Intelligent Spectrum Sensing with ConvNet for 5G and LTE Signals Identification.
SSP(2023)
摘要
The paper presents an intelligent spectrum sensing approach for next-generation wireless networks by exploiting deep learning, in which we develop a deep convolutional network (ConvNet) to automatically identify Fifth Generation New Radio (5G NR) and Long-Term Evolution (LTE) signals under standards-specified channel models with diversified RF impairments. In particular, we design a semantic segmentation ConvNet to detect and localize the spectral content of 5G NR and LTE in a synthetic signal featured by spectrum occupancy. A received signal is first converted by a short-time Fourier transform and represented as a wideband spectrogram image which is then passed through the ConvNet, incorporated by DeepLabv3+ and ResNet18 to improve the accuracy of pixel-wise segmentation to further increase the accuracy of signal identification. In the simulations, our ConvNet achieves around 95% mean accuracy and 91% mean intersection-over-union (IoU) at medium SNR level and demonstrates robustness under various practical channel impairments.
更多查看译文
关键词
5G NR, cognitive ration, deep learning, encoder-decoder architecture, signal identification, spectrum sensing
AI 理解论文
溯源树
样例
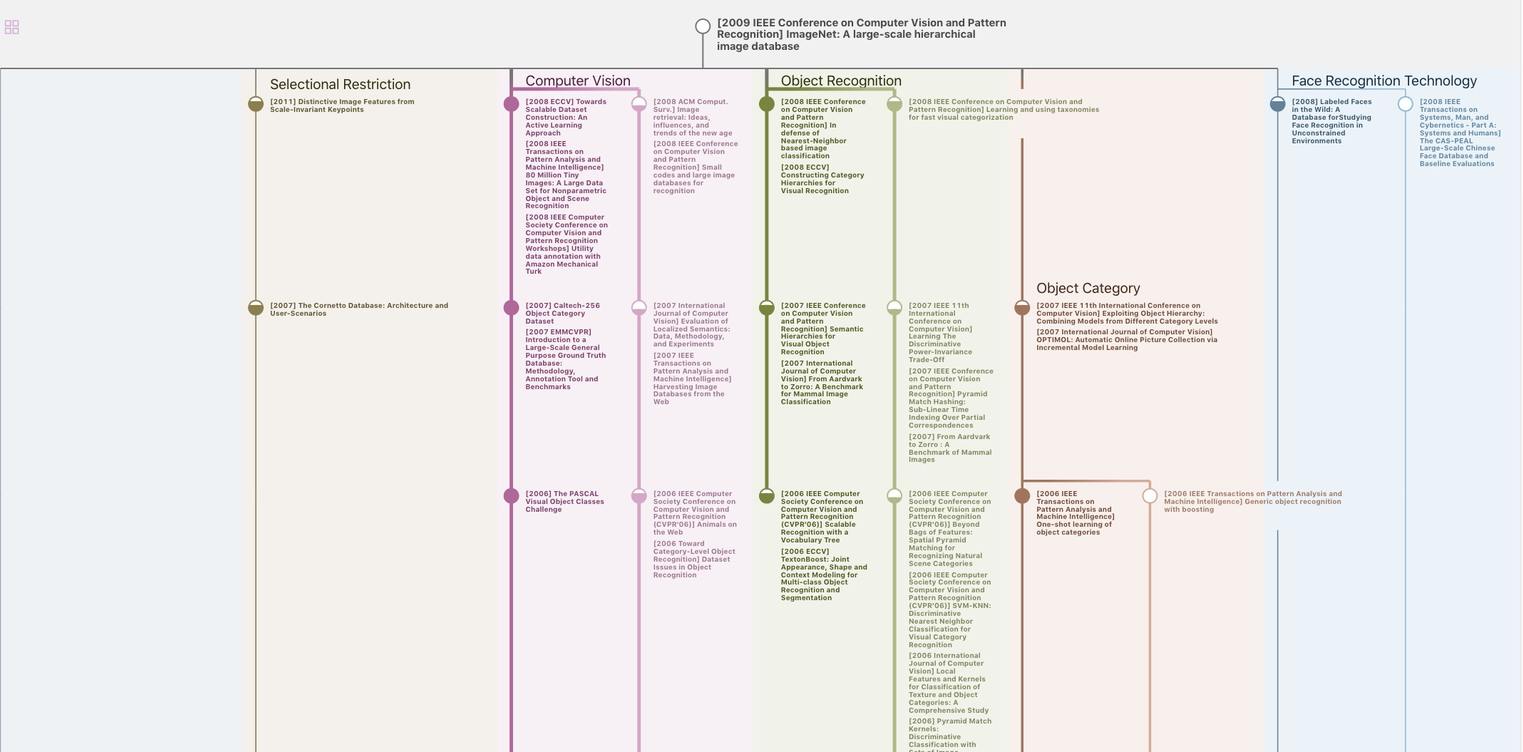
生成溯源树,研究论文发展脉络
Chat Paper
正在生成论文摘要