How to DP-fy ML: A Practical Tutorial to Machine Learning with Differential Privacy
PROCEEDINGS OF THE 29TH ACM SIGKDD CONFERENCE ON KNOWLEDGE DISCOVERY AND DATA MINING, KDD 2023(2023)
摘要
Machine Learning (ML) models are ubiquitous in real world applications and are a constant focus of research. At the same time, the community has started to realize the importance of protecting the privacy of models' training data. Differential Privacy (DP) has become a gold standard for making formal statements about data anonymization. However, while some adoption of DP has happened in industry, attempts to apply DP to real world ML models are still few and far between. The adoption of DP is hindered by limited practical guidance of what DP protection entails, what privacy guarantees to aim for, and the difficulty of achieving good privacy-utility-computation trade-offs for ML models. Tricks for tuning and maximizing performance are scattered among papers or stored in the heads of practitioners. Furthermore, the literature seems to present conflicting evidence on how and whether to apply architectural adjustments and which components are "safe" to use with DP. The question of hyperparameter-tuning for DP models is also often overlooked. Even in academic work, best practices for rigorous reporting of privacy guarantees, like the privacy cost of any data touches and amplification by sampling peculiarities, are often glanced over. In this tutorial, we guide the attendees through in-depth overview of the field of DP ML models. We present information about achieving the best possible DP ML model with rigorous privacy guarantees. People interested in applying DP to their ML models will benefit from a clear overview of current advances and areas for improvement. We also highlight important topics such as privacy accounting and its assumptions, as well as convergence. Additionally we provide an overview of how architectural decisions affect privacy and utility of the ML models. Finally, we have a write-up survey paper that covers all these topics and can serve as further reading material for interested parties.
更多查看译文
关键词
data anonymization,differential privacy,DP-ML,DP-Training
AI 理解论文
溯源树
样例
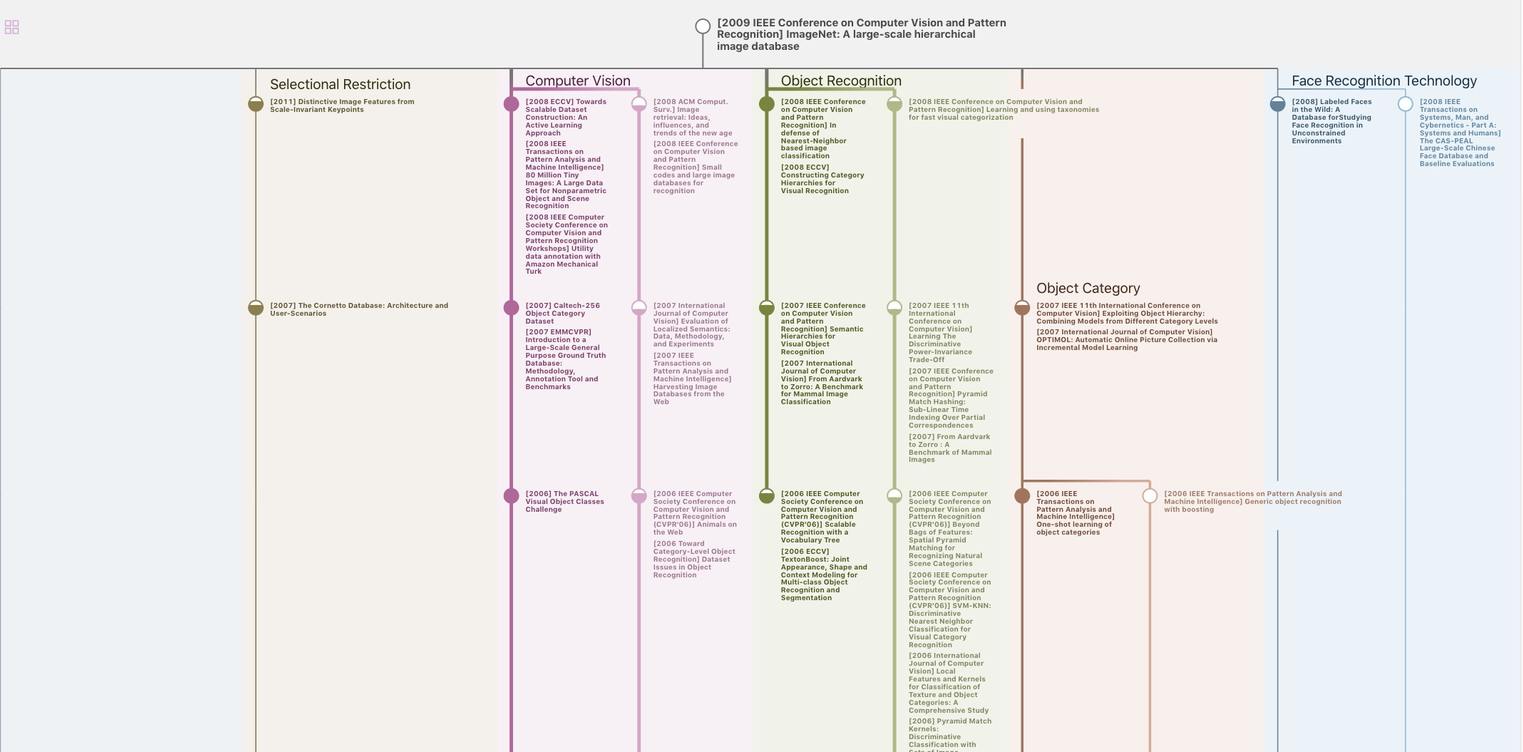
生成溯源树,研究论文发展脉络
Chat Paper
正在生成论文摘要