FedGM: Heterogeneous Federated Learning via Generative Learning and Mutual Distillation.
Euro-Par(2023)
摘要
Federated learning is a distributed machine learning that enables models to aggregate on the server after local training to protect privacy. However, user heterogeneity presents a challenge in federated learning. To address this issue, some recent work has proposed using knowledge distillation. But the application of knowledge distillation in federated learning is dependent on the proxy dataset, which can be difficult to obtain in practice. Additionally, the simple average aggregation method of model parameters may fail to achieve a global model with good generalization performance, and may also lead to potential privacy breaches. To tackle these issues, we propose FedGM, a data-free federated knowledge distillation method that combines generative learning with mutual distillation. FedGM addresses user heterogeneity while also protecting user privacy. We use a conditional generator to extract global knowledge to guide local model training and build a proxy dataset on the server-side to perform mutual distillation. Extensive experiments on benchmark datasets show that FedGM outperforms state-of-the-art approaches in terms of generalization performance and privacy protection.
更多查看译文
关键词
heterogeneous federated learning,generative learning,mutual distillation
AI 理解论文
溯源树
样例
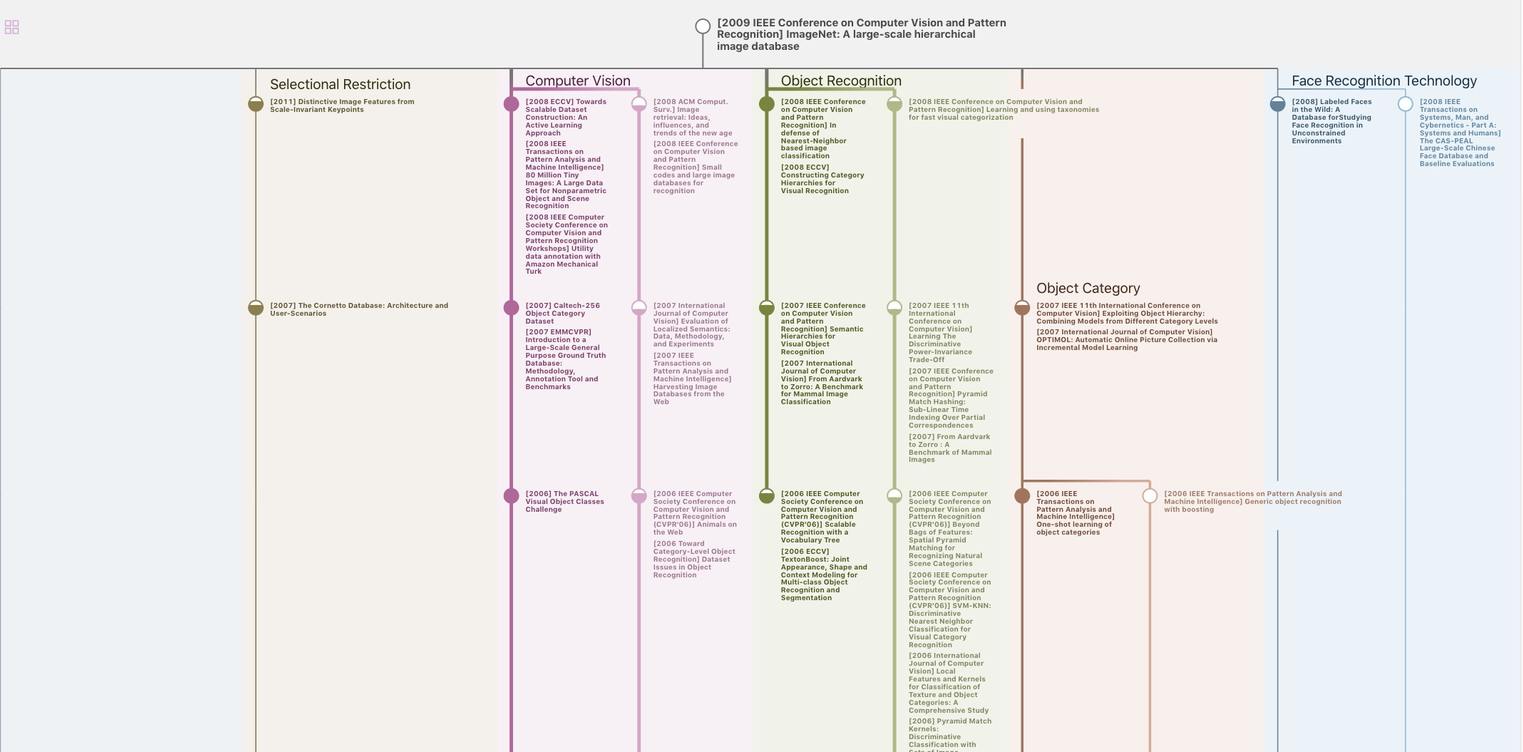
生成溯源树,研究论文发展脉络
Chat Paper
正在生成论文摘要