A Novel Tensor Tracking Algorithm for Block-Term Decomposition of Streaming Tensors.
SSP(2023)
摘要
Block-term decomposition (BTD), which factorizes a tensor (aka a multiway array) into block components of low rank, has been a powerful processing tool for multivariate and high-dimensional data analysis. In this paper, we propose a novel tensor tracking method called SBTD for factorizing tensors derived from multidimensional data streams under the BTD format. Thanks to the alternating optimization framework, SBTD first applies a regularized least-squares solver to estimate the temporal factor of the underlying streaming tensor. Then, SBTD adopts an adaptive filter to track the non-temporal tensor factors over time by minimizing a weighted least-squares cost function. Numerical experiments indicate that SBTD is capable of tensor tracking with competitive performance compared to the state-of-the-art BTD algorithms.
更多查看译文
关键词
Tensor tracking, block-term decomposition, adaptive algorithms, data streams
AI 理解论文
溯源树
样例
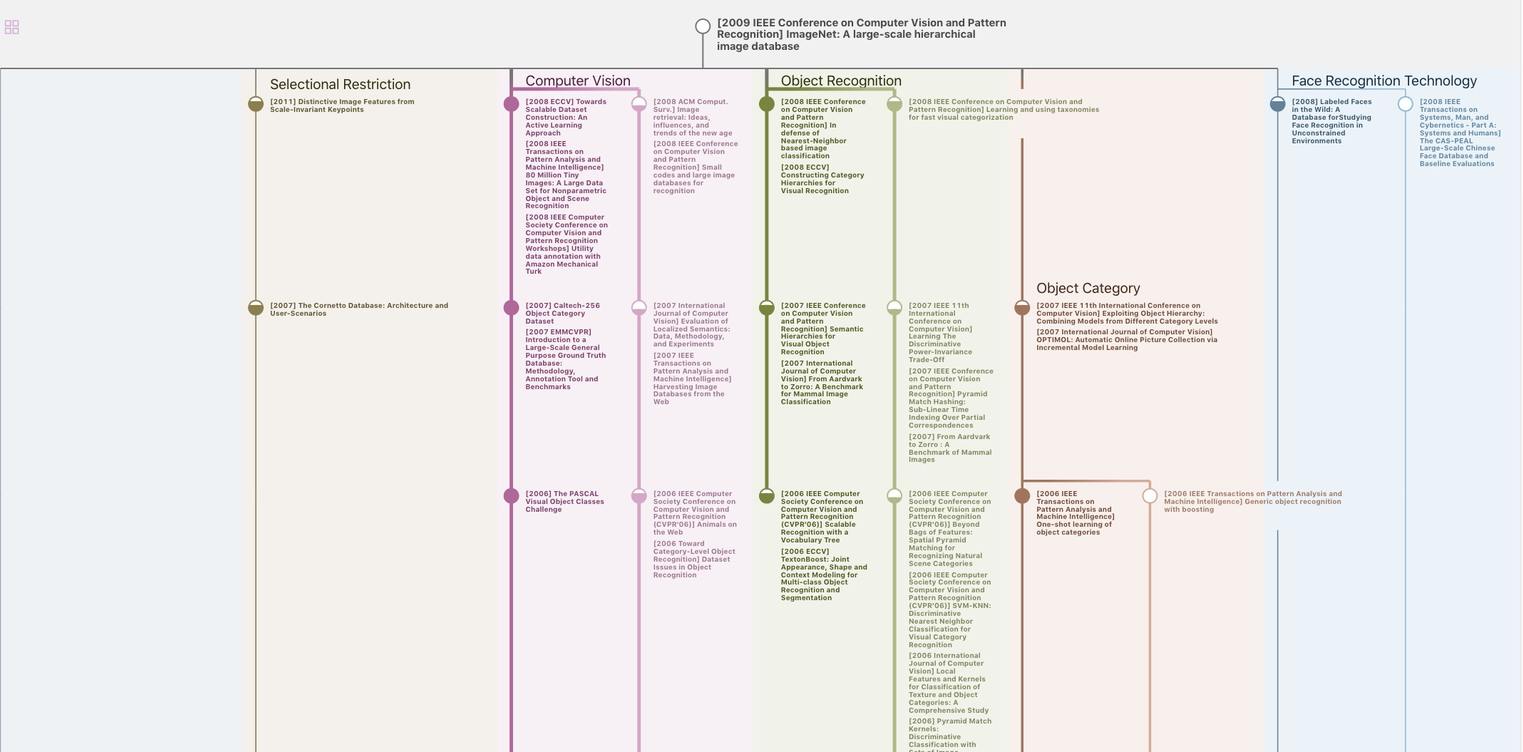
生成溯源树,研究论文发展脉络
Chat Paper
正在生成论文摘要