Hybrid Decision Making for Autonomous Driving in Complex Urban Scenarios
IV(2023)
摘要
Autonomous driving presents significant challenges due to the variability of behaviours exhibited by surrounding vehicles and the diversity of scenarios encountered. To address these challenges, we propose a hybrid architecture that combines traditional and deep learning techniques. Our architecture includes strategy, tactical and execution modules. Specifically, the strategy module defines the trajectory to be followed. Then, the tactical decision module employs a proximal policy optimization algorithm and deep reinforcement learning. Finally, the maneuver execution module uses a linear-quadratic regulator controller for trajectory tracking and a predictive model controller for lane change execution. This hybrid architecture and the comparison with other classical approaches are the main contributions of this research. Experimental results demonstrate that the proposed framework solves concatenated complex urban scenarios optimally.
更多查看译文
关键词
autonomous driving,complex urban scenarios,deep reinforcement learning,hybrid architecture,hybrid decision making,lane change execution,linear-quadratic regulator controller,maneuver execution module,predictive model controller,proximal policy optimization algorithm,strategy module,tactical decision module,trajectory tracking
AI 理解论文
溯源树
样例
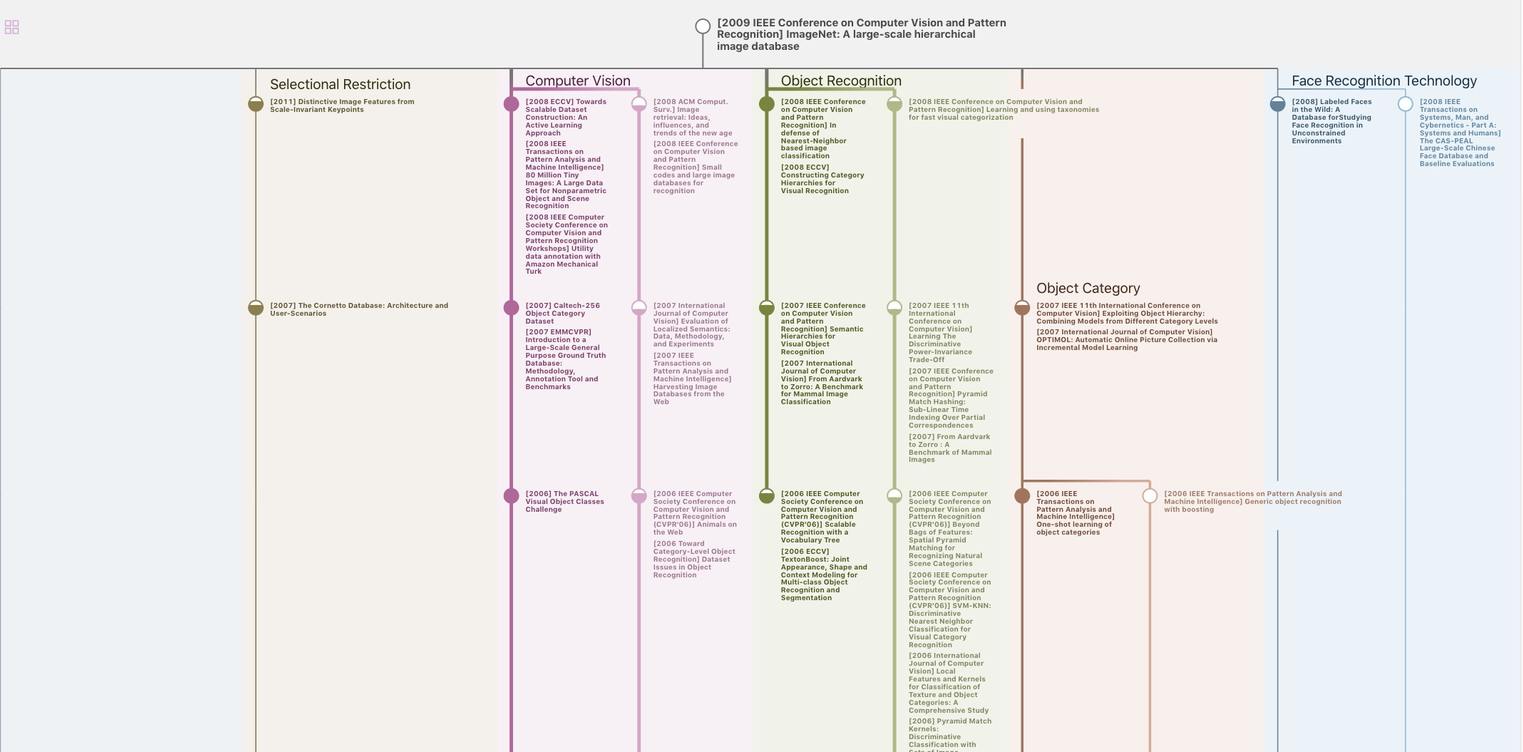
生成溯源树,研究论文发展脉络
Chat Paper
正在生成论文摘要