DeSRF: Deformable Stylized Radiance Field
CVPR Workshops(2023)
摘要
When stylizing 3D scenes, current methods need to render the full-resolution images from different views and use the style loss, which is proposed for 2D style transfer and needs to be calculated on the whole image, to optimize the stylized radiance fields. It is quite inefficient when we need to stylize a large-scale scene. This paper proposes a more efficient method, DeSRF, to stylize the radiance fields, which also transfers style information to the geometry according to the input style. To achieve this goal, on the one hand, we first introduce a deformable module, which can learn the geometric style contained in the input style image and transfer it to radiance fields. On the other hand, although the style loss needs to be calculated for the entire image, actually we do not need to process all the rays when updating the stylized radiance fields. Motivated by this observation, we propose a new training strategy called Dilated Sampling (DS) for efficient stylization propagation. Experimental results show that our method works more efficiently and produces more visually-reasonable stylized 3D scenes with geometry style information compared to other existing approaches.
更多查看译文
AI 理解论文
溯源树
样例
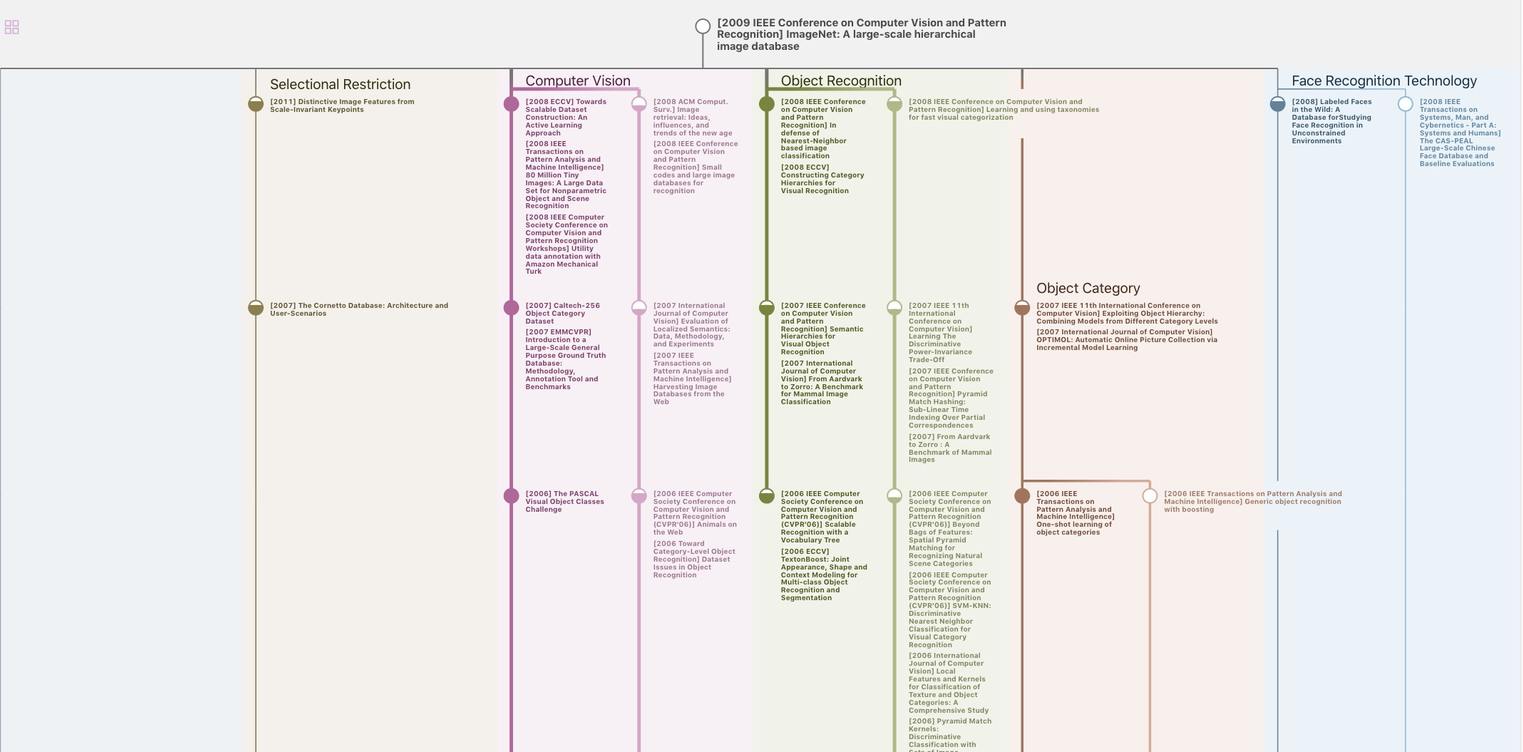
生成溯源树,研究论文发展脉络
Chat Paper
正在生成论文摘要