Failure Prediction in 2D Document Information Extraction with Calibrated Confidence Scores.
COMPSAC(2023)
摘要
Modern machine learning models can achieve impressive results in many tasks, but often fail to express reliably how confident they are with their predictions. In an industrial setting, the end goal is usually not a prediction of a model, but a decision based on that prediction. It is often not sufficient to generate high-accuracy predictions on average. One also needs to estimate the uncertainty and risks involved when making related decisions. Thus, having reliable and calibrated uncertainty estimates is highly useful for any model used in automated decision-making. In this paper, we present a case study, where we propose a novel method to improve the uncertainty estimates of an in-production machine learning model operating in an industrial setting with real-life data. This model is used by Basware, a Finnish software company, to extract information from invoices in the form of machine-readable PDFs. The solution we propose is shown to produce calibrated confidence estimates, which outperform legacy estimates on several relevant metrics, increasing coverage of automated invoices from 65.6% to 73.2% with no increase in error rate.
更多查看译文
关键词
machine learning, uncertainty estimation, confidence calibration, failure prediction, information extraction
AI 理解论文
溯源树
样例
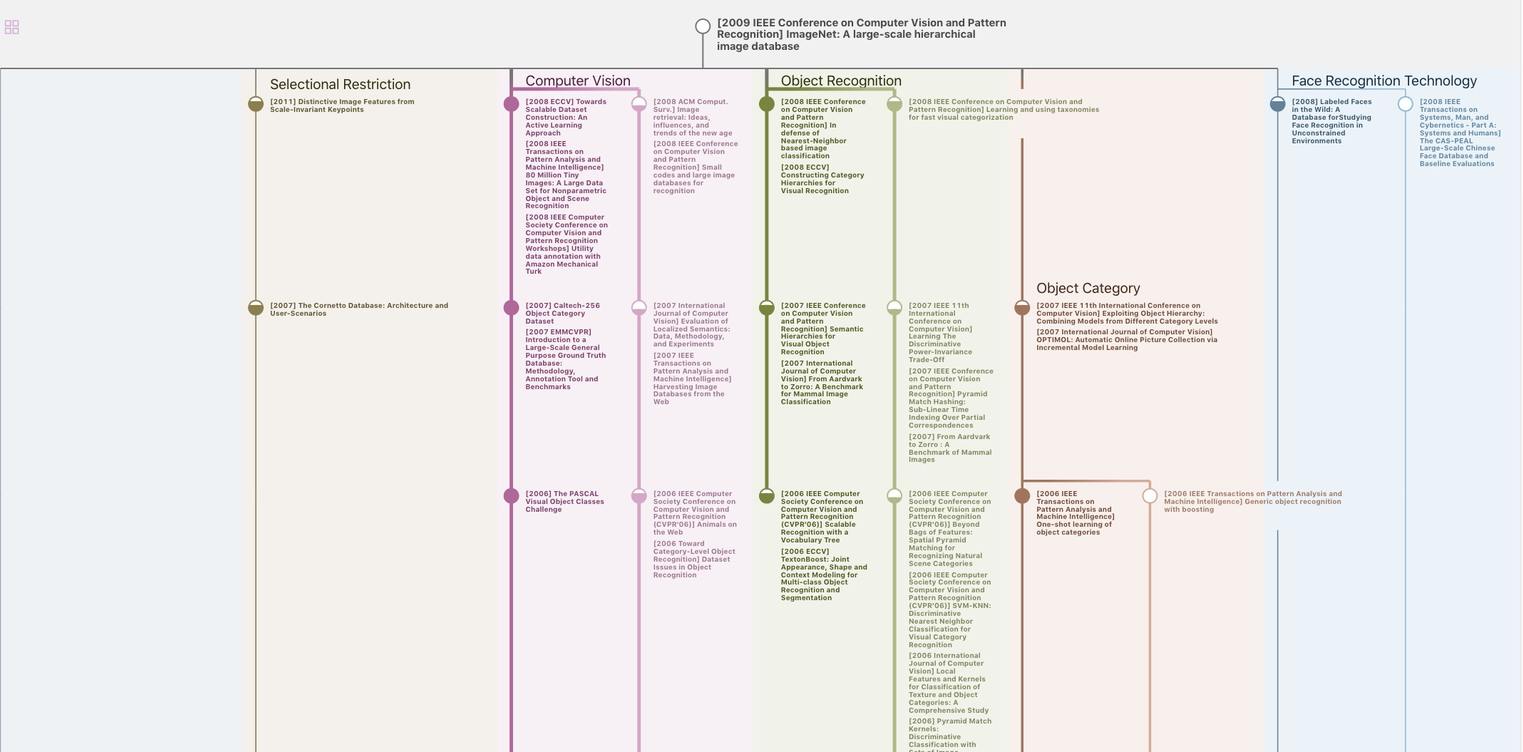
生成溯源树,研究论文发展脉络
Chat Paper
正在生成论文摘要