Addressing Domain Shift in Pedestrian Detection from Thermal Cameras without Fine-Tuning or Transfer Learning
SMARTCOMP(2023)
摘要
The use of thermal imaging to detect the presence of people in indoor and outdoor environments is gaining an increasing attention given its wide applicability in the tourism, security, and mobility domains. However, due to the particular characteristics of different contexts, it is necessary to train/finetuning specifically object detectors for each scenario in order to obtain accurate results. This is due to changes in appearance caused by camera position, scene size, environmental factors, etc. In this paper, we present a data augmentation method that can improve both versatility and robustness of pedestrian detection models based on thermal images. Thanks to our solution, the trained model can deal with unseen thermal data from both indoor and outdoor environments, reliably detecting pedestrians regardless of their apparent size and position in the image, without any fine-tuning or transfer learning, therefore avoiding time consuming labeling activities to fine-tune and deploy the system in different scenarios.
更多查看译文
关键词
YOLOV5,thermal imaging,data augmentation,pedestrian detection
AI 理解论文
溯源树
样例
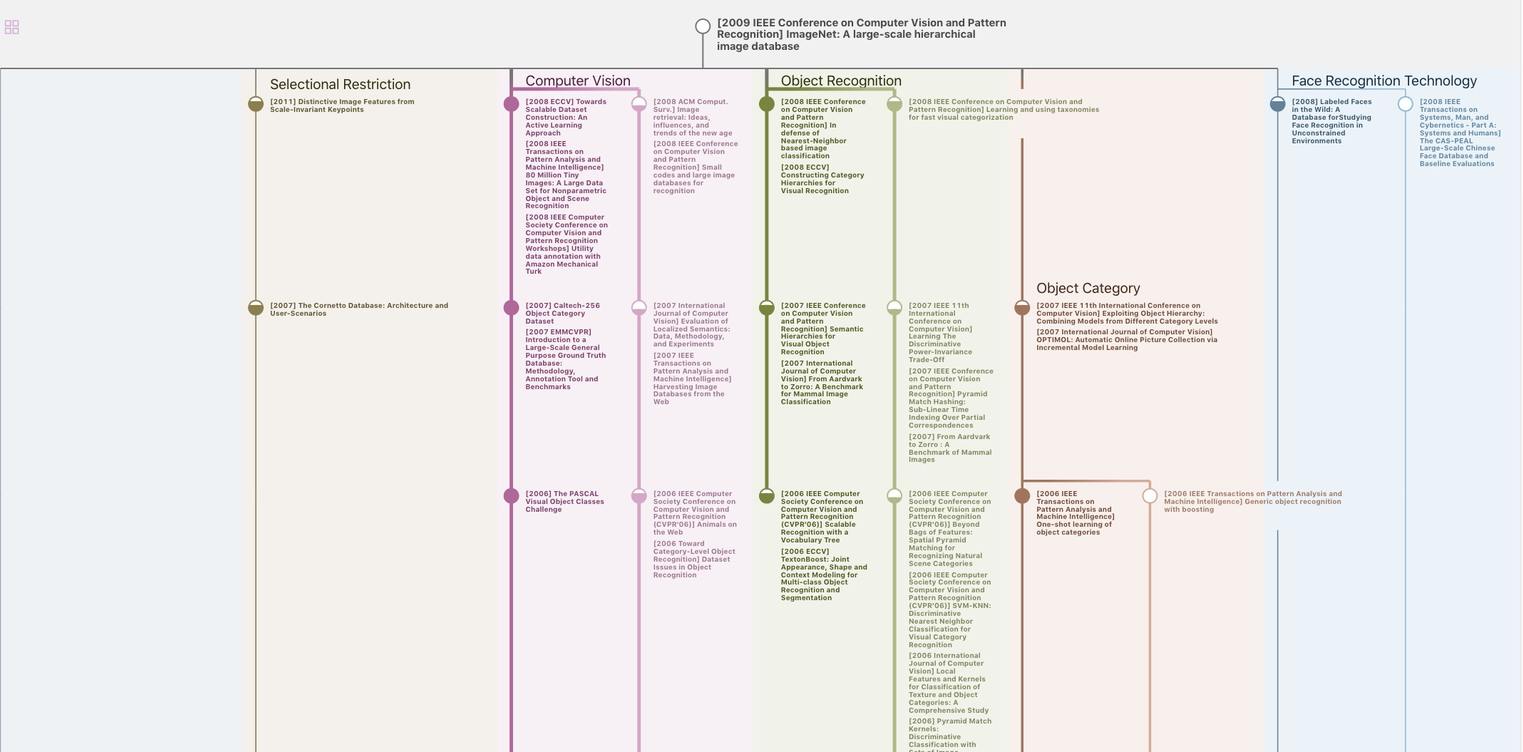
生成溯源树,研究论文发展脉络
Chat Paper
正在生成论文摘要