Auto-HPCnet: An Automatic Framework to Build Neural Network-based Surrogate for High-Performance Computing Applications
PROCEEDINGS OF THE 32ND INTERNATIONAL SYMPOSIUM ON HIGH-PERFORMANCE PARALLEL AND DISTRIBUTED COMPUTING, HPDC 2023(2023)
摘要
High-performance computing communities are increasingly adopting Neural Networks (NN) as surrogate models in their applications to generate scientific insights. Replacing an execution phase in the application with NN models can bring significant performance improvement. However, there is a lack of tools that can help domain scientists automatically apply NN-based surrogate models to HPC applications. We introduce a framework, named Auto-HPCnet, to democratize the usage of NN-based surrogates. Auto-HPCnet is the first end-to-end framework that makes past proposals for the NN-based surrogate model practical and disciplined. Auto-HPCnet introduces a workflow to address unique challenges when applying the approximation, such as feature acquisition and meeting the application-specific constraint on the quality of final computation outcome. We show that Auto-HPCnet can leverage NN for a set of HPC applications and achieve 5.50x speedup on average (up to 16.8x speedup and with data preparation cost included) while meeting the application-specific constraint on the final computation quality.
更多查看译文
关键词
Scientific Machine Learning,Neural Architecture Search,Surrogate Model Construction,Bayesian Optimization
AI 理解论文
溯源树
样例
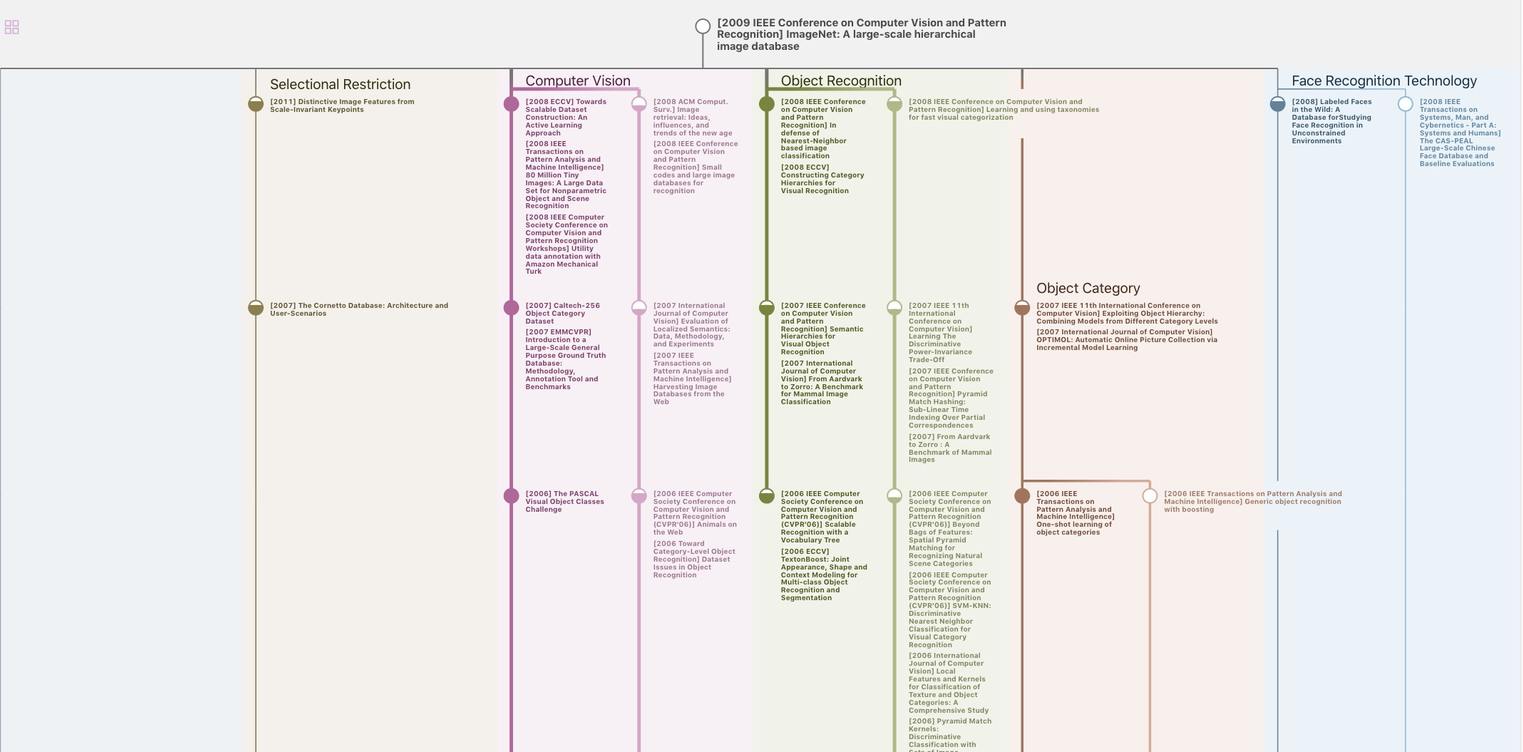
生成溯源树,研究论文发展脉络
Chat Paper
正在生成论文摘要