Cross Density Kernel for Nonstationary Signal Processing.
SSP(2023)
摘要
This paper introduces the cross density kernel function (CDKF), a new positive-definite kernel that quantifies the statistical dependence between random processes, to address the challenge of applying time series prediction and modeling techniques to nonstationary signals. The paper highlights the limited applicability of the Wiener filter and Parzen's autocorrelation reproducing kernel Hilbert spaces (RKHS) to stationary signals. CDKF extends these methods by capturing properties of probability density functions for random processes in the Hilbert space with a novel bidirectional recursion, and using two neural networks to optimize the kernel function based on realizations. The paper concludes by presenting experimental results that support the effectiveness of CDKF.
更多查看译文
关键词
nonstationary signals,kernels,statistical dependence
AI 理解论文
溯源树
样例
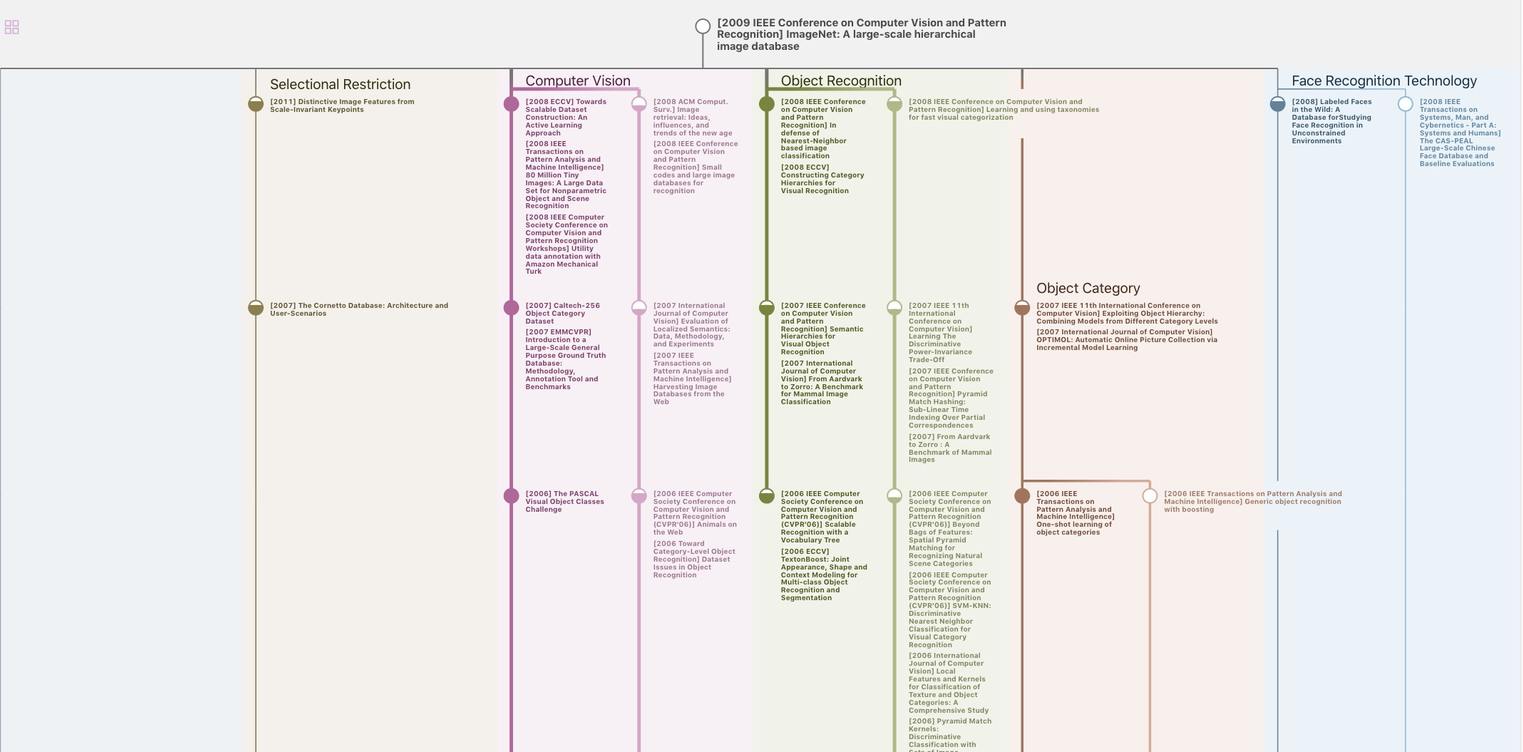
生成溯源树,研究论文发展脉络
Chat Paper
正在生成论文摘要