Multi-scale 2.5D Integrated Model for False Positive Reduction in Abdominal Lymph Node Detection.
CNIOT(2023)
摘要
Accurate lymph node detection based on CT images is an important pre-step in Lymphadenectomy, which is crucial for the subsequent treatment of patients. However, many interfering factors such as organs, tissues, and blood vessels in CT images lead a large number of false positive objects with the existing detection methods, which hinders the clinical application. In this study, we propose an effective and fast 2.5D framework algorithm for false positive reduction (FPR). Specifically, considering the globular shape of the lymph nodes, the axial, coronal and sagittal slices from CT data of lymph nodes are used as 2.5D inputs to the network to mine the three-dimensional spatial information of lymph nodes. In addition, multi-scale inputs are used to address the challenges posed by changes in the volume of the lymph nodes, and use stacking learning to fuse the results of models at various scales. Compared to 3D networks, it greatly decreases computational costs and running time. Based on the public dataset, we add 20 additional cases of CT data to construct a new dataset CTLymph for FPR. The proposed method achieves an AUC of 0.941, reducing the number of false positive lymph nodes from 25 to 4. The result shows that our proposed model achieves superior performance and outperforms several state-of-the-art methods.
更多查看译文
AI 理解论文
溯源树
样例
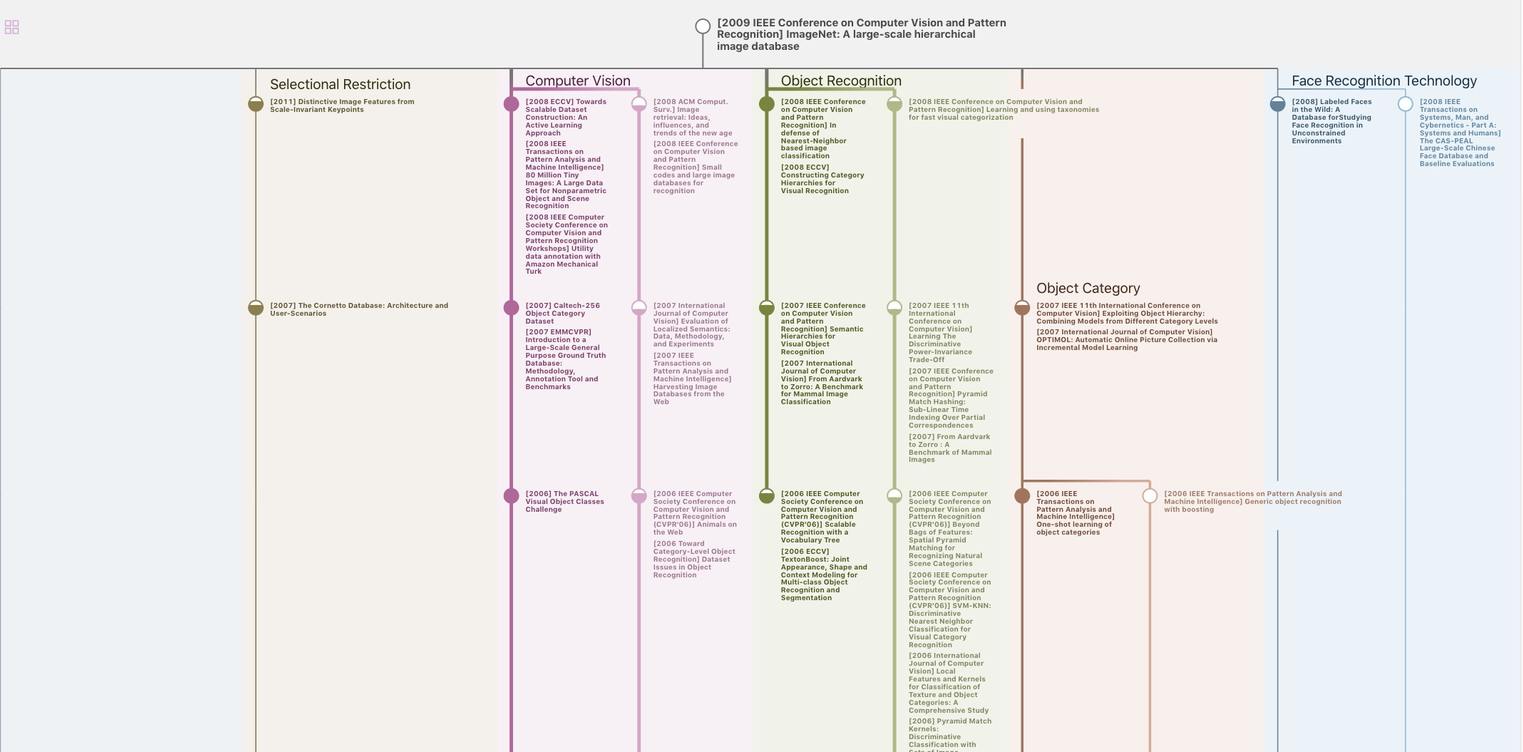
生成溯源树,研究论文发展脉络
Chat Paper
正在生成论文摘要