNA sequence expression data on the reconstruction of gene regulation networks. Then, we propose a novel graph neural network consisted of two convolution layers which extracts features of image nodes and topological information of their neighborhoods. It can sum the features of the extracted nodes and their neighbors as nodes new feature. Finally, the probability of regulating between transcription factors and target genes outputted through fully connected layers. We compare GeneSpider with eight state-of-the-art gene regulatory networks reconstruction methods on seven single-cell RNA sequence expression datasets with three ground-truth networks and show that GeneSpider achieves substantially better performance.
更多查看译文
关键词
inferring genespider regulation relationships,graph neural network,rna,neural network,single-cell
AI 理解论文
溯源树
样例
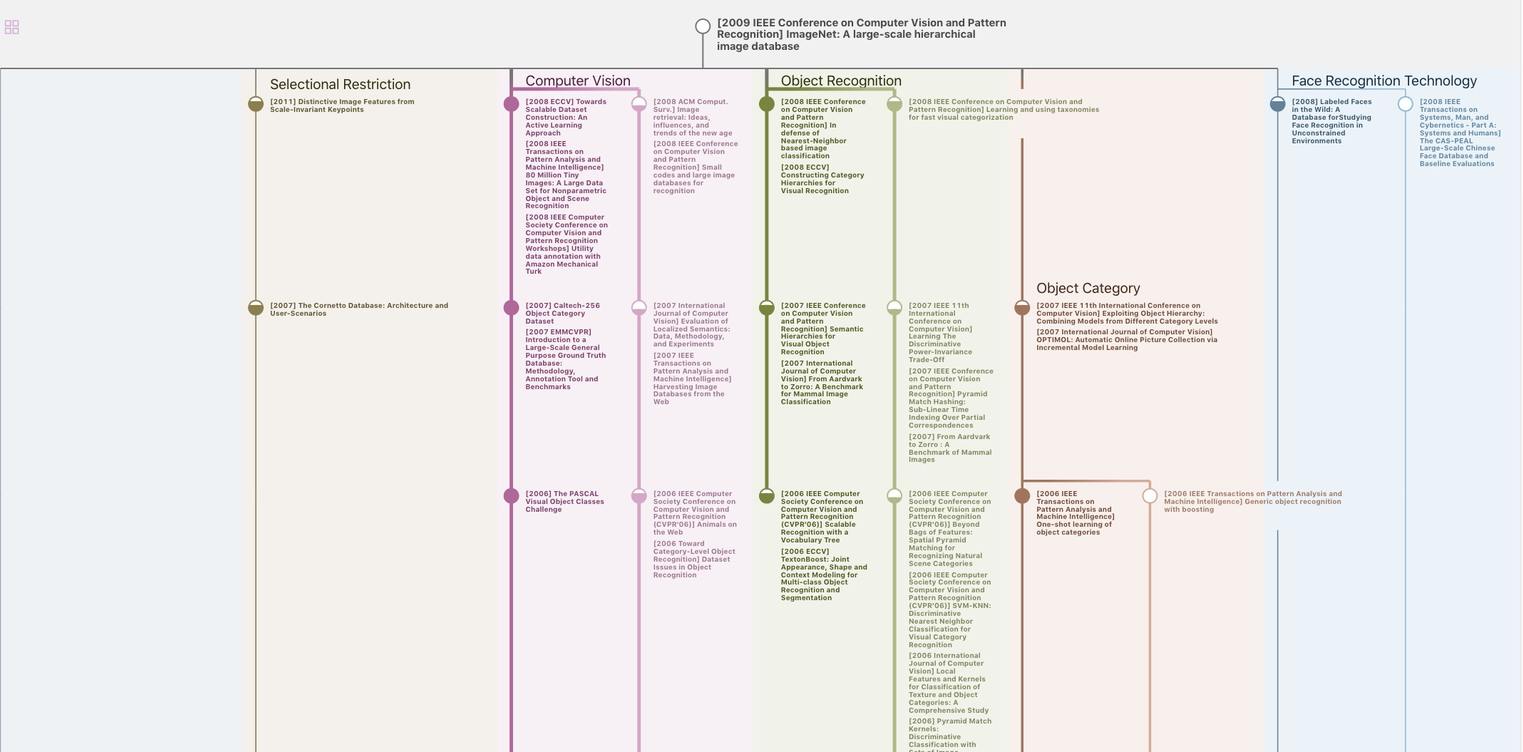
生成溯源树,研究论文发展脉络
Chat Paper
正在生成论文摘要