Many-Task Federated Learning: A New Problem Setting and A Simple Baseline.
CVPR Workshops(2023)
摘要
Federated Learning (FL) was originally proposed to effectively exploit more data that are distributed at local clients even though the local data follow non-i.i.d. distributions. The fundamental intuition is that, the more data we can use the better model we are likely to obtain in spite of the increased difficulty of learning due to the non-i.i.d. data distribution, or data heterogeneity. With this intuition, we strive to further scale up FL to cover more clients to participate and increase the effective coverage of more user data, by enabling FL to handle collaboration between clients that perform different yet related task types, i.e., enabling a new level of heterogeneity: task heterogeneity, which can be entangled with data heterogeneity and lead to more intractable clients. However, solving such compound heterogeneities from both data and task levels raises major challenges, against the current global, static, and identical federated aggregation ways across clients. To tackle this new and challenging FL setting, we propose an intuitive clustering-based training baseline to tackle the significant data and task heterogeneities. Specifically, each agent dynamically infers its "proximity" with others by comparing their layer-wise weight updates sent to the server, and then flexibly determines how to aggregate weights with selected similar clients. We construct new testbeds to examine our novel problem setting and algorithm on two benchmark datasets in multi-task learning: NYU Depth and PASCAL-Context datasets. Extensive experiments demonstrate that our proposed method shows superiority over plain FL algorithms such as FedAvg and FedProx in the 5-task setting on Pascal-Context and even enables jointly federated learning over the combined set of PASCAL-Context and NYU Depth (9 tasks, 2 data domains). Codes are available at: https://github.com/VITA-Group/MaT-FL.
更多查看译文
关键词
5-task setting,compound heterogeneities,data distribution,data heterogeneity,FedAvg,FedProx,FL algorithms,FL setting,intractable clients,intuitive clustering-based training baseline,local clients,many-task federated learning,multitask learning,noni.i.d. data distribution,NYU Depth dataset,PASCAL-Context dataset,significant data,task heterogeneities,task heterogeneity
AI 理解论文
溯源树
样例
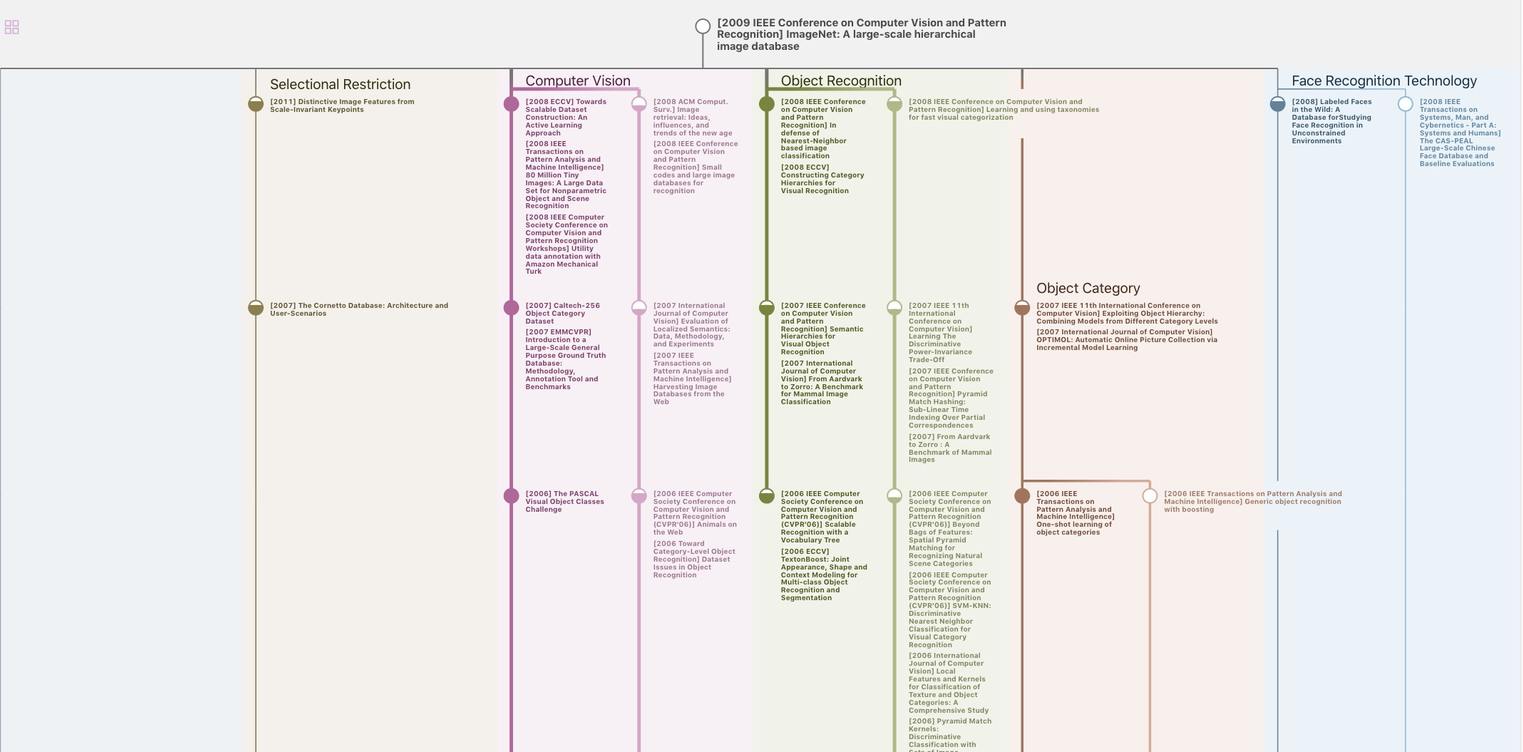
生成溯源树,研究论文发展脉络
Chat Paper
正在生成论文摘要