Discrepancy Scaling for Fast Unsupervised Anomaly Localization.
COMPSAC(2023)
摘要
Computer vision systems can automatically find and segment anomalies in images even without ever seeing anomalous observations during training. Many methods for such unsupervised anomaly detection (AD) and localization (AL) tasks have been introduced in recent years, but the most accurate methods tend to be computationally heavy. In this paper, we propose Discrepancy Scaling, a method that significantly improves the accuracy of a very fast AD and AL approach called Student-Teacher Feature Pyramid Matching. We show that with Discrepancy Scaling, even a small, mobile-friendly convolutional neural network can perform well on AD and AL tasks.
更多查看译文
关键词
Anomaly detection, anomaly localization, unsupervised learning, deep learning
AI 理解论文
溯源树
样例
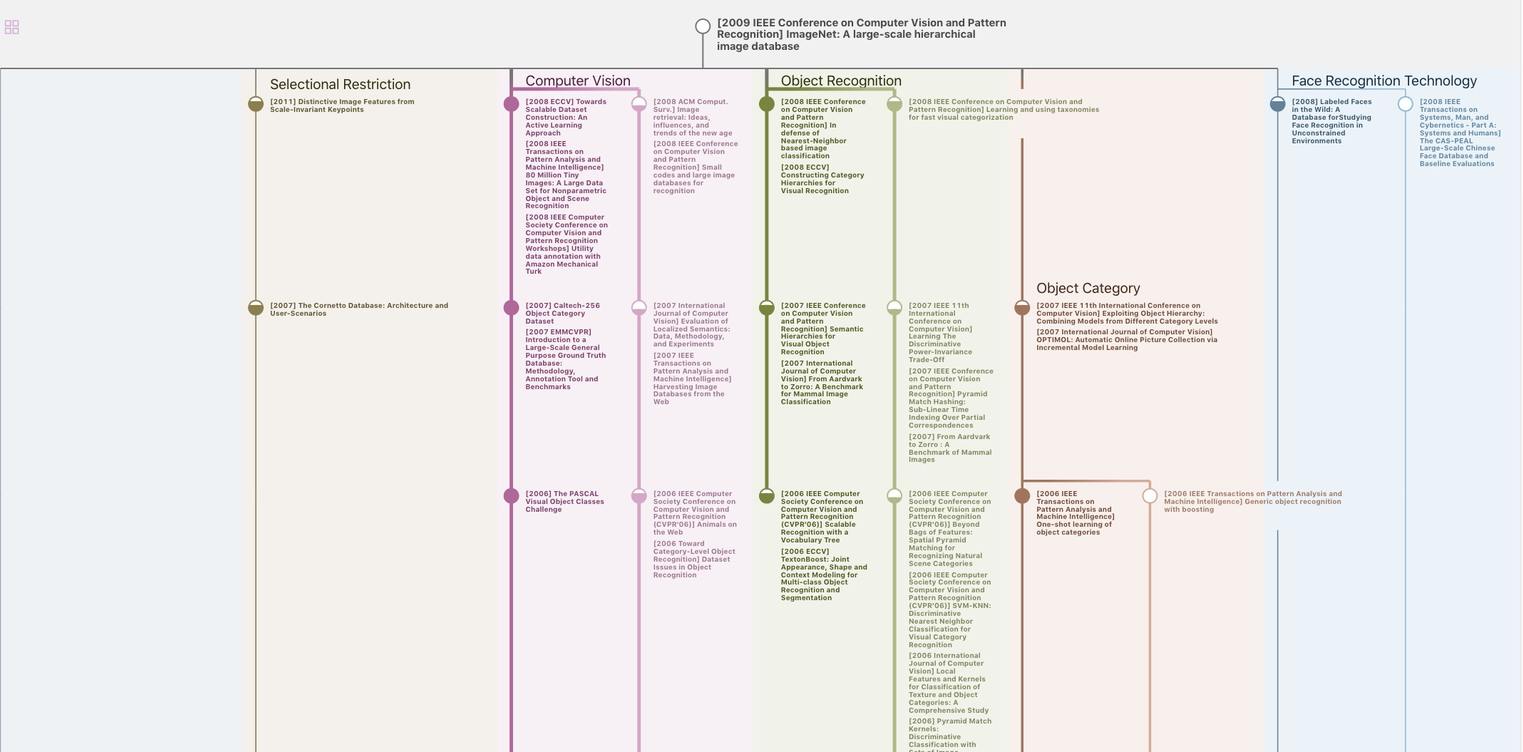
生成溯源树,研究论文发展脉络
Chat Paper
正在生成论文摘要