Robust and Scalable Vehicle Re-Identification via Self-Supervision.
CVPR Workshops(2023)
摘要
Many state-of-the-art solutions for vehicle re-identification (re-id) mostly focus on improving the accuracy on existing re-id benchmarks using additional annotated data. To balance the demands of accuracy, availability of annotated data, and computational efficiency, we propose a simple yet effective hybrid solution empowered by self-supervised learning which is free of intricate and computationally-demanding add-on attention modules often seen in state-of-the-art approaches. Through extensive experimentation, we show our approach, termed Self-Supervised and Boosted VEhicle Re-Identification (SSBVER), is on par with state-of-the-art alternatives in terms of accuracy without introducing any additional overhead during deployment. Additionally, we show that our approach, generalizes to different backbone architectures which accommodates various resource constraints and consistently results in a significant accuracy boost. Our code is available at https://github.com/Pirazh/SSBVER.
更多查看译文
关键词
annotated data,backbone architectures,re-id benchmarks,self-supervised and boosted vehicle re-identification,self-supervised learning,SSBVER
AI 理解论文
溯源树
样例
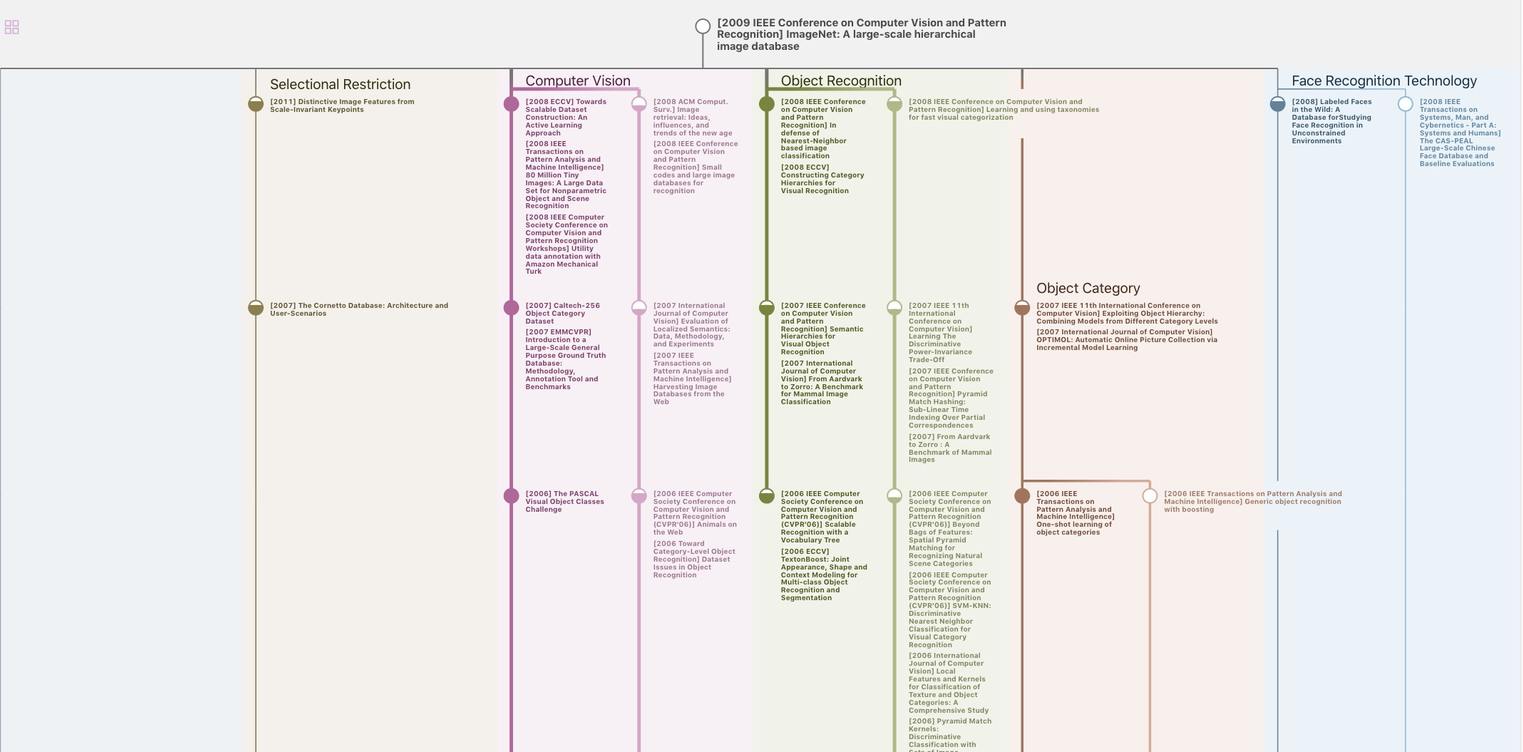
生成溯源树,研究论文发展脉络
Chat Paper
正在生成论文摘要