On Learning Data-Driven Models For In-Flight Drone Battery Discharge Estimation From Real Data.
SMARTCOMP(2023)
摘要
Accurate estimation of the battery state of charge (SOC) for unmanned aerial vehicles (UAV) in-flight monitoring is essential for the safety and survivability of the system. Successful physics-based models of the battery have been developed in the past, however, these models do not take into account the effects of mission profile and environmental conditions during flight on the battery power consumption. Recently, data-driven methods have become popular given their ease of use and scalability. Yet, most benchmarking experiments have been conducted on simulated battery datasets. In this work, we compare different data-driven models for battery SOC estimation of a hexacopter UAV system using real flight data. We analyze the importance of a number of flight variables under different environmental conditions to determine the factors that affect battery SOC over the course of the flight. Our experiments demonstrate that additional flight variables are necessary to create an accurate SOC estimation model through data-driven methods.
更多查看译文
关键词
unmanned aerial vehicles, battery discharge estimation, feature importance, data-driven models
AI 理解论文
溯源树
样例
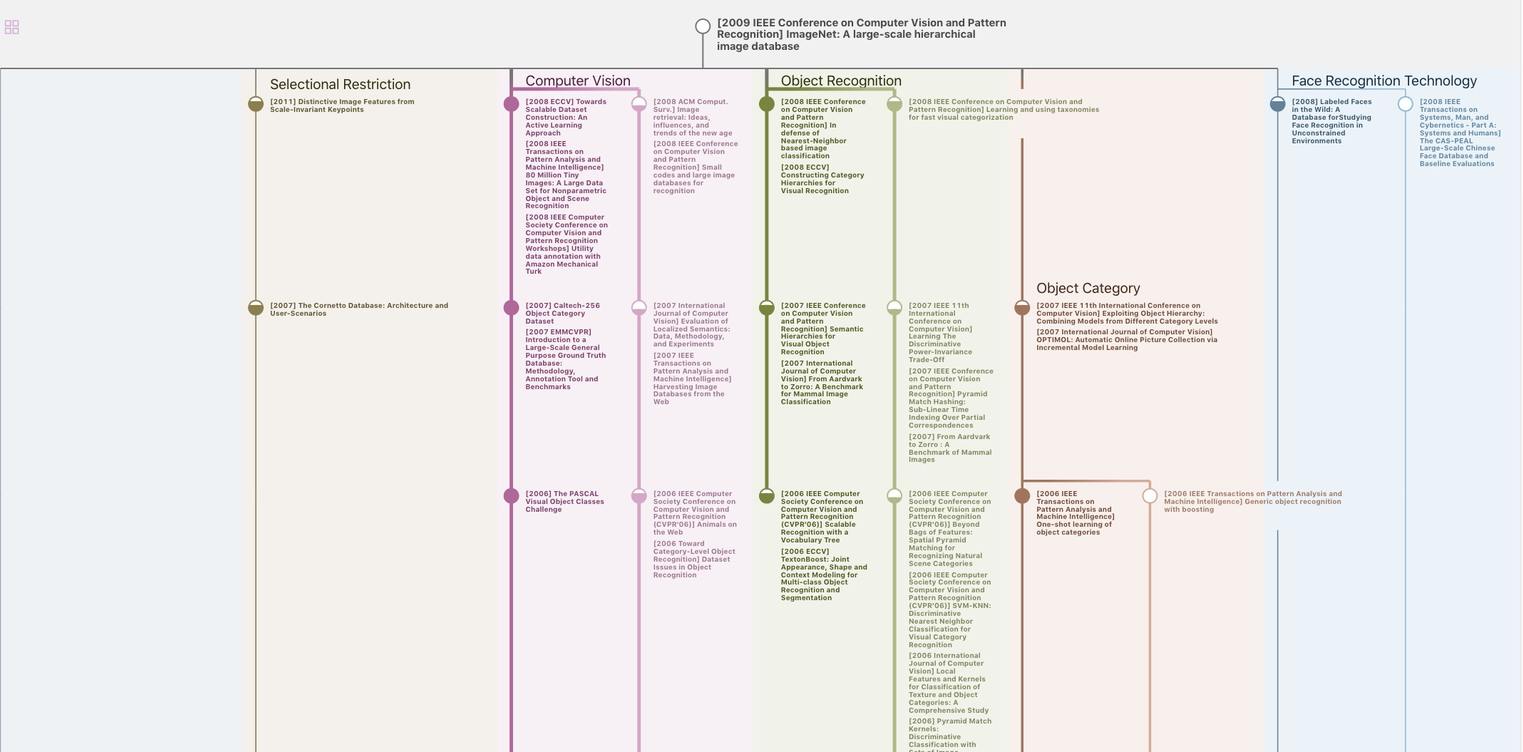
生成溯源树,研究论文发展脉络
Chat Paper
正在生成论文摘要