A Deep Generative Recommendation Method for Unbiased Learning from Implicit Feedback
PROCEEDINGS OF THE 2023 ACM SIGIR INTERNATIONAL CONFERENCE ON THE THEORY OF INFORMATION RETRIEVAL, ICTIR 2023(2023)
摘要
Variational autoencoders (VAEs) are the state-of-the-art model for recommendation with implicit feedback signals. Unfortunately, implicit feedback suffers from selection bias, e.g., popularity bias, position bias, etc., and as a result, training from such signals produces biased recommendation models. Existing methods for debiasing the learning process have not been applied in a generative setting. We address this gap by introducing an inverse propensity scoring (IPS) based method for training VAEs from implicit feedback data in an unbiased way. Our IPS-based estimator for the VAE training objective, VAE-IPS, is provably unbiased w.r.t. selection bias. Our experimental results show that the proposed VAE-IPS model reaches significantly higher performance than existing baselines. Our contributions enable practitioners to combine state-of-the-art VAE recommendation techniques with the advantages of bias mitigation for implicit feedback.
更多查看译文
关键词
Variational autoencoder,Implicit feedback,Selection bias
AI 理解论文
溯源树
样例
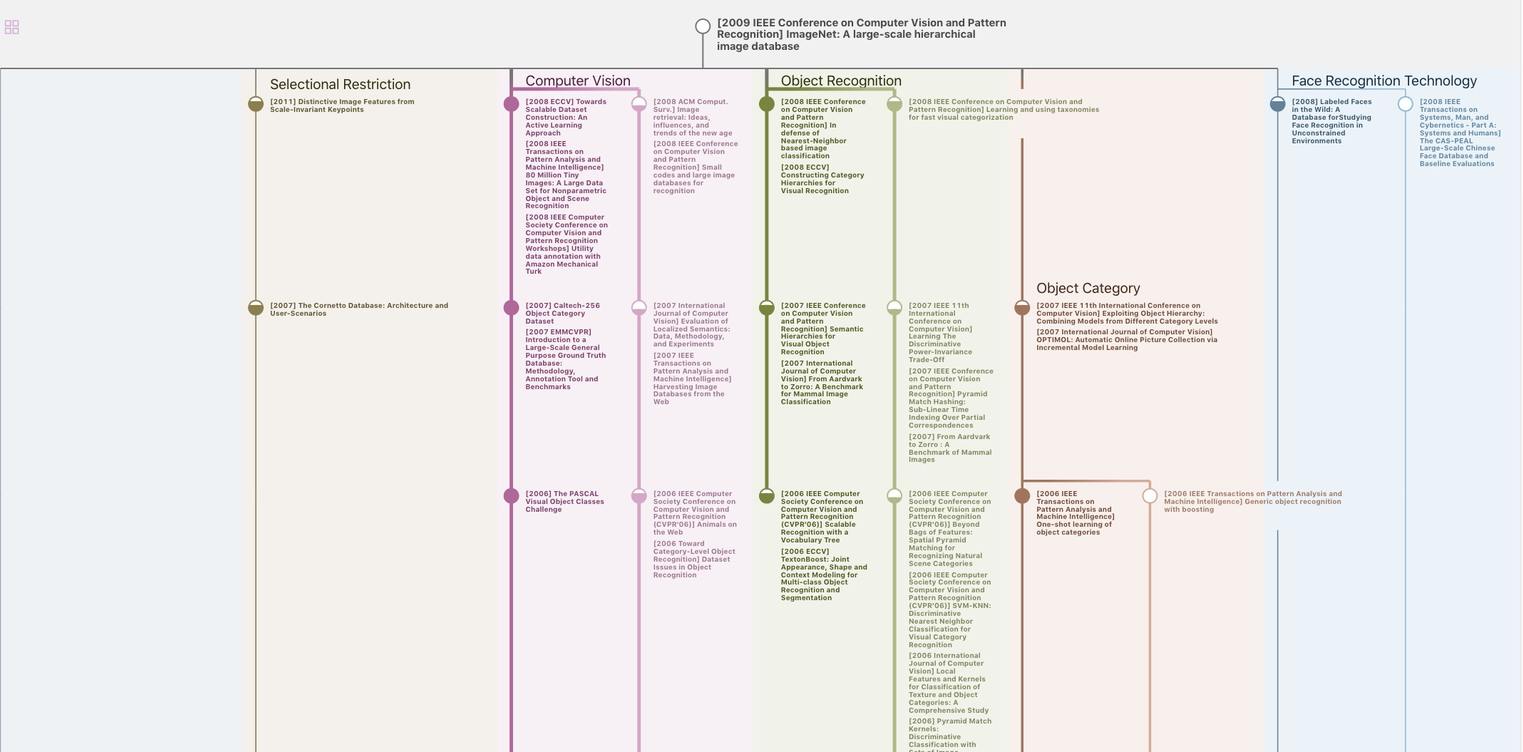
生成溯源树,研究论文发展脉络
Chat Paper
正在生成论文摘要