Implications of Solution Patterns on Adversarial Robustness.
CVPR Workshops(2023)
摘要
Empirical robustness evaluation (RE) of deep learning models against adversarial perturbations involves solving non-trivial constrained optimization problems. Recent work has shown that these RE problems can be reliably solved by a general-purpose constrained-optimization solver, PyGRANSO with Constraint-Folding (PWCF). In this paper, we take advantage of PWCF and other existing numerical RE algorithms to explore distinct solution patterns in solving RE problems with various combinations of losses, perturbation models, and optimization algorithms. We then provide extensive discussions on the implications of these patterns on current robustness evaluation and adversarial training. A comprehensive version of this work can be found in [19].
更多查看译文
关键词
adversarial attacker,adversarial perturbations,adversarial robustness,adversarial training,deep learning,general-purpose constrained-optimization solver,nontrivial constrained optimization problems,perturbation models,PWCF,PyGRANSO with constraint-folding,RE,robustness evaluation,solution patterns
AI 理解论文
溯源树
样例
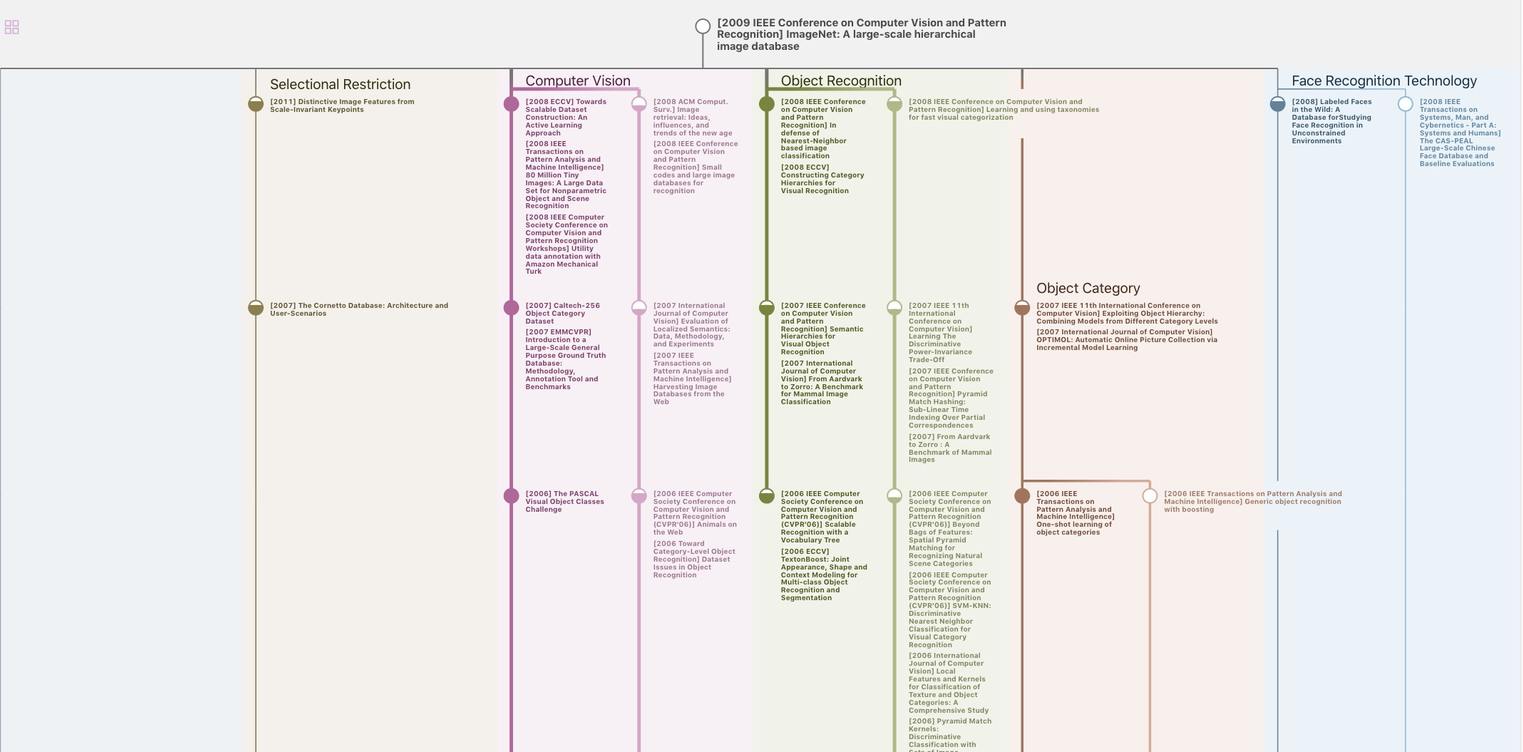
生成溯源树,研究论文发展脉络
Chat Paper
正在生成论文摘要