Dynamic Topic Modeling with Tensor Decomposition as a Tool to Explore the Legal Precedent Relevance Over Time
DocEng(2023)
摘要
The precedent is a textual citation of prior court decisions. This undoubtedly offers great value in a common-law-based judicial system where courts are bound to their previous rulings, such as in the United States, Canada, and India. In those countries, precedent relevance detection is an issue that has attracted considerable attention where studies propose Network Science techniques for relevance measurement - where decisions and their relationships are represented in network structures. However, those methods fail to capture the precedent relevance in the Brazilian scenario due to the massive and increasing number of decisions issued yearly. The Brazilian Supreme Court (STF), the highest judicial body in Brazil, has produced more than a million decisions over the last decade. Therefore, we propose an interpretable and cost-effective process to explore the precedent through latent topics that emerge, evolve, and fade over time in a collection of historical documents. To do so, we explore dynamic topic modeling with tensor decomposition as a tool to investigate the legal changes embedded in those decisions over time. We base our study on the individual decisions published by STF between 2000 and 2018. Additionally, through experiments, we explore the proposed process within different scenarios to investigate the precedent citations over the STF's recent history, and how those citations correlates with the legal named entities, such as legislative references. The experiments showed the process' capability to produce coherent and interpretable results for temporal analysis of the precedent citations in larger collections of documents. Also, it presents the potential to support further studies in the legal domain.
更多查看译文
关键词
Legal Rulings,Legal Documents,Precedents,Network Analysis,Legal Arguments,Brazilian Law
AI 理解论文
溯源树
样例
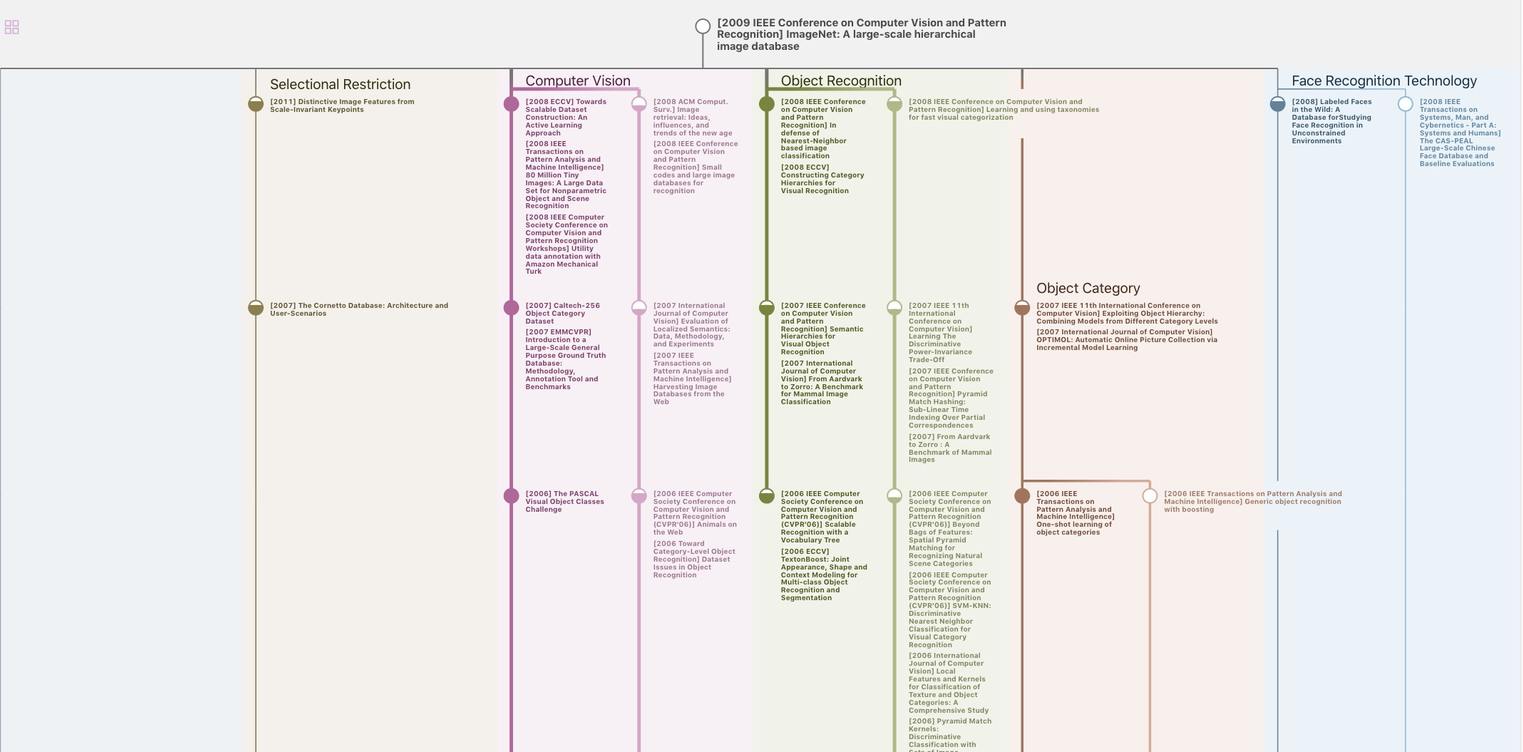
生成溯源树,研究论文发展脉络
Chat Paper
正在生成论文摘要