Image Quality Assessment Metric Fusing Traditional and Dempster-Shafer Theory.
ICPR Workshops (2)(2022)
摘要
Image analysis is being applied in many applications including industrial automation with the Industrial Internet of Things and machine vision. The images captured by cameras, from the outdoor environment are impacted by various parameters such as lens blur, dirty lens and lens distortion (barrel distortion). There exist many approaches that assess the impact of camera parameters on the quality of the images. However, most of these techniques do not use important quality assessment metrics such as Oriented FAST and Rotated BRIEF and Structural Content. None of these techniques objectively evaluate the impact of barrel distortion on the image quality using quality assessment metrics such as Mean Square Error, Peak signal-to-noise ratio, Structural Content, Oriented FAST and Rotated BRIEF and Structural Similarity Index. In this paper, besides lens dirtiness and blurring, we also examine the impact of barrel distortion using various types of dataset having different levels of barrel distortion. Analysis shows none of the existing metrics produces quality values consistent with intuitively defined impact levels for lens blur, dirtiness and barrel distortion. To address the loopholes of existing metrics and make the quality assessment metric more reliable, we present two new image quality assessment metrics. For our combined metric, results show that the maximum values of impact level created by barrel distortion, blurriness and dirtiness are 66.6%, 87.9% and 94.4%, respectively. These results demonstrate the effectiveness of our metric to assess the impact level more accurately. The second approach fuses the quality values obtained from different metrics using a decision fusion technique known as the Dempster-Shafer (DS) theory. Our metric produces quality values that are more consistent and conform with the perceptually defined camera parameter impact levels. For all above-mentioned camera impacts, our metric DS exhibits $$100\%$$ assessment reliability, which includes an enormous improvement over other metrics.
更多查看译文
关键词
quality,dempster-shafer
AI 理解论文
溯源树
样例
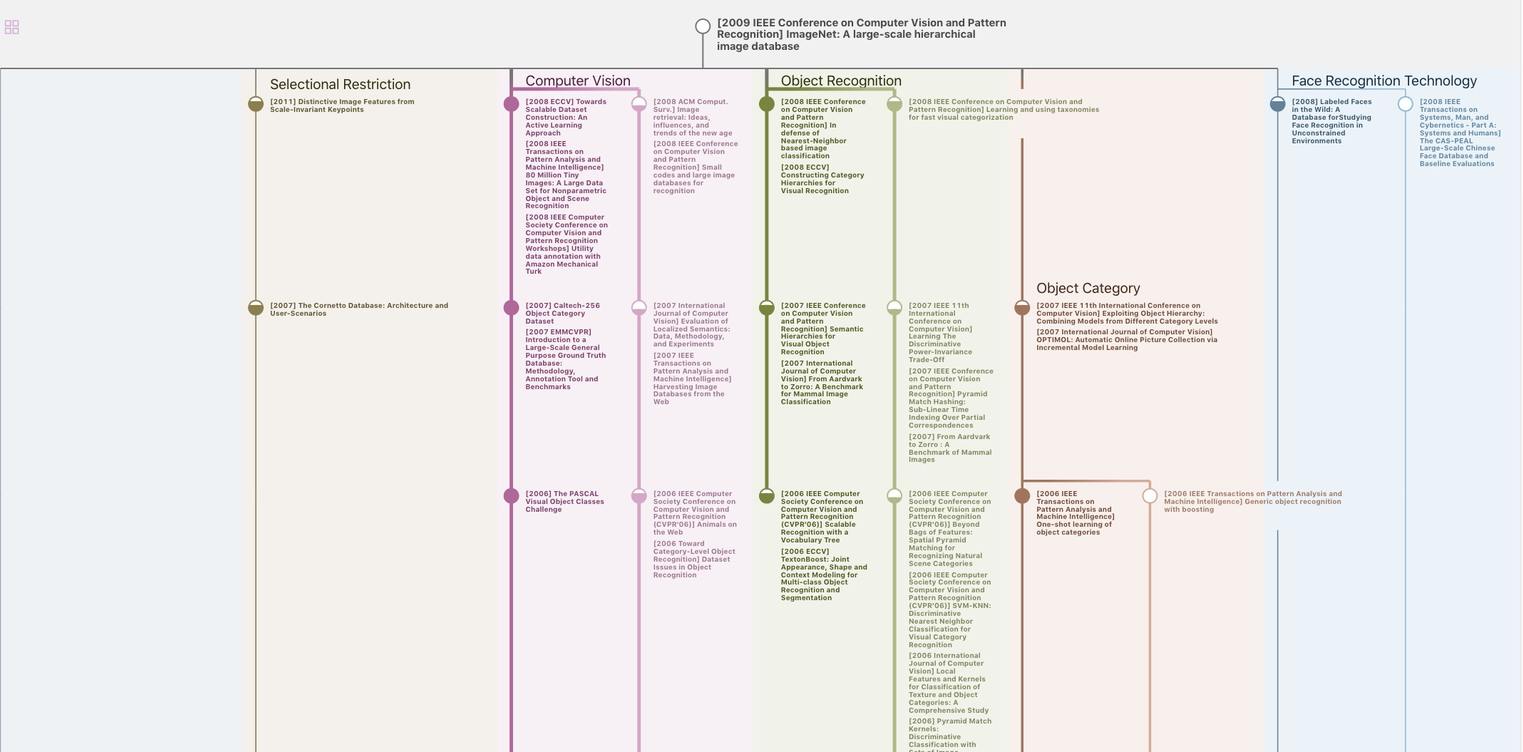
生成溯源树,研究论文发展脉络
Chat Paper
正在生成论文摘要