Fake it till you Detect it: Continual Anomaly Detection in Multivariate Time-Series using Generative AI.
EuroS&P Workshops(2023)
摘要
Anomaly detection in Multivariate Time-Series (MTS) data plays an important role in multiple domains, especially in cybersecurity, for the detection of unknown attacks. DC-VAE is a recent approach we have proposed for anomaly detection in network measurement multivariate data, which uses Variational Auto Encoders (VAEs) and Dilated Convolutional Neural Networks (DCNNs) to model complex and high-dimensional MTS data. However, detecting anomalies using VAEs can result in performance degradation and even catastrophic forgetting when trained on dynamic and evolving network measurements, particularly in the event of concept drifts. We extend DC-VAE to a continual learning setup, leveraging the generative AI properties of the underlying models to deal with continually evolving data. We introduce GenDeX, an approach to Generative AI-based anomaly detection which compresses the patterns extracted from past measurements into a generative model that can synthesize MTS data out of input Gaussian noise, mimicking the characteristics of the MTS data used for training. GenDeX relies on a Deep Generative Replay paradigm to realize continual learning, combining synthesized past MTS measurements with new observations to update the detection model. Using a large-scale, multi-dimensional network monitoring dataset collected from an operational mobile Internet Service Provider (ISP), we showcase the functionality of DCVAE in the event of concept drifts, and study in-depth its generative characteristics, assessing GenDeX synthetically generated MTS examples. GenDeX enables DC-VAE adapting to continually evolving data, overcoming the limitations of catastrophic forgetting.
更多查看译文
关键词
Anomaly Detection,Generative AI,VAE,Multivariate Time-Series,GenDeX
AI 理解论文
溯源树
样例
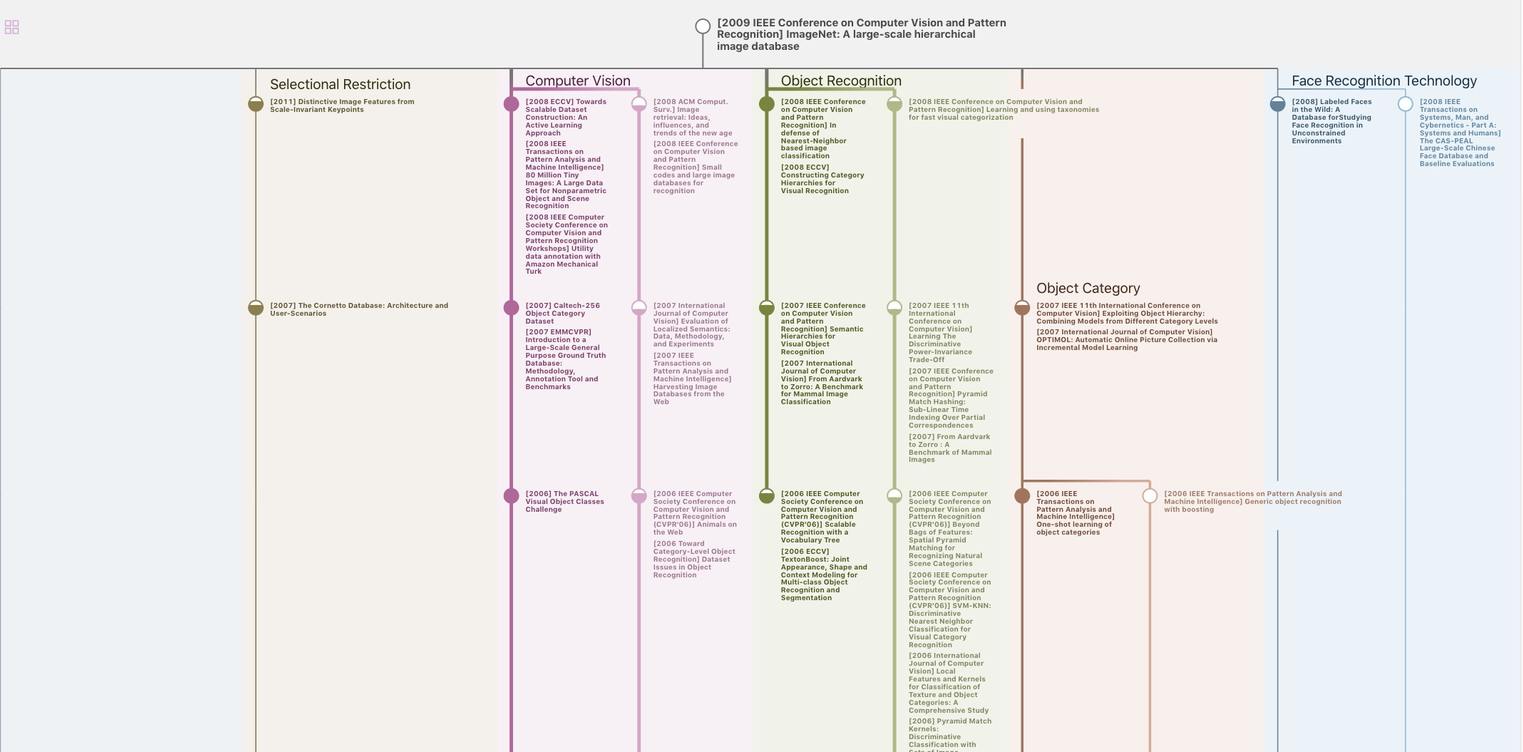
生成溯源树,研究论文发展脉络
Chat Paper
正在生成论文摘要